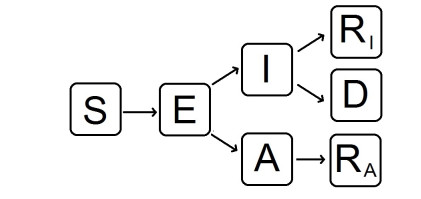
Citation: Ugo Avila-Ponce de León, Ángel G. C. Pérez, Eric Avila-Vales. A data driven analysis and forecast of an SEIARD epidemic model for COVID-19 in Mexico[J]. Big Data and Information Analytics, 2020, 5(1): 14-28. doi: 10.3934/bdia.2020002
[1] | Antonio N. Bojanic, Alejandro Jordán . Modeling the COVID-19 epidemic in Bolivia. Big Data and Information Analytics, 2020, 5(1): 47-57. doi: 10.3934/bdia.2020004 |
[2] | Shuqi Wang, Wen Tang, Liyan Xiong, Mengyu Fang, Bingsong Zhang, Chi-Yang Chiu, Ruzong Fan . Mathematical modeling of transmission dynamics of COVID-19. Big Data and Information Analytics, 2021, 6(0): 12-25. doi: 10.3934/bdia.2021002 |
[3] | Antonio N. Bojanic . Accounting for the Trump factor in modeling the COVID-19 epidemic: the case of Louisiana. Big Data and Information Analytics, 2021, 6(0): 74-85. doi: 10.3934/bdia.2021006 |
[4] | Nickson Golooba, Woldegebriel Assefa Woldegerima, Huaiping Zhu . Deep neural networks with application in predicting the spread of avian influenza through disease-informed neural networks. Big Data and Information Analytics, 2025, 9(0): 1-28. doi: 10.3934/bdia.2025001 |
[5] | Ricky Fok, Agnieszka Lasek, Jiye Li, Aijun An . Modeling daily guest count prediction. Big Data and Information Analytics, 2016, 1(4): 299-308. doi: 10.3934/bdia.2016012 |
[6] | Zhouchen Lin . A Review on Low-Rank Models in Data Analysis. Big Data and Information Analytics, 2016, 1(2): 139-161. doi: 10.3934/bdia.2016001 |
[7] | Minlong Lin, Ke Tang . Selective further learning of hybrid ensemble for class imbalanced increment learning. Big Data and Information Analytics, 2017, 2(1): 1-21. doi: 10.3934/bdia.2017005 |
[8] | Marco Tosato, Jianhong Wu . An application of PART to the Football Manager data for players clusters analyses to inform club team formation. Big Data and Information Analytics, 2018, 3(1): 43-54. doi: 10.3934/bdia.2018002 |
[9] | Jason Adams, Yumou Qiu, Luis Posadas, Kent Eskridge, George Graef . Phenotypic trait extraction of soybean plants using deep convolutional neural networks with transfer learning. Big Data and Information Analytics, 2021, 6(0): 26-40. doi: 10.3934/bdia.2021003 |
[10] | Wenxue Huang, Yuanyi Pan . On Balancing between Optimal and Proportional categorical predictions. Big Data and Information Analytics, 2016, 1(1): 129-137. doi: 10.3934/bdia.2016.1.129 |
The COVID-19 pandemic originated in Wuhan, China in December 2019. Since then, the number of cases has accelerated in China and subsequently all over the world. The causative agent is a new betacoronavirus related to the Middle East Respiratory Syndrome virus (MERS-CoV) and the Severe Acute Respiratory Syndrome virus (SARS-CoV).
On January 30, 2020, the World Health Organization (WHO) formally declared the outbreak of novel coronavirus a Global Public Health Emergency of International Concern. In [1], Cruz-Pacheco et al. estimated the arrival of the infectious outbreak to Mexico between March 20 and March 30, 2020. Other models for predicting the evolution of COVID-19 outbreak in Mexico have been proposed in [2,3,4,5].
