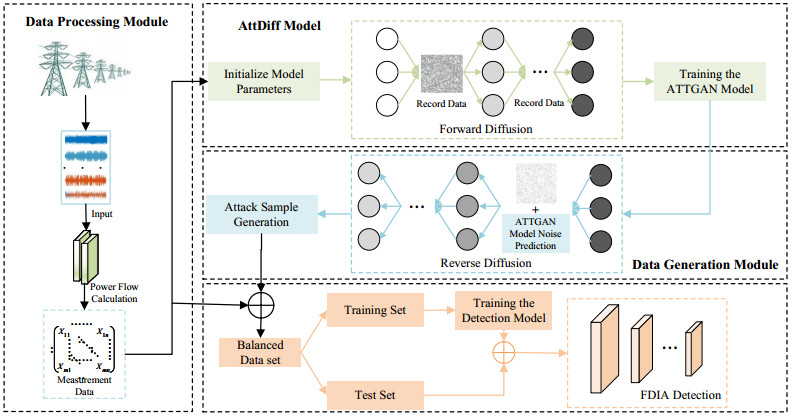
This study explores the service innovation model of Taiwan's Yingge Historical Street of Ceramics and analyzes how political capital and public-private partnerships (PPPs) influence service innovation development in the district. By adopting a case-study approach, data was collected from literature and secondary sources. Findings reveal three aspects of the service innovation model: (1) innovation based on cultural heritage, utilizing ceramic culture and technology to offer diverse cultural experiences; (2) community participation as the core, enhancing cohesion, identity, and promoting cultural heritage development; and (3) service innovation mechanism through PPPs, integrating public and private resources/capabilities to enhance efficiency and quality. The study highlights the significant impact of political capital (government support, funding, regulatory frameworks, and local groups' political influence) and PPPs (collective public-private actions including resource integration, cooperation norms, trust-building, and value co-creation) on service innovation. This contributes theoretically and practically to understanding service innovation mechanisms in cultural districts and promoting their development.
Citation: YiFu Hsu, ChunLiang Chen. Service innovation models in cultural districts: A case of Taiwan Yingge Historical Street[J]. Urban Resilience and Sustainability, 2024, 2(4): 371-389. doi: 10.3934/urs.2024020
[1] | Hashem Al-Mattarneh, Rabah Ismail, Adnan Rawashdeh, Hamsa Nimer, Mohanad Khodier, Randa Hatamleh, Dua'a Telfah, Yaser Jaradat . Development of new dielectric models for soil moisture content using mixture theory, empirical methods, and artificial neural network. AIMS Environmental Science, 2025, 12(1): 137-164. doi: 10.3934/environsci.2025007 |
[2] | Qianlan Li, Qingdan Wu, Xiaochen Zheng, Pengfei Wang, Dongsheng Zou, Fen Liu, Zhihua Xiao . The conversion and migration behavior of phosphorus speciation during pyrolysis of different sludges. AIMS Environmental Science, 2024, 11(1): 1-20. doi: 10.3934/environsci.2024001 |
[3] | Li Ma, Likun Zhan, Qingdan Wu, Longcheng Li, Xiaochen Zheng, Zhihua Xiao, Jingchen Zou . Optimization of liquefaction process based on global meta-analysis and machine learning approach: Effect of process conditions and raw material selection on remaining ratio and bioavailability of heavy metals in biochar. AIMS Environmental Science, 2024, 11(3): 342-359. doi: 10.3934/environsci.2024016 |
[4] | Diksha Puri, Parveen Sihag, Mohindra Singh Thakur, Mohammed Jameel, Aaron Anil Chadee, Mohammad Azamathulla Hazi . Analysis of data splitting on streamflow prediction using random forest. AIMS Environmental Science, 2024, 11(4): 593-609. doi: 10.3934/environsci.2024029 |
[5] | Adi Setiawan, Ananda Fringki, M. Iqbal Hifzi, Shafira Riskina, Jalaluddin, Eddy Kurniawan, Burhanuddin . The effect of feedstock densification on the process and product properties of sugarcane leaves pyrolysis. AIMS Environmental Science, 2024, 11(6): 866-882. doi: 10.3934/environsci.2024043 |
[6] | Carlos Garcia, Teresa Hernandez, Maria D Coll, Sara Ondoño . Organic amendments for soil restoration in arid and semiarid areas: a review. AIMS Environmental Science, 2017, 4(5): 640-676. doi: 10.3934/environsci.2017.5.640 |
[7] | Maja Radziemska, Agnieszka Bęś, Zygmunt M. Gusiatin, Jerzy Jeznach, Zbigniew Mazur, Martin Brtnický . Novel combined amendments for sustainable remediation of the Pb-contaminated soil. AIMS Environmental Science, 2020, 7(1): 1-12. doi: 10.3934/environsci.2020001 |
[8] | Benjamin Hersh, Amin Mirkouei, John Sessions, Behnaz Rezaie, Yaqi You . A review and future directions on enhancing sustainability benefits across food-energy-water systems: the potential role of biochar-derived products. AIMS Environmental Science, 2019, 6(5): 379-416. doi: 10.3934/environsci.2019.5.379 |
[9] | Voke O. Urhibo, Bernard O. Ejechi . Crude oil degradation potential of bacteria isolated from oil-polluted soil and animal wastes in soil amended with animal wastes. AIMS Environmental Science, 2017, 4(2): 277-286. doi: 10.3934/environsci.2017.2.277 |
[10] | Jerry R. Miller, John P. Gannon, Kyle Corcoran . Concentrations, mobility, and potential ecological risks of selected metals within compost amended, reclaimed coal mine soils, tropical South Sumatra, Indonesia. AIMS Environmental Science, 2019, 6(4): 298-325. doi: 10.3934/environsci.2019.4.298 |
This study explores the service innovation model of Taiwan's Yingge Historical Street of Ceramics and analyzes how political capital and public-private partnerships (PPPs) influence service innovation development in the district. By adopting a case-study approach, data was collected from literature and secondary sources. Findings reveal three aspects of the service innovation model: (1) innovation based on cultural heritage, utilizing ceramic culture and technology to offer diverse cultural experiences; (2) community participation as the core, enhancing cohesion, identity, and promoting cultural heritage development; and (3) service innovation mechanism through PPPs, integrating public and private resources/capabilities to enhance efficiency and quality. The study highlights the significant impact of political capital (government support, funding, regulatory frameworks, and local groups' political influence) and PPPs (collective public-private actions including resource integration, cooperation norms, trust-building, and value co-creation) on service innovation. This contributes theoretically and practically to understanding service innovation mechanisms in cultural districts and promoting their development.
As a key component of modern power systems, smart grids are designed to achieve efficient, flexible, and safe energy distribution. By integrating advanced sensor technology, communication networks, and intelligent control strategies, smart grids have gradually evolved into a highly integrated cyber-physical system (CPS) [1], and can respond to various operating conditions and external events in real time, optimize resource allocation, and improve the system's adaptability and anti-interference capabilities. However, this progress has also brought new security challenges, especially in the face of increasingly complex cyber attacks. A false data injection attack (FDIA) is one of the main security threats facing smart grids. By manipulating the measurement data of the power grid, attackers can mislead the system state estimation and decision-making process, thereby posing a serious threat to the stability and security of the power grid [2]. The stealth and complexity of such attacks make it difficult for traditional security measures to effectively deal with it. It is urgent to develop new defense mechanisms to ensure the data integrity and system security of smart grids.
In recent years, large-scale grid security incidents in which criminals use data tampering mechanisms to attack occur frequently. Take the attack on Ukrenergo as an example. From 2015 to 2016, Ukrenergo suffered two cyber attacks. The attackers injected false data into the monitoring data acquisition system and deleted the original data, causing huge economic losses and social unrest [3]. This has also attracted the attention of many researchers to FDIA attacks. Since false data can be cleverly designed by attackers to evade detection by detection mechanisms, the purpose of intrusion can be achieved, causing irreversible damage to the power system. Therefore, society has carried out a lot of research work to identify and defend against an FDIA. For example, Kumar et al. [4] explored the effectiveness of machine learning algorithms in detecting false data injection attacks (FDIA) in critical infrastructure. Cheng et al. [5] introduced a highly discriminative FDIA detector called k-minimum residual similarity (kSRS) detection. This method achieves a high detection rate and low false alarm rate for an FDIA by characterizing the statistical consistency of measurement residuals in AC state estimation. Qu et al. [6] proposed a novel approach to improve the accuracy of DDIA detection and localization by synthesizing topological correlations in non-Euclidean spatial attributes of grid data. However, the above research is based on traditional machine learning to design FDIA detection solutions, which are limited by the complexity of manual feature extraction, dimensionality disaster, and insufficient generalization ability when detecting FDIA attacks. Therefore, traditional FDIA detection methods have gradually lost their effectiveness in defending against these network attacks.
With the update and iteration of technology, some FDIA detection methods based on machine learning and deep learning have also been developed. In order to correctly detect false data injection attacks in power grids, Fand et al. [7] proposed a one-dimensional convolutional neural network (1DCNN) and long short-term memory (LSTM) network multi-channel fusion network model based on the residual neural network (ResNet) structure, referred to as the channel-fused Res-CNN-LSTM network model. In order to make the detection results more credible, Su et al. [8] proposed an interpretable deep learning FDIA detection method, namely the dual attention multi-head graph attention network (DAMGAT). Takiddin et al. [9] proposed an anomaly detector based on generalized graph neural networks (GNN), which is robust to an FDIA and data poisoning. Li et al. [10] proposed a false data detection method based on graph neural networks, extracting the spatial features of power grid topology information and operation data through gated graph neural networks (GGNN). Qu et al. [11] proposed an improved adaptive Kalman filter (AKF) and convolutional neural network (CNN) hybrid detection method for power information physical system (CPS) FDIA. Wang et al. [12] proposed a deep learning-based location detection architecture (DLLD) to detect the exact location of an FDIA in real time. Xie et al. [13] proposed a Bayesian deep learning-based method to detect network attacks and maintain the security of smart grids. Although these methods show great advantages in FDIA attack detection, they also have great limitations. Most of the methods rely on large-scale training attack samples, but in the actual scenario, FDIA attack samples are difficult to obtain, and samples are scarce, resulting in extremely unbalanced sample data sets. Although they have strong detection performance, if there are not enough attack samples for model training, the efficiency of the FDIA attack detection model in smart grids will also be reduced. Therefore, the construction of FDIA attack samples and the acquisition of balanced data sets become the first problems to be solved.
