Special Issue: Applications of Machine Learning in Financial Economics
Guest Editors
Prof. Zhenghui Li
Guangzhou Institute of International Finance, Guangzhou University, Guangzhou, China
Email: lizh@gzhu.edu.cn
Dr. Feite Zhou
Department of Applied Mathematics, The Hong Kong Polytechnic University, 11 Yuk Choi Road, Hung Hom, Hong Kong
Email: jupiterzhou@foxmail.com
Manuscript Topics
Nowadays, the appearance of machine learning in economics steadily increased. Initially, it was applied in forecasting financial time series where long datasets are widely available. Machine learning systems from that era required extensive data sets that did not exist in other areas of economics. Furthermore, training was very time-consuming due to the low processing power of the computers of the period. Today, the use of many new machine learning architectures that do not require unreasonably long data sets, is an interesting and very promising avenue in economic forecasting. This is the case not only for financial problems but also macroeconomics or microeconomics applications where data sets are inherently limited in size. Recent machine learning applications in financial fields seem very successful as compared to traditional empirical models. Currently, we encounter new methodologies merging and combining econometrics with machine learning. Moreover, it is interesting how machine techniques and empirical procedures are now popular and are steadily adopted to traditional econometrics methodologies.
The special issue aims to publish review papers, research articles, and communications on the application of machine learning to economics and finance that present new original methods, applications, data analyses, case studies, comparative studies, and other results.
Potential topics include but are not limited to the following:
• Machine learning algorithms
• Computational statistics
• Dimensionality reduction and variable selection
• Nonparametric statistical modeling
• Supervised learning (classification, regression)
• Clustering methods
• Financial statistics and econometrics
• Statistical algorithms
• Time series analysis and forecasting
• Decision trees
• Ensemble methods
• Neural networks
• Deep learning
• Hybrid models
Instruction for Authors
https://www.aimspress.com/dsfe/news/solo-detail/instructionsforauthors
Please submit your manuscript to online submission system
https://aimspress.jams.pub/
Paper Submission
All manuscripts will be peer-reviewed before their acceptance for publication. The deadline for manuscript submission is 31 December 2023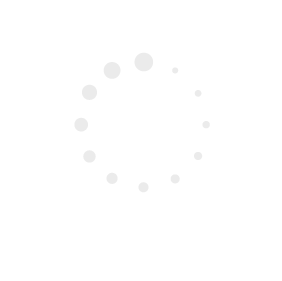