Compartmental models have been used for studying the spread of the COVID-19 pandemic in several countries, such as China [6,7,8,9], Italy [8,9], India [10] and Brazil [11]. Since it is known that individuals infected with SARS-CoV-2 can spread the virus to other people even when they do not present any symptoms of the disease, many authors have developed models that consider compartments for both the symptomatic and asymptomatic infectious individuals, see, e.g., [5,12,13,14,15,16]. Moreover, the rate at which the virus is transmitted to the susceptible population by contact with an infected individual is different for the symptomatic and asymptomatic, due to the varying infectivity at different stages of the infectious period and the fact that persons with COVID-19 symptoms take preventive measures like staying in quarantine, which reduces the effective number of contacts. Hence, it is more realistic to consider different transmission rates for the population in the symptomatic and asymptomatic compartments.
In this paper, we propose a novel SEIARD compartmental model to investigate the current outbreak of coronavirus disease in Mexico. Our model includes compartments for the exposed subpopulation and for the symptomatic and asymptomatic individuals. We conduct a detailed mathematical analysis of this model and demonstrate its application using the publicly reported data for the outbreak.
We obtained the daily cumulative number of new infected cases for 2019-nCoV epidemics in Mexico provided by the Ministry of Health of said country, but we downloaded the data of the outbreak directly from the repository of Johns Hopkins University [17], which is updated daily at 7 pm. The data were collected in the period since the first case of COVID-19 in Mexico (February 28, 2020) to August 12, 2020.
There are many mathematical models used to explain the spread of infectious diseases. In our case, we will use a compartmental differential equation model for the spread of COVID-19 in Mexico [18,19]. Our model monitors the dynamics of six subpopulations, which are: susceptible (S(t)), exposed (E(t)), infected (I(t)), asymptomatic (A(t)), recovered (RI(t) and RA(t)) and dead (D(t)).
The model simulations were carried out with the following assumptions:
(a) The susceptible and infected individuals are homogeneous in the population.
(b) At first, no interventions were applied to stop the spread of COVID-19.
(c) No births are allowed in the population, and we only take into account the fatalities associated to COVID-19.
A diagram of the flow through the compartmental subpopulations can be seen in Figure 1.
This number is of great importance in epidemiological modeling, because it represents the number of secondary infections derived from a single infected individual in a fully susceptible population. In other words, it determines the potential of the spread of a virus without any control measures. The basic reproduction number was obtained from the reported cases (infected, recovered and deaths) from the period of having roughly 12 infected individuals (March 12) until August 12, 2020. The estimation of the basic reproductive number from the SEIARD model was calculated using the next-generation matrix method [20].
We calibrated our mathematical model with the daily cumulative data for new infections, recovered individuals and deaths from COVID-19 in Mexico, obtained from the open source repository of Johns Hopkins University [17]. Even though the outbreak of the disease started in December 2019, the first reported case in Mexico was until February 28, 2020 (an imported case). We considered the data for the outbreak of COVID-19 in Mexico up to August 12, 2020.
The set of differential equations was solved using Matlab 2016b with the ode45 solver, which is based on an explicit Runge-Kutta (4, 5) formula.
The optimization of parameters to describe the outbreak of COVID-19 in Mexico was fitted in two steps: The first step was minimizing the Sum of Squared Errors (SSE), which is calculated as follows. For a given vector of parameters x, we compute the numerical solutions for our model (S(t),E(t),I(t),A(t),RI(t),RA(t),D(t)) and the cumulative number of infected cases with symptoms C(t):=I(t)+RI(t)+D(t). Then the Sum of Squared Errors is given by
SSE(x)=n∑i=1[k1(C(ti)−Cexpi)2+k2(D(ti)−Dexpi)2+k3(RI(ti)−Rexpi)2], | (2.1) |
where Cexpi, Dexpi and Rexpi denote the experimental data for cumulative infections, deaths and recoveries, respectively, reported for day ti (i=1,…,n), while k1, k2 and k3 are coefficients used to compensate the order of magnitude for the data. In our simulations, we used k1=20, k2=10 and k3=1. We considered fixed values for the parameters w and p, assuming a latent period of 4 days [21] and that the total number of infectious cases (symptomatic and asymptomatic) is around eight times larger than the reported cases [22]. The other parameter values were fitted by applying three searches to minimize the SSE function: A gradient-based method, a gradient-free algorithm, and finally, a gradient-based method. This method was necessary to obtain the global minimum. For this step, we adapted the code from [9] for our mathematical model.