Several researchers have conducted extensive research on the construction of FDIA attack vectors. Yan et al. [14] proposed the DoS-WGAN architecture, which uses a Wasserstein generative adversarial network (WGAN) with gradient penalty technology to evade network traffic classifiers. WGAN is used to automatically synthesize attack vectors that can be disguised as normal network traffic in the case of network attacks and bypass the detection of network intrusion detection systems. To address the problem of highly unbalanced data samples, Kumar et al. [15] proposed a Wasserstein conditional generative adversarial network (WCGAN) combined with an XGBoost classifier. The gradient penalty is used together with WCGAN for stable learning of the model. Tian et al. [16] introduced the joint adversarial examples and FDIA (AFDIA) to explore various attack scenarios on power system state estimation. Considering that disturbances directly added to the measurement are likely to be detected by the BDD, a method of adding disturbances to the state variables is proposed to ensure that the attack is invisible to the BDD. Bhattacharjee et al. [17] proposed a scheme for constructing invisible attack vectors based on independent component analysis to identify mixing matrices. Wu et al. [18] proposed a new FDIA sample generation method to construct large-scale attack samples by introducing a mixed Laplace model that can accurately fit the distribution of data changes. Li et al. [19] designed an efficient adversarial model for generating FDIA attack samples at the edge of public and private networks that can effectively bypass detection models and threaten grid systems. Through interactive adversarial learning, efficient FDIA attack samples can be continuously generated. Although the existing sample generation method can appropriately expand the FDIA attack sample library, its generation method is too simple, resulting in low concealment and weak attack capability of the generated samples, which is not conducive to the training of the detection model and the improvement of performance.
With the rise of generative AI technology, a new perspective has been brought to the detection of various network attacks. Among them, the diffusion model has gradually become a new and most advanced model among the deep generation models in recent years, showing superior performance in the research of image generation and multi-modal generation. Compared with traditional deep learning methods, it can generate new attack samples with better concealment and attack capabilities by learning the distribution of data, which provides a new way to understand the strategies that attackers may adopt. Therefore, in response to the above problems, considering the diversity, concealment, and scalability of the data generated by the diffusion model [20] and its powerful generation ability, this paper presents a method to generate FDIA attack samples using an adversarial attention diffusion model. The proposed AttDiff model consists of a diffusion model and a GAN (ATTGAN) model with an attention mechanism. First, as the time step increases, in the forward diffusion process, the original input data is gradually denoised, the attack vector is injected, and the data state corresponding to each time step and the added noise are recorded. The recorded data is then used to train generative adversarial network (ATTGAN) models with attention mechanisms. The purpose is to allow the ATTGAN model to effectively focus on the information of power grid measurements and topological nodes through adversarial training, while weakening irrelevant information and fully learning the characteristics of real sample data. Afterward, in the reverse diffusion process, the noise-added data processed in the forward diffusion process should be denoised from back to front in combination with the size of the time step, and the trained ATTGAN model should be combined to predict the noise value to be removed. In this process, the purpose is to build the attack sample data closest to the real sample from the noise. The FDIA sample generated by the method in this paper is more diversified, more hidden, and can effectively simulate the behavior of the real attacker, and is less likely to be detected by the detector.
Comparing with the existing schemes, we make the following novel contributions in FDIA attack sample generation:
● We propose a novel and efficient sample generation method. Our proposed scheme introduces an adversarial attention-diffusion model to generate a large number of attack samples, which can solve the problem of scarce attack samples in FDIA detection. According to the investigation, this is the first time that the diffusion model has been applied to the study of FDIA attack sample generation.
● We use the forward diffusion of the diffusion model to add noise to the real data while injecting the attack vector. Subsequently, the ATTGAN model is trained to effectively focus on the information of power grid measurements and topological nodes, while weakening irrelevant information. In the reverse diffusion process, we combine the trained ATTGAN model to predict the noise, and gradually iterate forward step by step and denoise in this process. A large number of efficient FDIA attack samples can be generated.
● Comprehensive experiments are performed with classical data sets. The experimental results demonstrate that the proposed method can significantly improve the overall performance against FDIA detection, and outperform the existing FDIA attack sample construction methods in terms of attack strength, concealment, and decline of FDIA detection accuracy.
The rest of this paper is organized as follows. In Section 2, related works on FDIAs and diffusion models are introduced. In Section 3, the framework of the proposed attack sample generation method based on the adversarial attention diffusion model and the specific structure of the AttDiff model are introduced in detail. In Section 4, a large number of experiments and corresponding comparative experiments are carried out, and the experimental results are presented. Finally, in Section 5, the work of this paper is summarized and prospected.
A fake data injection attack is a kind of network attack against CPS. Its core is to construct and inject fake data to bypass system detection, so as to achieve the purpose of attack. This type of attack is particularly common in critical infrastructure such as smart grids and can have a serious impact on the operational stability, supply reliability, and data accuracy of the power system.
The core principle of constructing an FDIA is to manipulate the key measurement data in the AC power system in order to change the state estimation results of the system. By tampering with the active power on any bus node, an attacker generates a set of attack vectors designed to interfere with the normal operation of the system. These attack vectors are injected into the measured data of the system, which causes the calculated state estimation to deviate from the real operating state of the power grid. In this way, attackers are able to mislead the system's decision-making process for their potential sabotage or manipulation purposes. In DC power flow, the relationship between system state x and measurement data z can be expressed as:
z=Hx+e | (1) |
where z={z1,z2,…,zI} represents the measurement data, x={x1,x2,…,xJ} represents the state vector, e={e1,e2,…,eI} represents the measurement error vector, I and J represent the number of measurements and the number of state data, respectively, and H represents the measurement Jacobian matrix, which is a matrix representing the grid topology information. The measurement data includes the flow and injection power of different buses.
For DC state estimation, assuming that the per unit voltage of each node in the system is 1, ignoring the influence of line resistance and ground branch, the power between bus i and bus j is:
Pij=(θi−θj)xij | (2) |
where xij represents the reactance of the transmission line between bus i and bus j. θi and θj are the voltage phase angles of bus i and bus j. The minimized objective function is estimated by linear weighted least squares:
minF(x)=(z−Hx)TR−1(z−Hx) | (3) |
where R is the error covariance matrix of the measurement, and ∧x of the minimized objective function is:
∧x=(HTR−1H)−1HTR−1z | (4) |
The principle of residual detection has been widely used in the traditional bad data detection mechanism (BDD). Residual r is the difference between the measurement value z and the measurement estimate ∧z=H∧x, i.e.:
r=z−∧z | (5) |
BDD can then detect bad data by comparing the Euclidean norm of the measured residuals to a predefined threshold τ, as follows:
||r||≤τ | (6) |
In order to make the injected attack vector invisible, we assume that the measurement error e follows an ideal normal distribution, and use a=[a1,a2,...,am]T to represent the FDIA vector injected by the attacker into the measured value. Then the actual measurement data is za=z+a, and the error vector of the state variable caused by the FDIA is c=[c1,c2,...,cn]T. At this time, the estimated state variable xa=∧x+c, and the residual error after attack can be expressed as:
||ra||=||za−Hxa||=||z+a−H(∧x+c)||=||z−H∧x+a−Hc|| | (7) |
When a=Hc, the following formula holds:
||ra||=||za−Hxa||=||z−H∧x||=||r||≤τ | (8) |
It can be seen that when the above conditions are met, an FDIA can successfully bypass BDD, causing changes and losses to the state estimation of the power system. However, it is worth noting that the basic assumption of an FDIA is that both the attacker and the defender (that is, the power system operator) have complete information about the parameters and topology of the power system, that is, H in Eq (1), and it is also necessary to find highly sparse attack vectors that meet certain conditions to launch attacks. For this, the cost is high.
Diffusion models have surpassed the previous leading generative adversarial network (GAN) technology in the field of image creation and have shown great application potential in multiple fields such as computer vision, natural language processing, signal processing, multimodal learning, molecular structure modeling, time series analysis, and adversarial sample purification. In the field of computer vision, diffusion models are used to perform various image restoration tasks, such as image resolution enhancement, image painting, and image translation [21–23]. In natural language processing, diffusion models can generate character level text through a technique called the discrete denoising diffusion probability model (D3PM) [24]. In addition, in terms of time series data generation, diffusion models are also used to fill in the blanks in time series data, as shown in the literature [25,26]. In the field of data generation, due to the similar structure of time series data and intrusion detection data, both of them are one-dimensional data composed of multiple features, which stimulates the idea of generating FDIA attack sample data in this experiment. According to the survey, there is no literature that uses diffusion models for FDIA attack sample generation to generate a balanced data set.
The existing sample generation methods are too simple, resulting in low concealment and weak attack ability of the generated samples, which is not conducive to the training and performance improvement of the detection model. The use of a diffusion model can construct more robust, more threatening, and more hidden attack samples, so that the training of the detection model can be further optimized and improved, and finally achieve the purpose of enhancing the defense capability of the power system, making its operation safer and more stable.
The proposed framework mainly consists of three parts: the data processing module, AttDiff model, and data generation module. In the data processing module, various interactive data from the virtual power grid and power plant are first collected and processed, and then the flow calculation is performed on these data. Then, the measurement data in the power system is further simulated through simulation. Then, the processed measurement data is input into the AttDiff model and the sample data is normalized to adapt to network training. After initializing the model parameters, the forward diffusion process of the diffusion model is entered. During this process, as the time step increases, noise and attack vectors are gradually added to the original data, and the data in the forward diffusion process is recorded. After this process is completed, the ATTGAN model is trained with the recorded data. In addition, in backpropagation, noise prediction is combined with a trained ATTGAN model, and noise is iteratively removed step by step with time steps. Finally, new attack samples are generated based on the features of the original data to obtain a balanced data set. The complete framework of the proposed attack sample generation method is shown in Figure 1.
In a cyber-physical system, data comes from a variety of sources and may include multiple sensors. These data have significant differences in structure, accuracy, transmission delay, and update frequency, so it is necessary to properly preprocess these diverse data. Each column of data in the data set represents a feature of the sensor, and different features correspond to different units of measurement and magnitude, which may lead to the uneven influence of certain attributes in data analysis and model training. In order to balance this dimensional difference and ensure that each attribute is given equal consideration during analysis and training, the minimum-maximum normalization technique is used to linearly transform the data into a specified interval while preserving the original relative order and distribution characteristics of the data. The specific normalization formula is as follows:
yi=xi−min(x)max(x)−min(x) | (9) |
where xi is the eigenvalue of the original data set, yi is the normalized data value, min(x) is the minimum eigenvalue, and max(x) is the maximum eigenvalue. In this way, each feature value xi is converted into a range of 0 to 1, which helps ensure that different features have the same importance in model training, and reduces the impact of dimension and magnitude.
In this section, the diffusion model is combined with the ATTGAN model to complete the forward diffusion and reverse diffusion process of the diffusion model, and finally generate more hidden FDIA attack samples. In the reverse diffusion process, the ATTGAN model is used for noise prediction. The specific structure of the AttDiff model is shown in Figure 2.
Diffusion models [20] usually contain two key stages: the forward diffusion process and reverse diffusion process. In this model, it is necessary to select a data point from the real data distribution as the starting point x0∼q(x), and gradually add Gaussian noise and attack vectors to x0 as the time step increases in the forward diffusion process, we finally turn x0 into standard Gaussian noise xT=N(0,1). For each forward step t∈[1,2,...,T], the noise disturbance is controlled by the following formula:
q(xt|xt−1)=N(xt;√1−βtxt−1,βtI) | (10) |
where {βt∈(0,1)}Tt=1 is a different variance scale. After the forward diffusion ends, the reverse diffusion process gradually reconstructs the original data x0 by sampling xT and learning the diffusion model pθ.
In addition, the parameter t plays a crucial role in the diffusion model, and can directly affect its performance. Therefore, choosing the right t value in our study is crucial to balance the concealment and quality of the generated attack samples. If t is too large, the model may overfit the noise rather than the true distribution of the data, causing the generated attack sample to lose its correlation to the real data. Conversely, if t is too small, the model may not be trained enough to efficiently generate high-quality attack samples. In the experimental part of this study, we find the optimal t value by adjusting the value of t and observing its effect on the quality of the generated sample to ensure that the generated attack sample is both highly concealed and able to effectively challenge the detection model.