For the second step, we used the set of parameters obtained by minimizing the SSE as the initial value of a Markov Chain Monte Carlo (MCMC) approach, where we set the iteration number to 8000 and the first 6000 iterations as burn-in periods. Then, we computed the solutions of the model for each set of parameters obtained after the burn-in period and computed the mean and standard deviation for these solutions. Finally, we used these results to plot the 95% confidence intervals for the time evolution of the solutions. The code is available at https://github.com/UgoAvila/COVID-19.Mexico.
Susceptible population S(t): This subpopulation cannot increase, because we are not considering the natural recruitment or deaths that are not related to COVID-19. The susceptible population will only decrease after an infection, an acquired characteristic due to the interaction with an infected person or asymptomatic one. The transmission coefficients will be βII (for contact with symptomatic infectious individuals) and βAA (for contact with asymptomatic infectious individuals). The rate of change of the susceptible population is expressed in the following equation:
dSdt=−S(βII+βAAN−D). | (3.1) |
Exposed population E(t): This subpopulation consists of individuals that are infected but cannot infect others. The population decreases at a rate w to become infectious with or without symptoms. We remark that 1/w represents the average length of the latent period, which is the period between the infection with the virus and the moment when the host is able to transmit the pathogen to susceptible individuals. An exposed individual becomes symptomatically infectious with a probability p or asymptomatically infectious with a probability 1−p. Consequently,
dEdt=S(βII+βAAN−D)−wE=S(βII+βAAN−D)−pwE−(1−p)wE. | (3.2) |
Infected population I(t): Infected (symptomatic) individuals are generated at a proportion p from the exposed class. They recover at a rate γ and die at a rate δ. This is the only population that acknowledges death. Thus,
dIdt=pwE−(δ+γ)I. | (3.3) |
Asymptomatic population A(t): This population is considered an infected population, but the individuals do not develop the common symptoms of COVID-19. Asymptomatic individuals are important to model because they have the ability to spread the virus without knowing; they are produced at a rate 1−p and recover at a rate γ. Consequently,
dAdt=(1−p)wE−γA. | (3.4) |
Recovered populations RI(t) and RA(t): All individuals infected with symptoms or not will recover at a rate γ. We subdivide the recovered population in two compartments: individuals who recover after having symptoms (RI) and individuals who recover from asymptomatic infection (RA). Hence
dRIdt=γI,dRAdt=γA. | (3.5) |
Dead population D(t): Infected individuals with symptoms die at a rate δ, that is,
dDdt=δI. | (3.6) |
Hence, the system of differential equations that will model the dynamics of coronavirus spread in Mexico is:
dSdt=−S(βII+βAAN−D),dEdt=S(βII+βAAN−D)−wE,dIdt=pwE−(δ+γ)I,dAdt=(1−p)wE−γA,dRIdt=γI,dRAdt=γA,dDdt=δI. | (3.7) |
We also observe that N:=S+E+I+A+RI+RA+D is constant, where N is the size of the population modeled.