For a specific data point x0 extracted from the actual data distribution q(x), this experiment constructs a forward diffusion process. The process involves gradually adding a small amount of Gaussian noise to the sample in T steps along with varying degrees of attack vectors to generate a series of noisy data points x1,...,xT. The noise variance at each step is controlled by {βt∈(0,1)}Tt=1. As the step size t increases, the original data x0 will have fewer and fewer recognizable features. When T→∞, the final data point xT will tend to be an isotropic Gaussian distribution.
In addition, in the forward diffusion process, this experiment extracted data points from normal sample data, and recorded each time step corresponding to the added random noise and the data value before the current state was noised. The purpose is to train the ATTGAN model with the recorded data after the forward diffusion is over. In the process of inverse diffusion, the added noise corresponding to each time step can be predicted and denoised more accurately, so that the attack sample is closer to the real original normal data, and the invisibility of the attack sample is increased.
One benefit of the above process is that we can use the re-parameterization technique. We can sample xt in closed form at any time point t. Here we define αt=1−βt, and ˉαt=t∏i=1αi,i.e., which means xt can be expressed as xt=√ˉαx0+√1−ˉαtε. In this way, we can directly use the initial data point x0 to represent xt.
q(xt|x0)=N(xt;√ˉαtx0,(1−ˉαt)I) | (11) |
By reversing the above forward diffusion process and sampling q(xt−1|xt) at the same time, the real sample data can be recovered from the Gaussian noise input xT∼N(0,I). If the variance βt at each step is small enough, then q(xt−1|xt) will also take on the characteristics of a Gaussian distribution. However, it is important to note that directly estimating q(xt−1|xt) is challenging because it often relies on the entire data set. Therefore, the ATTGAN model trained after the forward diffusion process mentioned above is used in this experiment to predict a noise value by inputting the xt corresponding to the current time step to approximate these conditional probabilities, so as to perform the denoising process of reverse diffusion. According to the nature of the Markov chain, the reverse diffusion process at the current time step t depends only on its previous time step t−1, which means that we have the following formula:
pθ(xt−1|xt)=N(xt−1;μθ(xt,t),∑θ(xt,t)) | (12) |
From this, we can know the overall process of generating samples by the diffusion model. The sample generation process of the diffusion model involves gradually adding noise to the data from front to back until the data is completely transformed into standard Gaussian noise. This process is called forward diffusion. Subsequently, in the reverse diffusion process, by learning how to reverse the operation of adding noise, the noise is gradually removed from the back to the front to restore the original sample data. In this way, new data points can be generated from a limited sample data set, and these newly generated sample data are exactly the data needed at the moment.
The ATTGAN model is mainly composed of generators and discriminators. In this experiment, in order to learn different features of the input data more effectively and improve the sensitivity of the model to specific parts of the input data, an attention mechanism layer is added to the generator, thereby improving the performance of the model.
The model's generator consists of six fully connected layers and is equipped with an appropriate activation function at each layer, such as LeakyReLU, to introduce nonlinear properties. In addition, an attention layer is introduced into the structure of the generator, which consists of three fully connected layers and focuses on the salient parts of the input features through a learnable weight vector w. The output of the attention mechanism is combined with the raw output of the generator to enhance the detail and quality of the data. Note that the design of the attention layer is based on the following formula:
a=softmax(Wa⋅h+ba) | (13) |
where h is the output of the previous layer, Wa and ba are the learnable weights and biases, and softmax functions are used to generate a normalized weight vector a.
The discriminator corresponds to the generator, again consisting of six fully connected layers, each of which is followed by an activation function. The goal of a discriminator is to distinguish between real data and data generated by a generator.
The training of the model follows the standard adversarial training process. In the training process, this experiment adopts binary cross entropy loss (BCELoss) to optimize the model. Generators and discriminators are optimized with the following loss functions:
LG=−Ez∼p(z)[log(D(G(z)))]LD=Ex∼pdata(x)[log(D(x))]+Ez∼p(z)[log(1−D(G(z)))] | (14) |
where LG and LD are the loss functions of the generator and discriminator, respectively, G and D represent the generator and discriminator, respectively, z is the noise vector sampled from the prior distribution p(z), and x is the data point from the real data distribution pdata(x). In this experiment, an Adam optimizer was used to train the model, the learning rate was 0.0001, and the batch size was 64. In the training process, the quality of the generated data and the performance of the discriminator are monitored in real time, and the hyperparameters are carefully adjusted to ensure the effectiveness and stability of the model.
After the forward diffusion of the diffusion model ends, the reverse diffusion process must be followed. Before the reverse diffusion, the ATTGAN model needs to be trained with data recorded during the forward diffusion process described above, which is used for noise prediction in the reverse diffusion. After the model is trained, it officially enters the process of reverse diffusion. Combined with the trained ATTGAN model, it iteratively denoises step by step from the back, and the noise value removed is predicted by the ATTGAN model. In the end, large-scale samples close to the real data will be generated, and these samples are the required attack samples, so that the rare sample library can be expanded to form a balanced data set. Finally, the purpose of improving the detection performance of the detector can be achieved, and then the defense capability of the power system can be enhanced. The overall process of FDIA attack sample generation based on the AttDiff model is shown in Algorithm 1.
Algorithm 1: FDIA Sample Generation Process based on the AttDiff Model | |
Input: | Original measurement data X, number of time steps T; |
Output: | Generated FDIA attack samples XFDIA; |
1 | Initialization the number of time steps T, batch size, and other related parameters; |
2 | Forward Diffusion Process: |
3 | for each time step t = 1 to T do: |
4 | Generate noise ε(t)∼N(0,σ2) and attack vectors of different intensities a; |
5 | Perform iterative calculation of forward diffusion according to Eq 11; |
6 | Record noisy data Xnoise(t)=X+ε(t)+a and current time step t; |
7 | Store the current Xnoise(t) and ε(t) corresponding to t for subsequent ATTGAN model training; |
8 | end for |
9 | Train ATTGAN model using data recorded during forward diffusion process; |
10 | Reverse Diffusion Process: |
11 | for each time step t = T to 1 do: |
12 | Predict noise εhat(t) for Xnoise(t) using the trained ATTGAN model; |
13 | Denoise the data Xdenoised(t)=Xnosie(t)−εhat(t); |
14 | Perform iterative calculation of reverse diffusion according to formula 12; |
15 | Update the data state Xdenoised(t) for the next iteration; |
16 | end for |
17 | Output the final denoised data XFDIA after the last iteration of the reverse diffusion process. |
18 | Return the generated FDIA attack samples XFDIA. |
Among them, steps 1–8 are the forward diffusion stage of the diffusion model. According to the size of the time step, noise and attack vectors are added to the original data by iterating from front to back, and the data state and added noise corresponding to each time step are recorded. Step 9 is the training stage of the ATTGAN model. The data recorded in the process of forward diffusion is used for training. The purpose is to enable the ATTGAN model to effectively focus on power grid measurement values and topological node information, while weakening irrelevant information and fully learning the characteristics of real sample data, so as to play a better role in reverse diffusion. Steps 10–18 are the reverse diffusion stage of the diffusion model. According to the size of the time step, the noisy data is denoised by iterating from back to front. In this process, the diffusion model is combined with the trained ATTGAN model to predict the noise value to be removed, so as to build the attack sample data that is closest to the real sample. Finally, a large number of FDIA attack samples with high quality and high concealment can be obtained.
In this study, three well-known power system test platforms, namely the IEEE 14 bus, IEEE 39 bus, and IEEE 118 bus systems [27,28], were used to evaluate and verify the performance of the proposed method. These bus systems are widely used benchmark models in power system analysis and research. They provide a simplified representation of the power system network, including bus configuration, electrical parameters, generator and load data, etc. Real load data from January 1, 2020, to May 1, 2022, were collected from the New York Independent System Operator (NYISO) as test data. These data cover a time range of more than two years, including seasonal changes throughout the year and load patterns under different weather conditions. By applying these actual load data to the above bus system, we can simulate the measurement data of the power system, and further obtain the load information of each bus state variable and each node, providing real data support for model evaluation.
In this experiment, the above test data are all the data under normal measurement conditions. In order to simulate the real attack behavior, the proposed method is used to attack the test data. A traditional FDIA is a classical method with a certain degree of invisibility and flexibility. The proposed method in this experiment combines the traditional FDIA method to construct an attack sample generation method based on adversarial attention diffusion. By combining the proposed adversarial generation network, the attack sample mixed with normal data features can be generated through reverse diffusion. In order to test the influence of different attack levels, the attack vector is divided into three different attack strengths according to the method in [29]: a) Weak attack: the ratio of the mean power injection deviation in c to x is less than 10%; b) Strong attack: the ratio of the mean power injection deviation in c to x is greater than 30%; c) Medium attack: FDIA samples that do not fall under either of the above attacks.
Since the three bus systems all contain 15,000 pieces of data, the data sets are divided into training sets, verification sets, and test sets according to the ratio of 5:3:2, and experimental comparison tests were carried out under different attack intensity scenarios.
In addition, the experiment uses four indicators, namely precision, recall, accuracy, and F1 score, as the evaluation criteria for the output results, which are defined as follows:
Precision=TPTP+FP | (15) |
Recall=TPTP+FN | (16) |
Accuracy=TP+TNTP+FP+TN+FN | (17) |
F1=2×Precision×RecallPrecision+Recall | (18) |
where true positive (TP) indicates the amount of incorrect data correctly detected, true negative (TN) indicates the amount of normal data detected as normal, false positive (FP) indicates the amount of normal data incorrectly detected as incorrect, and false negative (FN) indicates the amount of incorrect data incorrectly detected as normal. The higher the accuracy and precision, the worse the concealment of the attack sample and the easier it is to be detected.
All experimental simulations in this study were performed on devices with an Intel Core i7-11390H CPU, NVIDIA GeForce MX450, and 16 GB RAM. MATPOWER is used to calculate the power flow and estimate the state of the data in MATLAB, and the proposed AttDiff model is used to generate FDIA attack samples in Python. The noise error of power flow calculation simulation measurement data is set to 0.25 and Gaussian noise with mean zero and standard deviation 1 is adopted. The time step of the diffusion model is set to 4.
In this study, large-scale experiments are carried out on IEEE 14, IEEE 39, and IEEE 118 bus systems to verify the performance of the attack samples generated by the proposed method. Two advanced deep learning detection models are used to detect the generated samples, namely the CNN-based detection model [12] and the LSTM-based detection model [30]. The three types of high, medium, and low attack intensity are subdivided into 9 attack intensities with low intensity is subdivided into 2%, 5%, and 10%, medium intensity is subdivided into 15%, 20%, and 25%, and high intensity is subdivided into 30%, 40%, and 50% for comparison. The corresponding experimental results are shown in Tables 1-6, in which Tables 1 and 2 are the test results of the IEEE 14 bus system, Tables 3 and 4 are the test results of the IEEE 39 bus system, and Tables 5 and 6 are the test results of the IEEE 118 bus system.