System (3.7) has a disease-free equilibrium, which is given by S=N, E=I=A=RI=RA=D=0, and we will denote it by x0. We calculate the basic reproduction number R0 based on this steady state. We use the next-generation matrix method proposed by [20]. To find R0, we must solve the equation R0=ρ(FV−1), where F and V are the derivatives of the new infections matrix F and the transition matrix V, respectively, evaluated at the disease-free equilibrium. Then
F=[S(βII+βAAN−D)00] |
The derivative of F at x0 is:
F=[0βIβA000000]. |
The transition matrix is
V=[wE−pwE+(δ+γ)I−(1−p)wE+γA]. |
The derivative of V at x0 is
V=[w00−pwδ+γ0−(1−p)w0γ]. |
The inverse of V is
V−1=[1w00pδ+γ1δ+γ01−pγ01γ]. |
Then
FV−1=[βIpδ+γ+βA(1−p)γβIδ+γβAγ000000]. |
We need to find the eigenvalues of FV−1, which are λ1=βIpδ+γ+βA(1−p)γ, λ2=0 and λ3=0. Then, the basic reproduction number is given by the dominant eigenvalue, that is,
R0=βIpδ+γ+βA(1−p)γ. | (3.8) |
To describe the evolution of the epidemic in Mexico taking into account the social distancing measures taken by the government, we will assume that the infection rate, recovery rate and death rate are time-dependent functions, similar to those used by [9].
To model the effect of epidemic control measures, which cause the number of contacts per person per unit time to decrease as the epidemic progresses, we describe the infection rates by the functions
βI(t)=β0Iexp(−tτβ)+β1I,βA(t)=β0Aexp(−tτβ)+β1A, |
where β0I+β1I is the initial infection rate by contact with the symptomatically infectious (respectively, β0A+β1A, by contact with the asymptomatically infectious); this rate decreases exponentially to the value β1I (respectively, β1A) with a characteristic time of decrease τβ.
The time of recovery for patients may also vary with time due to the medical staff improving their therapeutic procedures. Hence, we will assume that the recovery rate is modeled by the function
γ(t)=γ0+γ11+exp(−t+τγ), |
where γ0 is the recovery rate at time zero, and γ0+γ1 is the recovery rate at a later time, which is reached after τγ days of adaptation.
Lastly, the death rate may decrease with time due to the adaptation of the pathogen or the development of more advanced treatments. Hence, we can model this with the function
δ(t)=δ0exp(−tτδ)+δ1, |
where δ0+δ1 is the initial death rate, which decreases to the value δ1 with a characteristic time τδ.
If we replace the constant parameters βI, βA, δ and γ in Eq (3.8) with the aforementioned time-dependent functions, we can define
Rd(t)=βI(t)pδ(t)+γ(t)+βA(t)(1−p)γ(t) | (3.9) |
as the effective daily reproduction ratio, which measures the number of new infections produced by a single infected individual per day, taking into account the evolving public health interventions and available resources [23].
The parameters of the model were fitted with the experimental data provided by a daily update from the University of Johns Hopkins, which is consistent with the data handed over daily by the Mexican Ministry of Health. Adjusting the data from the period from March 12, 2020 to August 12, 2020, we simulated the daily new COVID-19 cases in Mexico until December 2020. In Figure 2 we simulated the predicted evolution of the outbreak of COVID-19 in Mexico. As we can see in Figure 2, the red dotted line represents the active cases of this disease: Mexico has succeeded in flattening the curve. The peak of the disease will be roughly between October and November of 2020. The asymptomatic infected individuals (blue dashed line) are a major concern in the Mexican population because this subpopulation presents an exponential behavior with respect to the active cases.