Attack Level | Attack Strength | Traditional FDIA [8] | LMM-FDIA [18] | AttDiff-FDIA | ||||||||
Precision | Recall | F1-Score | Precision | Recall | F1-Score | Precision | Recall | F1-Score | ||||
Weak | 2% | 0.6883 | 0.6992 | 0.6819 | 0.6740 | 0.6958 | 0.6730 | 0.6469 | 0.7548 | 0.6892 | ||
5% | 0.7289 | 0.7233 | 0.7130 | 0.6831 | 0.7482 | 0.7045 | 0.6664 | 0.7173 | 0.6755 | |||
10% | 0.7601 | 0.7829 | 0.7602 | 0.7079 | 0.6778 | 0.6768 | 0.6937 | 0.7017 | 0.6860 | |||
Medium | 15% | 0.8205 | 0.8101 | 0.8062 | 0.7351 | 0.6716 | 0.6867 | 0.7081 | 0.6757 | 0.6777 | ||
20% | 0.8469 | 0.8647 | 0.8483 | 0.7514 | 0.7095 | 0.7146 | 0.7129 | 0.6529 | 0.6661 | |||
25% | 0.8772 | 0.8817 | 0.8731 | 0.7656 | 0.6992 | 0.7159 | 0.7209 | 0.6941 | 0.6958 | |||
Strong | 30% | 0.9092 | 0.9111 | 0.9050 | 0.7857 | 0.7062 | 0.7314 | 0.7324 | 0.7014 | 0.7037 | ||
40% | 0.9223 | 0.9423 | 0.9289 | 0.7995 | 0.7286 | 0.7503 | 0.7561 | 0.7280 | 0.7297 | |||
50% | 0.9313 | 0.9490 | 0.9371 | 0.8117 | 0.7547 | 0.7702 | 0.7789 | 0.7156 | 0.7339 |
Attack Level | Attac Strength | Traditional FDIA [8] | LMM-FDIA [18] | AttDiff-FDIA | ||||||||
Precision | Recall | F1-Score | Precision | Recall | F1-Score | Precision | Recall | F1-Score | ||||
Weak | 2% | 0.7038 | 0.7208 | 0.7117 | 0.6859 | 0.7404 | 0.7119 | 0.6669 | 0.7540 | 0.7064 | ||
5% | 0.7167 | 0.7437 | 0.7298 | 0.6927 | 0.7707 | 0.7293 | 0.6828 | 0.7456 | 0.7127 | |||
10% | 0.7671 | 0.7915 | 0.7602 | 0.7235 | 0.7583 | 0.7404 | 0.6972 | 0.7551 | 0.7248 | |||
Medium | 15% | 0.7958 | 0.8235 | 0.8062 | 0.7431 | 0.7351 | 0.7383 | 0.7203 | 0.6961 | 0.7060 | ||
20% | 0.8277 | 0.8976 | 0.8610 | 0.7547 | 0.6959 | 0.7240 | 0.7220 | 0.6695 | 0.6935 | |||
25% | 0.8679 | 0.9059 | 0.8864 | 0.7565 | 0.7253 | 0.7402 | 0.7334 | 0.6325 | 0.6787 | |||
Strong | 30% | 0.9075 | 0.9317 | 0.9194 | 0.7715 | 0.7086 | 0.7379 | 0.743 | 0.6395 | 0.6862 | ||
40% | 0.9484 | 0.9361 | 0.9421 | 0.8039 | 0.7286 | 0.7641 | 0.7651 | 0.6792 | 0.7190 | |||
50% | 0.9601 | 0.8864 | 0.9213 | 0.8248 | 0.6981 | 0.7558 | 0.7957 | 0.7144 | 0.7527 |
Attack Level | Attack Strength | Traditional FDIA [8] | LMM-FDIA [18] | AttDiff-FDIA | ||||||||
Precision | Recall | F1-Score | Precision | Recall | F1-Score | Precision | Recall | F1-Score | ||||
Weak | 2% | 0.7187 | 0.7181 | 0.7078 | 0.7199 | 0.7223 | 0.7074 | 0.6934 | 0.6977 | 0.6815 | ||
5% | 0.7664 | 0.7799 | 0.7650 | 0.7200 | 0.7521 | 0.7242 | 0.7042 | 0.7200 | 0.6990 | |||
10% | 0.8594 | 0.8605 | 0.8549 | 0.7404 | 0.7263 | 0.7215 | 0.7208 | 0.7177 | 0.7074 | |||
Medium | 15% | 0.9094 | 0.9250 | 0.9141 | 0.7549 | 0.7109 | 0.7159 | 0.7119 | 0.7214 | 0.7034 | ||
20% | 0.9507 | 0.9437 | 0.9452 | 0.7672 | 0.7272 | 0.7342 | 0.7205 | 0.7132 | 0.7052 | |||
25% | 0.9737 | 0.9609 | 0.9660 | 0.7743 | 0.7122 | 0.7285 | 0.7309 | 0.7130 | 0.7082 | |||
Strong | 30% | 0.9801 | 0.9789 | 0.9787 | 0.7850 | 0.7346 | 0.7485 | 0.7439 | 0.7227 | 0.7211 | ||
40% | 0.9922 | 0.9817 | 0.9864 | 0.7836 | 0.7219 | 0.7385 | 0.7629 | 0.7115 | 0.7247 | |||
50% | 0.9919 | 0.9900 | 0.9906 | 0.8051 | 0.7451 | 0.7639 | 0.7943 | 0.7208 | 0.7456 |
Attack Level | Attack Strength | Traditional FDIA [8] | LMM-FDIA [18] | AttDiff-FDIA | ||||||||
Precision | Recall | F1-Score | Precision | Recall | F1-Score | Precision | Recall | F1-Score | ||||
Weak | 2% | 0.7414 | 0.7723 | 0.7555 | 0.7737 | 0.7234 | 0.7472 | 0.7180 | 0.7508 | 0.7328 | ||
5% | 0.8171 | 0.9069 | 0.8593 | 0.7976 | 0.8309 | 0.8138 | 0.7277 | 0.6972 | 0.7112 | |||
10% | 0.8814 | 0.9246 | 0.9011 | 0.8270 | 0.7857 | 0.8054 | 0.7461 | 0.7098 | 0.7273 | |||
Medium | 15% | 0.9326 | 0.9334 | 0.9325 | 0.8317 | 0.8007 | 0.8157 | 0.7526 | 0.7548 | 0.7533 | ||
20% | 0.9562 | 0.9651 | 0.9605 | 0.8549 | 0.7868 | 0.8194 | 0.7721 | 0.7269 | 0.7485 | |||
25% | 0.9835 | 0.9819 | 0.9826 | 0.8705 | 0.8009 | 0.8340 | 0.7866 | 0.7460 | 0.7656 | |||
Strong | 30% | 0.9867 | 0.9881 | 0.9874 | 0.8828 | 0.8098 | 0.8442 | 0.8135 | 0.7936 | 0.8024 | ||
40% | 0.9993 | 0.9954 | 0.9973 | 0.9039 | 0.7797 | 0.8371 | 0.8417 | 0.7673 | 0.8024 | |||
50% | 1.0000 | 0.9987 | 0.9993 | 0.9234 | 0.7419 | 0.8220 | 0.8860 | 0.7651 | 0.8195 |
Attack Level | Attack Strength | Traditional FDIA [8] | LMM-FDIA [18] | AttDiff-FDIA | ||||||||
Precision | Recall | F1-Score | Precision | Recall | F1-Score | Precision | Recall | F1-Score | ||||
Weak | 2% | 0.7134 | 0.7293 | 0.710 | 0.6996 | 0.7330 | 0.7039 | 0.6880 | 0.7415 | 0.7043 | ||
5% | 0.7462 | 0.7644 | 0.7455 | 0.7174 | 0.7304 | 0.7107 | 0.7035 | 0.7280 | 0.7039 | |||
10% | 0.8235 | 0.8068 | 0.8072 | 0.7407 | 0.7309 | 0.7208 | 0.7106 | 0.7240 | 0.7049 | |||
Medium | 15% | 0.8652 | 0.8707 | 0.8628 | 0.7644 | 0.7457 | 0.7439 | 0.7106 | 0.7357 | 0.7118 | ||
20% | 0.9282 | 0.9162 | 0.9190 | 0.7856 | 0.7318 | 0.7461 | 0.7329 | 0.7134 | 0.7118 | |||
25% | 0.9493 | 0.9322 | 0.9382 | 0.7990 | 0.7221 | 0.7467 | 0.7424 | 0.7187 | 0.7194 | |||
Strong | 30% | 0.9571 | 0.9603 | 0.9571 | 0.8110 | 0.7554 | 0.7733 | 0.7513 | 0.7469 | 0.7381 | ||
40% | 0.9746 | 0.9741 | 0.9734 | 0.8227 | 0.7357 | 0.7691 | 0.7991 | 0.7441 | 0.7601 | |||
50% | 0.9836 | 0.9773 | 0.9797 | 0.8422 | 0.7668 | 0.7925 | 0.8364 | 0.7577 | 0.7861 |
Attack Level | Attack Strength | Traditional FDIA [8] | LMM-FDIA [18] | AttDiff-FDIA | ||||||||
Precision | Recall | F1-Score | Precision | Recall | F1-Score | Precision | Recall | F1-Score | ||||
Weak | 2% | 0.7263 | 0.6764 | 0.6960 | 0.7159 | 0.8198 | 0.7640 | 0.6963 | 0.7457 | 0.7192 | ||
5% | 0.7541 | 0.7241 | 0.7382 | 0.7353 | 0.8149 | 0.7730 | 0.7179 | 0.6841 | 0.6994 | |||
10% | 0.8359 | 0.8269 | 0.8305 | 0.7637 | 0.8015 | 0.7819 | 0.7204 | 0.6691 | 0.6928 | |||
Medium | 15% | 0.8815 | 0.8458 | 0.8630 | 0.7829 | 0.7701 | 0.7761 | 0.7219 | 0.6527 | 0.6853 | ||
20% | 0.9153 | 0.9364 | 0.9255 | 0.7971 | 0.7372 | 0.7657 | 0.7323 | 0.6584 | 0.6920 | |||
25% | 0.9341 | 0.9515 | 0.9427 | 0.8090 | 0.7355 | 0.7704 | 0.7456 | 0.7242 | 0.7327 | |||
Strong | 30% | 0.9538 | 0.9624 | 0.9580 | 0.8270 | 0.7113 | 0.7648 | 0.7608 | 0.7020 | 0.7272 | ||
40% | 0.9776 | 0.9731 | 0.9753 | 0.8409 | 0.7082 | 0.7688 | 0.8002 | 0.7195 | 0.7574 | |||
50% | 0.9873 | 0.9737 | 0.9804 | 0.8630 | 0.7151 | 0.7812 | 0.8269 | 0.7501 | 0.7861 |
As can be seen from the table above, the attack sample generation method proposed in this experiment can obtain the lowest accuracy and lower F1 score under different attack intensifications. When the CNN-based FDIA detection on the IEEE 14 bus system is used for low-intensity attacks of 5%, the proposed method has a precision reduction of 6.256% and 1.676% compared with the traditional FDIA [8] method and the hybrid Laplace method [18], and a score reduction of 3.744% and 2.896% in F1, respectively. For medium-intensity attacks of 20%, the proposed method has a precision reduction of 13.4% and 3.854% compared with the traditional FDIA [8] method and the mixed Laplacian method [18], and a score reduction of 18.22% and 4.852% in F1, respectively. For high-intensity attacks of 40%, the proposed method has a precision reduction of 16.63% and 4.344% compared with the traditional FDIA [8] method and hybrid Laplacian method [18], and a score reduction of 19.91% and 2.06% in F1, respectively.
In addition, on the IEEE 39 and IEEE 118 bus systems, both the accuracy and F1 score achieved the lowest values under the three attack intensities. This is enough to show that the samples generated by the method proposed in this experiment have a stronger ability to evade detection, and have better concealment and attack capabilities. In the comparison of the three methods, the reason why the method proposed in this experiment can achieve better performance results mainly depends on the following two reasons: First, this experiment introduces an attention mechanism in the application of the diffusion model, which makes the model itself pay more attention to the node features that have a greater impact on the results, thereby promoting the model's efficient learning of node data features; and second, by combining adversarial training in the entire diffusion process, it helps the model learn more robust feature representations, improves the generalization ability of the model, and then generates high-quality, indistinguishable attack sample data.
The main framework adopted in this experiment is the diffusion model. As we all know, the diffusion model is closely related to the time step, and the performance of the samples generated by the diffusion model is also closely related to the time step. In order to verify that the time step used is the most cost-effective value, a series of experiments were conducted to observe the effect of different time steps on the detection results of the final generated attack samples. Comparative experiments were carried out on IEEE 14, IEEE 39, and IEEE 118 bus systems using detection models based on CNN and LSTM. The result is shown in Figure 3.
This comparative experiment discusses the cases where the time step of the diffusion model is set to 2, 4, 6, 8, and 10, respectively. From the above experimental results, it can be seen that the detection accuracy on the three bus systems will decrease slightly with the increase of the time step. On the IEEE 14 bus test system, the detection accuracy of the CNN detector at different time steps is 0.6953, 0.6715, 0.6751, 0.673, and 0.6679, respectively. The detection accuracy of the LSTM detector at different time steps is 0.7051, 0.6788, 0.6744, 0.6727, and 0.6681, respectively. When the time step is 2, the detection accuracy based on the two detectors is the highest, and when the time step is 10, the detection accuracy is the lowest. However, when the time steps are 4, 6, 8, and 10, the accuracy only decreases in a small range, and there is not much difference.
In addition, for the comparison of the IEEE 39 and IEEE 118 bus systems, the detection accuracy based on the two detectors eventually showed a downward trend. For the diffusion model, the time step has a direct impact on the final detection result. Within a reasonable range, the larger the time step, the more detailed control the model will have, and the more opportunities to learn and approximate the true distribution of the data, and better capture and recover the complex patterns and structures in the data, so as to improve the diversity and authenticity of the generated samples. However, a larger the time step is not the better, because the increase of the time step will lead to a large increase in the amount of computation and training time, and too high of a time step may also cause the model to overfit the training data, and can not be well generalized to new data. In order to guarantee the quality of attack samples, the calculation cost is reduced as much as possible by analyzing the comparative experiments on three bus systems with different time steps. Therefore, the most cost-effective time step setting was chosen for this experiment, that is, the time step was set to 4. This setting can not only effectively control the time overhead, but also ensure the high quality of the generated samples.
In order to verify the reliability of the proposed sample generation method, a series of experiments were carried out to study the influence of different noise disturbances on the measured data of the power system. Because the actual power system measurement data is often disturbed by noise, it is crucial to understand the impact of these disturbances on the proposed approach. This experiment simulates this kind of interference by adding Gaussian noise with different variance to the data of each node in the power system. In addition, on the IEEE 14, IEEE 39, and IEEE 118 bus systems, the detection models based on CNN and LSTM were used to conduct comparative tests on the attack samples generated by the traditional FDIA method, the mixed Laplacian method, and the method proposed in this experiment. The test results are shown in the figure below. Figures 4, 5, and 6 are the tests based on the IEEE 14 bus system, the IEEE 39 bus system, and the IEEE 118 bus system, respectively.
In this comparative experiment, seven different noise variance levels were set for the measurement data: 0.25, 0.30, 0.35, 0.40, 0.45, 0.50, and 0.55. By observing the above experimental results, it can be found that the precision of all models shows a decreasing trend with the increase of the noise variance level. Therefore, the noise variance level in the simulated power grid environment in this study is set at 0.25. This decrease in detection accuracy is mainly due to the fact that the increase in noise variance makes it more difficult to distinguish between normal and attack samples. Noise interference makes it easier for pure data to be masked by it. In addition, the experimental data also show that under the two detection models, the attack sample generation method proposed in this experiment performs better than the traditional FDIA sample generation method and the mixed Laplacian sample generation method under various noise levels. This proves that the sample generation method proposed in this study has more obvious advantages over the existing technology in terms of robustness and adaptability. In general, the method proposed in this paper can generate more stable and anti-jamming FDIA attack samples, and has stronger ability to evade detection. This provides a large number of efficient attack sample resources for constructing and training FDIA detection models based on deep learning and AI.
The existing FDIA detection methods have been limited by the problem of sample scarcity and data set imbalance. This paper presents a method to generate FDIA attack samples using an adversarial attention diffusion model. The overall model of the method consists of two parts: the diffusion model and GAN model with an attention mechanism (ATTGAN). First, the original power flow data is noised by the forward diffusion of the diffusion model combined with the time step. In this process, the original measurement data and added noise corresponding to each time step are recorded for the subsequent model training. After the forward diffusion is completed, the data recorded during the forward diffusion process is used to train the proposed ATTGAN model, which is used for noise prediction during the reverse diffusion process. Finally, in the reverse diffusion process, the trained ATTGAN model is combined with the diffusion model, and according to the size of the time step, the iterative denoising is done step by step from back to front to generate FDIA attack samples with stronger concealment and attack capabilities. This experiment was conducted on a large scale on the IEEE 14, IEEE 39, and IEEE 118 bus systems. The experimental results show that the attack samples generated by the proposed sample generation method have better ability to evade detection after detection by two kinds of detectors, thus effectively reducing the detection precision and accuracy.
Although our proposed attention-diffusion method can generate more covert and disguised attack samples, thereby expanding the attack sample base, it also has some limitations. The main limitation is the relatively large time overhead of the model, which may affect its efficiency in real-time applications. In addition, model performance is sensitive to parameter selection and requires careful tuning to achieve optimal performance, potentially adding complexity to model deployment. To overcome these limitations, future work could explore algorithmic optimization and hardware acceleration to improve the computational efficiency of the model, as well as the application of hyperparameter optimization techniques to automate the parameter selection process to reduce the effort of manual adjustment and potentially discover a better combination of parameters. Future work could also further explore the explainability of the model, improve user trust in the model output, and extend the model's application to support broader smart grid security research.