The values of the best fit parameters are given in Table 1. After performing the MCMC simulations, we obtained the 95% confidence intervals for the estimated parameters. Figure 3 shows the variation of the infection rate by contact with symptomatic individuals (βI(t)) and the infection rate by contact with asymptomatic individuals (βA(t)). Let us evaluate first the force of infection of the symptomatic subpopulation (Figure 3a), which varies over time. The first 50 days from March 12 were the most important, starting with a value around 0.6, due to the "Jornada de Sana Distancia'' it descended to a value of 0.2. Since then this rate has stayed at a value close to 0.1. Roughly speaking, the symptomatic individuals may not be the responsible for spreading the disease due to the fact that they are quarantined with mild symptomatology or hospitalized. The force of infection of the asymptomatic individuals (Figure 3b) behaves practically the same than the symptomatic subpopulation starting around 0.8. In the first 50 days, it descended to a value of 0.3 and by then it has continued to a value near 0.2. This being said, an asymptomatic individual has the capacity to infect more individuals than symptomatic individuals. It is important to mention that the transmission rate decreased at a characteristic time of τβ≈31.91, which demonstrates the emphasis of the lockdown. In Mexico, it took roughly 50 to 60 days to substantially reduce the spread of the virus.
Parameter | Best fit value for Mexico | 95% CI | Unit |
β0I | 0.5534 | (0.4779, 0.6290) | 1/day |
β1I | 0.1069 | (0.0800, 0.1339) | 1/day |
τβ | 31.9108 | (27.8195, 36.0022) | day |
β0A | 0.6855 | (0.6326, 0.7385) | 1/day |
β1A | 0.1465 | (0.1185, 0.1746) | 1/day |
δ0 | 0.0561 | (0.0439, 0.0683) | 1/day |
δ1 | 0.0211 | (0.0171, 0.0251) | 1/day |
τδ | 13.9249 | (0, 29.7695) | day |
γ0 | 0.0708 | (0.0556, 0.0860) | 1/day |
γ1 | 0.0675 | (0.0577, 0.0774) | 1/day |
τγ | 17.4348 | (11.2411, 23.6284) | day |
w | 0.25 | (fixed) | 1/day |
p | 0.12 | (fixed) | – |
Figure 4 shows the variation of the recovery rate γ(t) and death rate δ(t) with respect to time. The death rate is represented in Figure 4a. At the beginning of the pandemic, the death rate in Mexico was very high with a value between 0.08 and 0.12. The average time of death was roughly 10 days since the onset of symptoms. As time passed, the increase in the average time of death was for two main reasons: The addition of less severe cases and an improvement in the treatments used in the patients. Eventually, the death rate will stabilize at a long term lethality betweem 0.02 and 0.03. The recovery rate is depicted in Figure 4b: The first 10 to 15 days were the most difficult in Mexico showing a recovery rate lower than 0.1. This dynamic could be associated with a much higher death rate. At 40 days past March 12, the recovery of patients was higher compared to the beginning of the pandemic in Mexico.
Using these values for the parameters, we can calculate the effective daily reproductive ratio Rd(t) for each day (Figure 5). At the beginning of March, Rd(t) was very high. After the month of March passed and the "Jornada de Sana Distancia'' was implemented, the value descended rapidly 60% percent with respect to the initial days of the pandemic in Mexico. By June, 2020, the value was near 1, and it will continue very close to that value as time passes.
In Figures 6–10, we carried out the simulation with the best fit parameters. We show a comparison of the cumulative number of infections in Figure 6). The death toll (Figure 7) in Mexico caused by COVID-19 may be around 250 000 if the outbreak of the disease maintains its course modeled by our differential equations, with a lower bound of 140 000 given by the 95% confidence interval. In Figure 8 we plotted the number of active symptomatic infections, in this graph we can acknowledge the peak of the disease will be approximately between August and Novermber 2020. At the moment we are writing this article in mid-August, Mexico is in the plateau of the exponential growth of the number of active cases. Asymptomatic individuals maintain the same behavior than the active cases (Figure 9). Finally, the recovered subpopulation until December 2020 will be between 800 000 and 2 million people (Figure 10).