The authors declare they have not used Artificial Intelligence (AI) tools in the creation of this article.
This research is supported by grants from the Engineering Research Center of Offshore Wind Technology Ministry of Education (Shanghai University of Electric Power).
The authors declare no conflicts of interest.
Conceptualization, Kunzhan Li; methodology, Kunzhan Li and Fengyong Li; validation, Kunzhan Li; formal analysis, Fengyong Li and Baonan Wang; writing—original draft preparation, Kunzhan Li; writing—review and editing, Fengyong Li and Baonan Wang; supervision, Meijing Shan. All authors have read and agreed to the published version of the manuscript.
[1] |
Matarrita-Cascante D, Brennan MA, Luloff A (2010) Community agency and sustainable tourism development: The case of La Fortuna, Costa Rica. J Sustain Tour 18: 735–756. https://doi.org/10.1080/09669581003653526 doi: 10.1080/09669581003653526
![]() |
[2] | Evans G (2005) Measure for measure: Evaluating the evidence of culture's contribution to regeneration. Urban Stud 42: 959–983. Available from: http://www.jstor.org/stable/43197307. |
[3] |
Lin CP, Chen SH, Trac LVT, et al. (2021) An expert-knowledge-based model for evaluating cultural tourism strategies: A case of Tainan City, Taiwan. J Hosp Tour Manag 49: 214–225. https://doi.org/10.1016/j.jhtm.2021.08.020 doi: 10.1016/j.jhtm.2021.08.020
![]() |
[4] |
Emery M, Flora C (2006) Spiraling-up: Mapping community transformation with community capitals framework. Community Dev 37: 19–35. https://doi.org/10.1080/15575330609490152 doi: 10.1080/15575330609490152
![]() |
[5] |
Della Corte V, Savastano I, Storlazzi A (2009) Service innovation in cultural heritages management and valorization. Int J Qual Serv Sci 1: 225–240. https://doi.org/10.1108/17566690911004177 doi: 10.1108/17566690911004177
![]() |
[6] |
Chen T, Park H, Rajwani T (2024) Diverse human resource slack and firm innovation: Evidence from politically connected firms. Int Bus Rev 33: 102244. https://doi.org/10.1016/j.ibusrev.2023.102244 doi: 10.1016/j.ibusrev.2023.102244
![]() |
[7] |
Berger AN, Karakaplan MU, Roman RA (2023) Whose bailout is it anyway? The roles of politics in PPP bailouts of small businesses vs. banks. J Financial Intermediation 56: 101044. https://doi.org/10.1016/j.jfi.2023.101044 doi: 10.1016/j.jfi.2023.101044
![]() |
[8] |
Hodge GA, Greve C (2007) Public–private partnerships: An international performance review. Public Adm Rev 67: 545–558. https://doi.org/10.1111/j.1540-6210.2007.00736.x doi: 10.1111/j.1540-6210.2007.00736.x
![]() |
[9] |
Osei-Kyei R, Chan APC (2015) Review of studies on the Critical Success Factors for Public–Private Partnership (PPP) projects from 1990 to 2013. Int J Project Manage 33: 1335–1346. https://doi.org/10.1016/j.ijproman.2015.02.008 doi: 10.1016/j.ijproman.2015.02.008
![]() |
[10] |
Ostrom E (1998) A behavioral approach to the rational choice theory of collective action: Presidential address, American Political Science Association, 1997. Am Polit Sci Rev 92: 1–22. https://doi.org/10.2307/2585925 doi: 10.2307/2585925
![]() |
[11] |
Kim H, Kim H, Woosnam KM (2023) Collaborative governance and conflict management in cultural heritage-led regeneration projects: The case of urban Korea. Habitat Int 134: 102767. https://doi.org/10.1016/j.habitatint.2023.102767 doi: 10.1016/j.habitatint.2023.102767
![]() |
[12] |
Kim H, Kim H, Woosnam KM (2023) Considering urban regeneration policy support: Perceived collaborative governance in cultural heritage-led regeneration projects of Korea. Habitat Int 140: 102921. https://doi.org/10.1016/j.habitatint.2023.102921 doi: 10.1016/j.habitatint.2023.102921
![]() |
[13] |
Li Z, Lin Y, Hooimeijer P, et al. (2024) Heritage conflict evolution: Changing framing strategies and opportunity structures in two heritage district redevelopment projects in China. Geoforum 149: 103959. https://doi.org/10.1016/j.geoforum.2024.103959 doi: 10.1016/j.geoforum.2024.103959
![]() |
[14] | Smith L (2006) Uses of Heritage, London: Routledge. https://doi.org/10.4324/9780203602263 |
[15] | Casey KL (2005) Defining political capital: A reconsideration of Bourdieu's interconvertibility theory. |
[16] | Cleere H (2012) Archaeological Heritage Management in the Modern World, London: Routledge. https://doi.org/10.4324/9780203060223 |
[17] | Pine BJ, Gilmore JH (2011) The Experience Economy, Boston: Harvard Business Press. |
[18] |
Chen CL (2022) Strategic sustainable service design for creative-cultural hotels: A multi-level and multi-domain view. Local Environ 27: 46–79. https://doi.org/10.1080/13549839.2021.2001796 doi: 10.1080/13549839.2021.2001796
![]() |
[19] |
Chen CL (2021) Cultural product innovation strategies adopted by the performing arts industry. Rev Manag Sci 15: 1139–1171. https://doi.org/10.1007/s11846-020-00393-1 doi: 10.1007/s11846-020-00393-1
![]() |
[20] |
Chen JS, Kerr D, Chou CY, et al. (2017) Business co-creation for service innovation in the hospitality and tourism industry. Int J Contemp Hosp Manag 29: 1522–1540. https://doi.org/10.1108/IJCHM-06-2015-0308 doi: 10.1108/IJCHM-06-2015-0308
![]() |
[21] |
Hincapié M, Díaz C, Zapata-Cárdenas MI, et al. (2021) Augmented reality mobile apps for cultural heritage reactivation. Comput Electr Eng 93: 107281. https://doi.org/10.1016/j.compeleceng.2021.107281 doi: 10.1016/j.compeleceng.2021.107281
![]() |
[22] |
Lyu Y, Abd Malek MI, Jaafar NH, et al. (2023) Unveiling the potential of space syntax approach for revitalizing historic urban areas: A case study of Yushan Historic District, China. Front Archit Res 12: 1144–1156. https://doi.org/10.1016/j.foar.2023.08.004 doi: 10.1016/j.foar.2023.08.004
![]() |
[23] |
Vargo SL, Lusch RF (2017) Service-dominant logic 2025. Int J Res Mark 34: 46–67. https://doi.org/10.1016/j.ijresmar.2016.11.001 doi: 10.1016/j.ijresmar.2016.11.001
![]() |
[24] |
Aal K, Di Pietro L, Edvardsson B, et al. (2016) Innovation in service ecosystems. J Serv Manag 27: 619–651. https://doi.org/10.1108/JOSM-02-2015-0044 doi: 10.1108/JOSM-02-2015-0044
![]() |
[25] |
Vargo SL, Lusch RF (2008) Service-dominant logic: Continuing the evolution. J Acad Mark Sci 36: 1–10. https://doi.org/10.1007/s11747-007-0069-6 doi: 10.1007/s11747-007-0069-6
![]() |
[26] |
Hörger C, Ward P (2023) Coordination mechanisms and the role of taskscape in value co-creation: The British 'milkman'. J Bus Res 162: 113849. https://doi.org/10.1016/j.jbusres.2023.113849 doi: 10.1016/j.jbusres.2023.113849
![]() |
[27] |
Vargo SL (2020) From promise to perspective: Reconsidering value propositions from a service-dominant logic orientation. Ind Mark Manag 87: 309–311. https://doi.org/10.1016/j.indmarman.2019.10.013 doi: 10.1016/j.indmarman.2019.10.013
![]() |
[28] |
Bennett N, Lemelin RH, Koster R, et al. (2012) A capital assets framework for appraising and building capacity for tourism development in aboriginal protected area gateway communities. Tour Manag 33: 752–766. https://doi.org/https://doi.org/10.1016/j.tourman.2011.08.009 doi: 10.1016/j.tourman.2011.08.009
![]() |
[29] |
Flora CB, Flora JL (1993) Entrepreneurial social infrastructure: A necessary ingredient. Ann Am Acad Polit Soc Sci 529: 48–58. https://doi.org/10.1177/000271629352900100 doi: 10.1177/000271629352900100
![]() |
[30] |
Hale J, Irish A, Carolan M, et al. (2023) A systematic review of cultural capital in US community development research. J Rural Stud 103: 103113. https://doi.org/10.1016/j.jrurstud.2023.103113 doi: 10.1016/j.jrurstud.2023.103113
![]() |
[31] | Flora CB (2016) Rural Communities: Legacy+ Change, New York: Routledge. https://doi.org/10.4324/9780429494697 |
[32] | Green GP, Haines A (2016) Asset Building & Community Development, Sage publications. https://doi.org/10.4135/9781483398631 |
[33] |
Turner RS (1999) Entrepreneurial neighborhood initiatives: Political capital in community development. Econ Dev Q 13: 15–22. https://doi.org/10.1177/089124249901300103 doi: 10.1177/089124249901300103
![]() |
[34] |
McDonald C, Kirk-Brown A, Frost L, et al. (2013) Partnerships and integrated responses to rural decline: The role of collective efficacy and political capital in Northwest Tasmania, Australia. J Rural Stud 32: 346–356. https://doi.org/10.1016/j.jrurstud.2013.08.003 doi: 10.1016/j.jrurstud.2013.08.003
![]() |
[35] |
Núñez APB, Gutiérrez-Montes I, Hernández-Núñez HE, et al. (2023) Diverse farmer livelihoods increase resilience to climate variability in southern Colombia. Land Use Policy 131: 106731. https://doi.org/10.1016/j.landusepol.2023.106731 doi: 10.1016/j.landusepol.2023.106731
![]() |
[36] |
Elsahn ZF, Benson-Rea M (2018) Political schemas and corporate political activities during foreign market entry: A micro-process perspective. Manag Int Rev 58: 771–811. https://doi.org/10.1007/s11575-018-0350-6 doi: 10.1007/s11575-018-0350-6
![]() |
[37] |
Xia T, Liu X (2022) The innovation paradox of TMT political capital in transition economy firms. J Bus Res 142: 775–790. https://doi.org/10.1016/j.jbusres.2022.01.011 doi: 10.1016/j.jbusres.2022.01.011
![]() |
[38] |
Aquino RS, Lück M, Schänzel HA (2018) A conceptual framework of tourism social entrepreneurship for sustainable community development. J Hosp Tour Manag 37: 23–32. https://doi.org/https://doi.org/10.1016/j.jhtm.2018.09.001 doi: 10.1016/j.jhtm.2018.09.001
![]() |
[39] |
Pigg K, Gasteyer S, Martin K, et al. (2013) The community capitals framework: An empirical examination of internal relationships. Community Dev 44: 492–502. http://dx.doi.org/10.1080/15575330.2013.814698 doi: 10.1080/15575330.2013.814698
![]() |
[40] |
Sørensen JFL, Svendsen GLH (2023) What makes peripheral places matter? Applying the concept of political capital within a multiple capital framework. J Rural Stud 103: 103136. https://doi.org/10.1016/j.jrurstud.2023.103136 doi: 10.1016/j.jrurstud.2023.103136
![]() |
[41] |
Zekeri AA (2013) Community capital and local economic development efforts. Prof Agric Workers J 1. http://dx.doi.org/10.22004/ag.econ.236728 doi: 10.22004/ag.econ.236728
![]() |
[42] |
Jung TH, Lee J, Yap MH, et al. (2015) The role of stakeholder collaboration in culture-led urban regeneration: A case study of the Gwangju project, Korea. Cities 44: 29–39. https://doi.org/10.1016/j.cities.2014.12.003 doi: 10.1016/j.cities.2014.12.003
![]() |
[43] |
Montalto V, Alberti V, Panella F, et al. (2023) Are cultural cities always creative? An empirical analysis of culture-led development in 190 European cities. Habitat Int 132: 102739. https://doi.org/10.1016/j.habitatint.2022.102739 doi: 10.1016/j.habitatint.2022.102739
![]() |
[44] | Carroll P, Steane P (2000) Public-private partnerships: Sectoral perspectives, In: Public-Private Partnerships, Routledge, 54–74. |
[45] |
Azarian M, Shiferaw AT, Lædre O, et al. (2023) Project ownership in public-private partnership (PPP) projects of Norway. Procedia Comput Sci 219: 1838–1846. https://doi.org/10.1016/j.procs.2023.01.481 doi: 10.1016/j.procs.2023.01.481
![]() |
[46] |
Brown TL, Potoski M, Van Slyke DM (2006) Managing public service contracts: Aligning values, institutions, and markets. Public Adm Rev 66: 323–331. https://doi.org/https://doi.org/10.1111/j.1540-6210.2006.00590.x00 doi: 10.1111/j.1540-6210.2006.00590.x00
![]() |
[47] |
Zhao Y (2015) 'China's leading historical and cultural city': Branding Dali City through public–private partnerships in Bai architecture revitalization. Cities 49: 106–112. https://doi.org/10.1016/j.cities.2015.07.009 doi: 10.1016/j.cities.2015.07.009
![]() |
[48] |
Rufin C, Rivera-Santos M (2012) Between commonweal and competition: Understanding the governance of public–private partnerships. J Manag 38: 1634–1654. https://doi.org/10.1177/0149206310373948 doi: 10.1177/0149206310373948
![]() |
[49] | Stephen O (2000) Public-Private Partnerships: Theory and Practice in International Perspective. London: Routledge. https://doi.org/10.4324/9780203207116 |
[50] |
Robaczewska J, Vanhaverbeke W, Lorenz A (2019) Applying open innovation strategies in the context of a regional innovation ecosystem: The case of Janssen Pharmaceuticals. Glob Transit 1: 120–131. https://doi.org/10.1016/j.glt.2019.05.001 doi: 10.1016/j.glt.2019.05.001
![]() |
[51] |
Absalyamov T (2015) Tatarstan model of public-private partnership in the field of cultural heritage preservation. Procedia: Soc Behav Sci 188: 214–217. https://doi.org/10.1016/j.sbspro.2015.03.375 doi: 10.1016/j.sbspro.2015.03.375
![]() |
[52] |
Rossetti G, Quinn B (2021) Understanding the cultural potential of rural festivals: A conceptual framework of cultural capital development. J Rural Stud 86: 46–53. https://doi.org/10.1016/j.jrurstud.2021.05.009 doi: 10.1016/j.jrurstud.2021.05.009
![]() |
[53] | Yin RK (2009) Case Study Research: Design and Methods, Sage Publications. |
[54] | Stake RE (1995) Case Study Research, Thousand Oaks, CA: Sage Publications. |
[55] | Patton MQ (2014) Qualitative Research & Evaluation Methods: Integrating Theory and Practice: Thousand Oaks, CA: SAGE Publications. |
[56] | Denzin NK (2017) The Research Act: A Theoretical Introduction to Sociological Methods, New York: Routledge. https://doi.org/10.4324/9781315134543 |
[57] |
Lin CL (2019) Establishing environment sustentation strategies for urban and rural/town tourism based on a hybrid MCDM approach. Curr Issues Tour 23: 2360–2395. https://doi.org/10.1080/13683500.2019.1642308 doi: 10.1080/13683500.2019.1642308
![]() |
[58] |
Berry LL, Zeithaml VA, Parasuraman A (1985) Quality counts in services, too. Bus Horiz 28: 44–52. https://doi.org/10.1016/0007-6813(85)90008-4 doi: 10.1016/0007-6813(85)90008-4
![]() |
[59] |
Nishant R, Kennedy M, Corbett J (2020) Artificial intelligence for sustainability: Challenges, opportunities, and a research agenda. Int J Inf Manage 53: 102104. https://doi.org/10.1016/j.ijinfomgt.2020.102104 doi: 10.1016/j.ijinfomgt.2020.102104
![]() |
1. | Qianlan Li, Qingdan Wu, Xiaochen Zheng, Pengfei Wang, Dongsheng Zou, Fen Liu, Zhihua Xiao, The conversion and migration behavior of phosphorus speciation during pyrolysis of different sludges, 2024, 11, 2372-0352, 1, 10.3934/environsci.2024001 |
Attack Level | Attack Strength | Traditional FDIA [8] | LMM-FDIA [18] | AttDiff-FDIA | ||||||||
Precision | Recall | F1-Score | Precision | Recall | F1-Score | Precision | Recall | F1-Score | ||||
Weak | 2% | 0.6883 | 0.6992 | 0.6819 | 0.6740 | 0.6958 | 0.6730 | 0.6469 | 0.7548 | 0.6892 | ||
5% | 0.7289 | 0.7233 | 0.7130 | 0.6831 | 0.7482 | 0.7045 | 0.6664 | 0.7173 | 0.6755 | |||
10% | 0.7601 | 0.7829 | 0.7602 | 0.7079 | 0.6778 | 0.6768 | 0.6937 | 0.7017 | 0.6860 | |||
Medium | 15% | 0.8205 | 0.8101 | 0.8062 | 0.7351 | 0.6716 | 0.6867 | 0.7081 | 0.6757 | 0.6777 | ||
20% | 0.8469 | 0.8647 | 0.8483 | 0.7514 | 0.7095 | 0.7146 | 0.7129 | 0.6529 | 0.6661 | |||
25% | 0.8772 | 0.8817 | 0.8731 | 0.7656 | 0.6992 | 0.7159 | 0.7209 | 0.6941 | 0.6958 | |||
Strong | 30% | 0.9092 | 0.9111 | 0.9050 | 0.7857 | 0.7062 | 0.7314 | 0.7324 | 0.7014 | 0.7037 | ||
40% | 0.9223 | 0.9423 | 0.9289 | 0.7995 | 0.7286 | 0.7503 | 0.7561 | 0.7280 | 0.7297 | |||
50% | 0.9313 | 0.9490 | 0.9371 | 0.8117 | 0.7547 | 0.7702 | 0.7789 | 0.7156 | 0.7339 |
Attack Level | Attac Strength | Traditional FDIA [8] | LMM-FDIA [18] | AttDiff-FDIA | ||||||||
Precision | Recall | F1-Score | Precision | Recall | F1-Score | Precision | Recall | F1-Score | ||||
Weak | 2% | 0.7038 | 0.7208 | 0.7117 | 0.6859 | 0.7404 | 0.7119 | 0.6669 | 0.7540 | 0.7064 | ||
5% | 0.7167 | 0.7437 | 0.7298 | 0.6927 | 0.7707 | 0.7293 | 0.6828 | 0.7456 | 0.7127 | |||
10% | 0.7671 | 0.7915 | 0.7602 | 0.7235 | 0.7583 | 0.7404 | 0.6972 | 0.7551 | 0.7248 | |||
Medium | 15% | 0.7958 | 0.8235 | 0.8062 | 0.7431 | 0.7351 | 0.7383 | 0.7203 | 0.6961 | 0.7060 | ||
20% | 0.8277 | 0.8976 | 0.8610 | 0.7547 | 0.6959 | 0.7240 | 0.7220 | 0.6695 | 0.6935 | |||
25% | 0.8679 | 0.9059 | 0.8864 | 0.7565 | 0.7253 | 0.7402 | 0.7334 | 0.6325 | 0.6787 | |||
Strong | 30% | 0.9075 | 0.9317 | 0.9194 | 0.7715 | 0.7086 | 0.7379 | 0.743 | 0.6395 | 0.6862 | ||
40% | 0.9484 | 0.9361 | 0.9421 | 0.8039 | 0.7286 | 0.7641 | 0.7651 | 0.6792 | 0.7190 | |||
50% | 0.9601 | 0.8864 | 0.9213 | 0.8248 | 0.6981 | 0.7558 | 0.7957 | 0.7144 | 0.7527 |
Attack Level | Attack Strength | Traditional FDIA [8] | LMM-FDIA [18] | AttDiff-FDIA | ||||||||
Precision | Recall | F1-Score | Precision | Recall | F1-Score | Precision | Recall | F1-Score | ||||
Weak | 2% | 0.7187 | 0.7181 | 0.7078 | 0.7199 | 0.7223 | 0.7074 | 0.6934 | 0.6977 | 0.6815 | ||
5% | 0.7664 | 0.7799 | 0.7650 | 0.7200 | 0.7521 | 0.7242 | 0.7042 | 0.7200 | 0.6990 | |||
10% | 0.8594 | 0.8605 | 0.8549 | 0.7404 | 0.7263 | 0.7215 | 0.7208 | 0.7177 | 0.7074 | |||
Medium | 15% | 0.9094 | 0.9250 | 0.9141 | 0.7549 | 0.7109 | 0.7159 | 0.7119 | 0.7214 | 0.7034 | ||
20% | 0.9507 | 0.9437 | 0.9452 | 0.7672 | 0.7272 | 0.7342 | 0.7205 | 0.7132 | 0.7052 | |||
25% | 0.9737 | 0.9609 | 0.9660 | 0.7743 | 0.7122 | 0.7285 | 0.7309 | 0.7130 | 0.7082 | |||
Strong | 30% | 0.9801 | 0.9789 | 0.9787 | 0.7850 | 0.7346 | 0.7485 | 0.7439 | 0.7227 | 0.7211 | ||
40% | 0.9922 | 0.9817 | 0.9864 | 0.7836 | 0.7219 | 0.7385 | 0.7629 | 0.7115 | 0.7247 | |||
50% | 0.9919 | 0.9900 | 0.9906 | 0.8051 | 0.7451 | 0.7639 | 0.7943 | 0.7208 | 0.7456 |
Attack Level | Attack Strength | Traditional FDIA [8] | LMM-FDIA [18] | AttDiff-FDIA | ||||||||
Precision | Recall | F1-Score | Precision | Recall | F1-Score | Precision | Recall | F1-Score | ||||
Weak | 2% | 0.7414 | 0.7723 | 0.7555 | 0.7737 | 0.7234 | 0.7472 | 0.7180 | 0.7508 | 0.7328 | ||
5% | 0.8171 | 0.9069 | 0.8593 | 0.7976 | 0.8309 | 0.8138 | 0.7277 | 0.6972 | 0.7112 | |||
10% | 0.8814 | 0.9246 | 0.9011 | 0.8270 | 0.7857 | 0.8054 | 0.7461 | 0.7098 | 0.7273 | |||