In our simulations, we assumed that the population of asymptomatic individuals represents roughly 88% of all possible infections. The behavior of the asymptomatic population is important because they have the capacity to spread the virus without developing any symptoms, and public health interventions should focus on these individuals. By declaring self-quarantine to all individuals, we could separate the healthy population from the asymptomatic population and prevent the spread of the virus more rapidly. This action will only gain time in the number of infections and help avoiding the saturation of hospitals. If we control the population of infected individuals, we will prevent the death of many individuals, and the hospitals will have sufficient supplies to mitigate the severity of symptoms in patients with any type of chronic degenerative disease.
This work makes a forecast of the spread of COVID-19 in Mexico starting on August 12, 2020. The epidemic of COVID-19 in Mexico is far from over, we estimate that the force of infection has already passed and it is on the plateau of the exponential growth of the disease.
The Mexican government went ahead in establishing quarantine for all the population, a preventive measure specific to the Phase 2 of an epidemic. This measure may explain why in Mexico the number of infected individuals is different than in other countries [6,7,8,9]. The asymptomatic individuals are of great concern in Mexico and the world. In our case, their force of infection is greater than for symptomatic individuals because the initial value of the infection kinetics is β0A+β1A=0.83 compared with β0I+β1I=0.66 for the best fit parameters. This being said, Mexico should focus much more their actions on discovering rapidly this subpopulation, because they are spreading the virus without knowing and this is why the virus has been a success in infecting the entire world, but by advancing the quarantine theoretically, this subpopulation is isolated, ergo reducing considerably its kinetic infection at infinite time.
On June 1, Mexico ended the "Jornada de Sana Distancia'' and developed a system very similar to a traffic light system. This new normality was developed for the reactivation of the Mexican economy. It was determined based on 4 colors (red, orange, yellow and green), where red is the highest risk level, while green is the lowest. Due to the heterogeneity of the outbreak in Mexico, the first half of June the entire country remained at the highest risk level, which meant 15 days more of isolation.
For the 90% of those infected with COVID-19 to decrease, Mexico must have been quarantined for 93 days. Instead, they only stayed for 86 days, which resulted in the orderly reopening of quarantined individuals when the infected population had only decreased by 83%, which is a reasonable value for the reopening of non-essential economies in Mexico. Our mathematical model can be useful for real-time preparedness, anticipating the number of hospital beds and artificial respirators that will be needed once we reach the peak of infections or once Mexico enters Phase 3. The number of beds could be obtained from the number of infected symptomatic individuals, because this population can die from different illnesses caused by 2019-nCoV. Future directions of this work would be extending the SEIARD mathematical model, where we could incorporate how the isolation of infected symptomatic individuals and the quarantine of asymptomatic individuals are sufficient measures to flatten the curve, as Mexico did prior to entering Phase 2.
Ugo Avila Ponce de León is a doctoral student from Programa de Doctorado en Ciencias Biológicas of the Universidad Nacional Autónoma de México (UNAM). This paper was developed in the period of his PhD studies. Ugo Avila Ponce de León also received a fellowship (CVU: 774988) from Consejo Nacional de Ciencia y Tecnología (CONACYT).
This article was supported in part by Mexican SNI under grant number 15284.
All authors declare no conflict of interest in this paper.
[1] | Cruz-Pacheco G, Bustamante-Castañeda JF, Caputo JG, et al. (2020) Dispersion of a new coronavirus SARS-CoV-2 by airlines in 2020: Temporal estimates of the outbreak in Mexico. Rev Invest Clin 72: 138-143. |
[2] | Vivanco-Lira A, (2020) Predicting COVID-19 distribution in Mexico through a discrete and timedependent Markov chain and an SIR-like model. arXiv 2003.06758. |
[3] | Alvarez MM, Gonzalez-Gonzalez E and Trujillo-de Santiago G, (2020) Modeling COVID-19 epidemics in an Excel spreadsheet: Democratizing the access to first-hand accurate predictions of epidemic outbreaks. medRxiv. |
[4] | Acuña-Zegarra MA, Comas-García A, Hernández-Vargas E, et al. (2020) The SARS-CoV-2 epidemic outbreak: A review of plausible scenarios of containment and mitigation for Mexico. medRxiv. |
[5] | Acuña-Zegarra MA, Santana-Cibrian M and Velasco-Hernandez JX, (2020) Modeling behavioral change and COVID-19 containment in Mexico: A trade-off between lockdown and compliance. Math Biosci 325: 108370. |
[6] | Lin Q, Zhao S, Gao D, et al. (2020) A conceptual model for the coronavirus disease 2019 (COVID-19) outbreak in Wuhan, China with individual reaction and governmental action. Int J Infect Dis 93: 211-216. |
[7] | Khrapov P and Loginova A, (2020) Mathematical modelling of the dynamics of the Coronavirus COVID-19 epidemic development in China. Int J Open Inf Technol 8: 13-16. |
[8] | Fanelli D and Piazza F, (2020) Analysis and forecast of COVID-19 spreading in China, Italy and France. Chaos Solitons Fractals 134: 109761. |
[9] | Caccavo D, (2020) Chinese and Italian COVID-19 outbreaks can be correctly described by a modified SIRD model. medRxiv. |
[10] | Singh R and Adhikari R, (2020) Age-structured impact of social distancing on the COVID-19 epidemic in India. arXiv 2003.12055. |
[11] | Bastos SB and Cajueiro DO, (2020) Modeling and forecasting the Covid-19 pandemic in Brazil. arXiv 2003.14288. |
[12] | Sharma S, Volpert V and Banerjee M, (2020) Extended SEIQR type model for COVID-19 epidemic and data analysis. medRxiv. |
[13] | Khoshnaw SHA, Shahzad M, Ali M, et al. (2020) A quantitative and qualitative analysis of the COVID-19 pandemic model. Chaos Solitons Fractals 138: 109932. |
[14] | Monteiro LHA, (2020) An epidemiological model for SARS-CoV-2. Ecol Complex 43: 100836. |
[15] | Nabi KN, (2020) Forecasting COVID-19 pandemic: A data-driven analysis. Chaos Solitons Fractals 139: 110046. |
[16] | Okuonghae D and Omame A, (2020) Analysis of a mathematical model for COVID-19 population dynamics in Lagos, Nigeria. Chaos Solitons Fractals 139: 110032. |
[17] | Johns Hopkins CSSE, 2019 Novel Coronavirus COVID-19 (2019-nCoV) Data Repository, 2020. Available from: https://github.com/CSSEGISandData/COVID-19. |
[18] | Hethcote HW, (2000) The mathematics of infectious diseases. SIAM Rev 42: 599-653. |
[19] | Diekmann O and Heesterbeek JAP, (2000) Mathematical Epidemiology of Infectious Diseases: Model Building, Analysis and Interpretation, New York: John Wiley & Sons. |
[20] | Diekmann O, Heesterbeek JAP and Roberts MG, (2010) The construction of next-generation matrices for compartmental epidemic models. J Royal Soc Interface 7: 873-885. |