Medium | 15% | 0.9326 | 0.9334 | 0.9325 | 0.8317 | 0.8007 | 0.8157 | 0.7526 | 0.7548 | 0.7533 | ||
20% | 0.9562 | 0.9651 | 0.9605 | 0.8549 | 0.7868 | 0.8194 | 0.7721 | 0.7269 | 0.7485 | |||
25% | 0.9835 | 0.9819 | 0.9826 | 0.8705 | 0.8009 | 0.8340 | 0.7866 | 0.7460 | 0.7656 | |||
Strong | 30% | 0.9867 | 0.9881 | 0.9874 | 0.8828 | 0.8098 | 0.8442 | 0.8135 | 0.7936 | 0.8024 | ||
40% | 0.9993 | 0.9954 | 0.9973 | 0.9039 | 0.7797 | 0.8371 | 0.8417 | 0.7673 | 0.8024 | |||
50% | 1.0000 | 0.9987 | 0.9993 | 0.9234 | 0.7419 | 0.8220 | 0.8860 | 0.7651 | 0.8195 |
Attack Level | Attack Strength | Traditional FDIA [8] | LMM-FDIA [18] | AttDiff-FDIA | ||||||||
Precision | Recall | F1-Score | Precision | Recall | F1-Score | Precision | Recall | F1-Score | ||||
Weak | 2% | 0.7134 | 0.7293 | 0.710 | 0.6996 | 0.7330 | 0.7039 | 0.6880 | 0.7415 | 0.7043 | ||
5% | 0.7462 | 0.7644 | 0.7455 | 0.7174 | 0.7304 | 0.7107 | 0.7035 | 0.7280 | 0.7039 | |||
10% | 0.8235 | 0.8068 | 0.8072 | 0.7407 | 0.7309 | 0.7208 | 0.7106 | 0.7240 | 0.7049 | |||
Medium | 15% | 0.8652 | 0.8707 | 0.8628 | 0.7644 | 0.7457 | 0.7439 | 0.7106 | 0.7357 | 0.7118 | ||
20% | 0.9282 | 0.9162 | 0.9190 | 0.7856 | 0.7318 | 0.7461 | 0.7329 | 0.7134 | 0.7118 | |||
25% | 0.9493 | 0.9322 | 0.9382 | 0.7990 | 0.7221 | 0.7467 | 0.7424 | 0.7187 | 0.7194 | |||
Strong | 30% | 0.9571 | 0.9603 | 0.9571 | 0.8110 | 0.7554 | 0.7733 | 0.7513 | 0.7469 | 0.7381 | ||
40% | 0.9746 | 0.9741 | 0.9734 | 0.8227 | 0.7357 | 0.7691 | 0.7991 | 0.7441 | 0.7601 | |||
50% | 0.9836 | 0.9773 | 0.9797 | 0.8422 | 0.7668 | 0.7925 | 0.8364 | 0.7577 | 0.7861 |
Attack Level | Attack Strength | Traditional FDIA [8] | LMM-FDIA [18] | AttDiff-FDIA | ||||||||
Precision | Recall | F1-Score | Precision | Recall | F1-Score | Precision | Recall | F1-Score | ||||
Weak | 2% | 0.7263 | 0.6764 | 0.6960 | 0.7159 | 0.8198 | 0.7640 | 0.6963 | 0.7457 | 0.7192 | ||
5% | 0.7541 | 0.7241 | 0.7382 | 0.7353 | 0.8149 | 0.7730 | 0.7179 | 0.6841 | 0.6994 | |||
10% | 0.8359 | 0.8269 | 0.8305 | 0.7637 | 0.8015 | 0.7819 | 0.7204 | 0.6691 | 0.6928 | |||
Medium | 15% | 0.8815 | 0.8458 | 0.8630 | 0.7829 | 0.7701 | 0.7761 | 0.7219 | 0.6527 | 0.6853 | ||
20% | 0.9153 | 0.9364 | 0.9255 | 0.7971 | 0.7372 | 0.7657 | 0.7323 | 0.6584 | 0.6920 | |||
25% | 0.9341 | 0.9515 | 0.9427 | 0.8090 | 0.7355 | 0.7704 | 0.7456 | 0.7242 | 0.7327 | |||
Strong | 30% | 0.9538 | 0.9624 | 0.9580 | 0.8270 | 0.7113 | 0.7648 | 0.7608 | 0.7020 | 0.7272 | ||
40% | 0.9776 | 0.9731 | 0.9753 | 0.8409 | 0.7082 | 0.7688 | 0.8002 | 0.7195 | 0.7574 | |||
50% | 0.9873 | 0.9737 | 0.9804 | 0.8630 | 0.7151 | 0.7812 | 0.8269 | 0.7501 | 0.7861 |
Attack Level | Attack Strength | Traditional FDIA [8] | LMM-FDIA [18] | AttDiff-FDIA | ||||||||
Precision | Recall | F1-Score | Precision | Recall | F1-Score | Precision | Recall | F1-Score | ||||
Weak | 2% | 0.6883 | 0.6992 | 0.6819 | 0.6740 | 0.6958 | 0.6730 | 0.6469 | 0.7548 | 0.6892 | ||
5% | 0.7289 | 0.7233 | 0.7130 | 0.6831 | 0.7482 | 0.7045 | 0.6664 | 0.7173 | 0.6755 | |||
10% | 0.7601 | 0.7829 | 0.7602 | 0.7079 | 0.6778 | 0.6768 | 0.6937 | 0.7017 | 0.6860 | |||
Medium | 15% | 0.8205 | 0.8101 | 0.8062 | 0.7351 | 0.6716 | 0.6867 | 0.7081 | 0.6757 | 0.6777 | ||
20% | 0.8469 | 0.8647 | 0.8483 | 0.7514 | 0.7095 | 0.7146 | 0.7129 | 0.6529 | 0.6661 | |||
25% | 0.8772 | 0.8817 | 0.8731 | 0.7656 | 0.6992 | 0.7159 | 0.7209 | 0.6941 | 0.6958 | |||
Strong | 30% | 0.9092 | 0.9111 | 0.9050 | 0.7857 | 0.7062 | 0.7314 | 0.7324 | 0.7014 | 0.7037 | ||
40% | 0.9223 | 0.9423 | 0.9289 | 0.7995 | 0.7286 | 0.7503 | 0.7561 | 0.7280 | 0.7297 | |||
50% | 0.9313 | 0.9490 | 0.9371 | 0.8117 | 0.7547 | 0.7702 | 0.7789 | 0.7156 | 0.7339 |
Attack Level | Attac Strength | Traditional FDIA [8] | LMM-FDIA [18] | AttDiff-FDIA | ||||||||
Precision | Recall | F1-Score | Precision | Recall | F1-Score | Precision | Recall | F1-Score | ||||
Weak | 2% | 0.7038 | 0.7208 | 0.7117 | 0.6859 | 0.7404 | 0.7119 | 0.6669 | 0.7540 | 0.7064 | ||
5% | 0.7167 | 0.7437 | 0.7298 | 0.6927 | 0.7707 | 0.7293 | 0.6828 | 0.7456 | 0.7127 | |||
10% | 0.7671 | 0.7915 | 0.7602 | 0.7235 | 0.7583 | 0.7404 | 0.6972 | 0.7551 | 0.7248 | |||
Medium | 15% | 0.7958 | 0.8235 | 0.8062 | 0.7431 | 0.7351 | 0.7383 | 0.7203 | 0.6961 | 0.7060 | ||
20% | 0.8277 | 0.8976 | 0.8610 | 0.7547 | 0.6959 | 0.7240 | 0.7220 | 0.6695 | 0.6935 | |||
25% | 0.8679 | 0.9059 | 0.8864 | 0.7565 | 0.7253 | 0.7402 | 0.7334 | 0.6325 | 0.6787 | |||
Strong | 30% | 0.9075 | 0.9317 | 0.9194 | 0.7715 | 0.7086 | 0.7379 | 0.743 | 0.6395 | 0.6862 | ||
40% | 0.9484 | 0.9361 | 0.9421 | 0.8039 | 0.7286 | 0.7641 | 0.7651 | 0.6792 | 0.7190 | |||
50% | 0.9601 | 0.8864 | 0.9213 | 0.8248 | 0.6981 | 0.7558 | 0.7957 | 0.7144 | 0.7527 |
Attack Level | Attack Strength | Traditional FDIA [8] | LMM-FDIA [18] | AttDiff-FDIA | ||||||||
Precision | Recall | F1-Score | Precision | Recall | F1-Score | Precision | Recall | F1-Score | ||||
Weak | 2% | 0.7187 | 0.7181 | 0.7078 | 0.7199 | 0.7223 | 0.7074 | 0.6934 | 0.6977 | 0.6815 | ||
5% | 0.7664 | 0.7799 | 0.7650 | 0.7200 | 0.7521 | 0.7242 | 0.7042 | 0.7200 | 0.6990 | |||
10% | 0.8594 | 0.8605 | 0.8549 | 0.7404 | 0.7263 | 0.7215 | 0.7208 | 0.7177 | 0.7074 | |||
Medium | 15% | 0.9094 | 0.9250 | 0.9141 | 0.7549 | 0.7109 | 0.7159 | 0.7119 | 0.7214 | 0.7034 | ||
20% | 0.9507 | 0.9437 | 0.9452 | 0.7672 | 0.7272 | 0.7342 | 0.7205 | 0.7132 | 0.7052 | |||
25% | 0.9737 | 0.9609 | 0.9660 | 0.7743 | 0.7122 | 0.7285 | 0.7309 | 0.7130 | 0.7082 | |||
Strong | 30% | 0.9801 | 0.9789 | 0.9787 | 0.7850 | 0.7346 | 0.7485 | 0.7439 | 0.7227 | 0.7211 | ||
40% | 0.9922 | 0.9817 | 0.9864 | 0.7836 | 0.7219 | 0.7385 | 0.7629 | 0.7115 | 0.7247 | |||
50% | 0.9919 | 0.9900 | 0.9906 | 0.8051 | 0.7451 | 0.7639 | 0.7943 | 0.7208 | 0.7456 |
Attack Level | Attack Strength | Traditional FDIA [8] | LMM-FDIA [18] | AttDiff-FDIA | ||||||||
Precision | Recall | F1-Score | Precision | Recall | F1-Score | Precision | Recall | F1-Score | ||||
Weak | 2% | 0.7414 | 0.7723 | 0.7555 | 0.7737 | 0.7234 | 0.7472 | 0.7180 | 0.7508 | 0.7328 | ||
5% | 0.8171 | 0.9069 | 0.8593 | 0.7976 | 0.8309 | 0.8138 | 0.7277 | 0.6972 | 0.7112 | |||
10% | 0.8814 | 0.9246 | 0.9011 | 0.8270 | 0.7857 | 0.8054 | 0.7461 | 0.7098 | 0.7273 | |||
Medium | 15% | 0.9326 | 0.9334 | 0.9325 | 0.8317 | 0.8007 | 0.8157 | 0.7526 | 0.7548 | 0.7533 | ||
20% | 0.9562 | 0.9651 | 0.9605 | 0.8549 | 0.7868 | 0.8194 | 0.7721 | 0.7269 | 0.7485 | |||
25% | 0.9835 | 0.9819 | 0.9826 | 0.8705 | 0.8009 | 0.8340 | 0.7866 | 0.7460 | 0.7656 | |||
Strong | 30% | 0.9867 | 0.9881 | 0.9874 | 0.8828 | 0.8098 | 0.8442 | 0.8135 | 0.7936 | 0.8024 | ||
40% | 0.9993 | 0.9954 | 0.9973 | 0.9039 | 0.7797 | 0.8371 | 0.8417 | 0.7673 | 0.8024 | |||
50% | 1.0000 | 0.9987 | 0.9993 | 0.9234 | 0.7419 | 0.8220 | 0.8860 | 0.7651 | 0.8195 |
Attack Level | Attack Strength | Traditional FDIA [8] | LMM-FDIA [18] | AttDiff-FDIA | ||||||||
Precision | Recall | F1-Score | Precision | Recall | F1-Score | Precision | Recall | F1-Score | ||||
Weak | 2% | 0.7134 | 0.7293 | 0.710 | 0.6996 | 0.7330 | 0.7039 | 0.6880 | 0.7415 | 0.7043 | ||
5% | 0.7462 | 0.7644 | 0.7455 | 0.7174 | 0.7304 | 0.7107 | 0.7035 | 0.7280 | 0.7039 | |||
10% | 0.8235 | 0.8068 | 0.8072 | 0.7407 | 0.7309 | 0.7208 | 0.7106 | 0.7240 | 0.7049 | |||
Medium | 15% | 0.8652 | 0.8707 | 0.8628 | 0.7644 | 0.7457 | 0.7439 | 0.7106 | 0.7357 | 0.7118 | ||
20% | 0.9282 | 0.9162 | 0.9190 | 0.7856 | 0.7318 | 0.7461 | 0.7329 | 0.7134 | 0.7118 | |||
25% | 0.9493 | 0.9322 | 0.9382 | 0.7990 | 0.7221 | 0.7467 | 0.7424 | 0.7187 | 0.7194 | |||
Strong | 30% | 0.9571 | 0.9603 | 0.9571 | 0.8110 | 0.7554 | 0.7733 | 0.7513 | 0.7469 | 0.7381 | ||
40% | 0.9746 | 0.9741 | 0.9734 | 0.8227 | 0.7357 | 0.7691 | 0.7991 | 0.7441 | 0.7601 | |||
50% | 0.9836 | 0.9773 | 0.9797 | 0.8422 | 0.7668 | 0.7925 | 0.8364 | 0.7577 | 0.7861 |
Attack Level | Attack Strength | Traditional FDIA [8] | LMM-FDIA [18] | AttDiff-FDIA | ||||||||
Precision | Recall | F1-Score | Precision | Recall | F1-Score | Precision | Recall | F1-Score | ||||
Weak | 2% | 0.7263 | 0.6764 | 0.6960 | 0.7159 | 0.8198 | 0.7640 | 0.6963 | 0.7457 | 0.7192 | ||
5% | 0.7541 | 0.7241 | 0.7382 | 0.7353 | 0.8149 | 0.7730 | 0.7179 | 0.6841 | 0.6994 | |||
10% | 0.8359 | 0.8269 | 0.8305 | 0.7637 | 0.8015 | 0.7819 | 0.7204 | 0.6691 | 0.6928 | |||
Medium | 15% | 0.8815 | 0.8458 | 0.8630 | 0.7829 | 0.7701 | 0.7761 | 0.7219 | 0.6527 | 0.6853 | ||
20% | 0.9153 | 0.9364 | 0.9255 | 0.7971 | 0.7372 | 0.7657 | 0.7323 | 0.6584 | 0.6920 | |||
25% | 0.9341 | 0.9515 | 0.9427 | 0.8090 | 0.7355 | 0.7704 | 0.7456 | 0.7242 | 0.7327 | |||
Strong | 30% | 0.9538 | 0.9624 | 0.9580 | 0.8270 | 0.7113 | 0.7648 | 0.7608 | 0.7020 | 0.7272 | ||
40% | 0.9776 | 0.9731 | 0.9753 | 0.8409 | 0.7082 | 0.7688 | 0.8002 | 0.7195 | 0.7574 | |||
50% | 0.9873 | 0.9737 | 0.9804 | 0.8630 | 0.7151 | 0.7812 | 0.8269 | 0.7501 | 0.7861 |