[21] | Sjödin H, Wilder-Smith A, Osman S, et al. (2020) Only strict quarantine measures can curb the coronavirus disease (COVID-19) in Italy, 2020. Eurosurveillance 25: 2000280. |
[22] | Expansión política, La magnitud de la epidemia de COVID-19 no se puede medir en tiempo real, 2020. Available from: https://politica.expansion.mx/mexico. |
[23] | Tang B, Bragazzi NL, Li Q, et al. (2020) An updated estimation of the risk of transmission of the novel coronavirus (2019-nCov). Infect Dis Model 5: 248-255. |
1. | Bijay Kumar Sahoo, Balvinder Kaur Sapra, A data driven epidemic model to analyse the lockdown effect and predict the course of COVID-19 progress in India, 2020, 139, 09600779, 110034, 10.1016/j.chaos.2020.110034 | |
2. | Faihatuz Zuhairoh, Dedi Rosadi, Real-time prediction for multi-wave COVID-19 outbreaks, 2022, 29, 2287-7843, 499, 10.29220/CSAM.2022.29.5.499 | |
3. | Fernando Javier Aguilar-Canto, Ugo Avila-Ponce de León, Eric Avila-Vales, Sensitivity theorems of a model of multiple imperfect vaccines for COVID-19, 2022, 156, 09600779, 111844, 10.1016/j.chaos.2022.111844 | |
4. | Souvik Manik, Sabyasachi Pal, Manoj Mandal, Mangal Hazra, Effect of 2021 assembly election in India on COVID-19 transmission, 2022, 107, 0924-090X, 1343, 10.1007/s11071-021-07041-7 | |
5. | Omar Faruk, Suman Kar, A Data Driven Analysis and Forecast of COVID-19 Dynamics during the Third Wave Using SIRD Model in Bangladesh, 2021, 1, 2673-8112, 503, 10.3390/covid1020043 | |
6. | Faihatuz Zuhairoh, Dedi Rosadi, Data-driven analysis and prediction of COVID-19 infection in Southeast Asia by using a phenomenological model, 2022, 2220-5810, 59, 10.18187/pjsor.v18i1.3714 | |
7. | Ugo Avila-Ponce de León, Angel G. C. Pérez, Eric Avila-Vales, Modeling the SARS-CoV-2 Omicron variant dynamics in the United States with booster dose vaccination and waning immunity, 2023, 20, 1551-0018, 10909, 10.3934/mbe.2023484 | |
8. | Jean-David Morel, Jean-Michel Morel, Luis Alvarez, Virginia E. Pitzer, Learning from the past: A short term forecast method for the COVID-19 incidence curve, 2023, 19, 1553-7358, e1010790, 10.1371/journal.pcbi.1010790 |
Parameter | Best fit value for Mexico | 95% CI | Unit |
β0I | 0.5534 | (0.4779, 0.6290) | 1/day |
β1I | 0.1069 | (0.0800, 0.1339) | 1/day |
τβ | 31.9108 | (27.8195, 36.0022) | day |
β0A | 0.6855 | (0.6326, 0.7385) | 1/day |
β1A | 0.1465 | (0.1185, 0.1746) | 1/day |
δ0 | 0.0561 | (0.0439, 0.0683) | 1/day |
δ1 | 0.0211 | (0.0171, 0.0251) | 1/day |
τδ | 13.9249 | (0, 29.7695) | day |
γ0 | 0.0708 | (0.0556, 0.0860) | 1/day |
γ1 | 0.0675 | (0.0577, 0.0774) | 1/day |
τγ | 17.4348 | (11.2411, 23.6284) | day |
w | 0.25 | (fixed) | 1/day |
p | 0.12 | (fixed) | – |
Parameter | Best fit value for Mexico | 95% CI | Unit |
β0I | 0.5534 | (0.4779, 0.6290) | 1/day |
β1I | 0.1069 | (0.0800, 0.1339) | 1/day |
τβ | 31.9108 | (27.8195, 36.0022) | day |
β0A | 0.6855 | (0.6326, 0.7385) | 1/day |
β1A | 0.1465 | (0.1185, 0.1746) | 1/day |
δ0 | 0.0561 | (0.0439, 0.0683) | 1/day |
δ1 | 0.0211 | (0.0171, 0.0251) | 1/day |
τδ | 13.9249 | (0, 29.7695) | day |
γ0 | 0.0708 | (0.0556, 0.0860) | 1/day |
γ1 | 0.0675 | (0.0577, 0.0774) | 1/day |
τγ | 17.4348 | (11.2411, 23.6284) | day |
w | 0.25 | (fixed) | 1/day |
p | 0.12 | (fixed) | – |