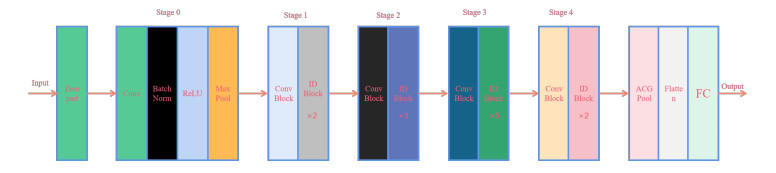
The emergence of COVID-19 has broken the silence of humanity and people are gradually becoming concerned about pneumonia-related diseases; thus, improving the recognition rate of pneumonia-related diseases is an important task. Neural networks have a remarkable effectiveness in medical diagnoses, though the internal parameters need to be set in accordance to different data sets; therefore, an important challenge is how to further improve the efficiency of neural network models. In this paper, we proposed a learning exponential distribution optimizer based on chaotic evolution, and we optimized Resnet50 for COVID classification, in which the model is abbreviated as IEDO-net. The algorithm introduces a criterion for judging the distance of the signal-to-noise ratio, a chaotic evolution mechanism is designed according to this criterion to effectively improve the search efficiency of the algorithm, and a rotating flight mechanism is introduced to improve the search capability of the algorithm. In the computed tomography (CT) image data of COVID-19, the accuracy, sensitivity, specificity, precision, and F1 score of the optimized Resnet50 were 94.42%, 93.40%, 94.92%, 94.29% and 93.84%, respectively. The proposed network model is compared with other algorithms and models, and ablation experiments and convergence and statistical analyses are performed. The results show that the diagnostic performance of IEDO-net is competitive, which validates the feasibility and effectiveness of the proposed network.
Citation: Chengtian Ouyang, Huichuang Wu, Jiaying Shen, Yangyang Zheng, Rui Li, Yilin Yao, Lin Zhang. IEDO-net: Optimized Resnet50 for the classification of COVID-19[J]. Electronic Research Archive, 2023, 31(12): 7578-7601. doi: 10.3934/era.2023383
[1] | Yazao Yang, Haodong Tang, Tangzheng Weng . Changes in public travel willingness in the post-COVID-19 era: Evidence from social network data. Electronic Research Archive, 2023, 31(7): 3688-3703. doi: 10.3934/era.2023187 |
[2] | Khongorzul Dashdondov, Mi-Hye Kim, Mi-Hwa Song . Deep autoencoders and multivariate analysis for enhanced hypertension detection during the COVID-19 era. Electronic Research Archive, 2024, 32(5): 3202-3229. doi: 10.3934/era.2024147 |
[3] | Comfort Ohajunwa, Carmen Caiseda, Padmanabhan Seshaiyer . Computational modeling, analysis and simulation for lockdown dynamics of COVID-19 and domestic violence. Electronic Research Archive, 2022, 30(7): 2446-2464. doi: 10.3934/era.2022125 |
[4] | Hao Nong, Yitan Guan, Yuanying Jiang . Identifying the volatility spillover risks between crude oil prices and China's clean energy market. Electronic Research Archive, 2022, 30(12): 4593-4618. doi: 10.3934/era.2022233 |
[5] | Xu Xin, Xiaoli Wang, Tao Zhang, Haichao Chen, Qian Guo, Shaorui Zhou . Liner alliance shipping network design model with shippers' choice inertia and empty container relocation. Electronic Research Archive, 2023, 31(9): 5509-5540. doi: 10.3934/era.2023280 |
[6] | Zhiliang Li, Lijun Pei, Guangcai Duan, Shuaiyin Chen . A non-autonomous time-delayed SIR model for COVID-19 epidemics prediction in China during the transmission of Omicron variant. Electronic Research Archive, 2024, 32(3): 2203-2228. doi: 10.3934/era.2024100 |
[7] | S. Suganya, V. Parthiban, R Kavikumar, Oh-Min Kwon . Transmission dynamics and stability of fractional order derivative model for COVID-19 epidemic with optimal control analysis. Electronic Research Archive, 2025, 33(4): 2172-2194. doi: 10.3934/era.2025095 |
[8] | Xiaoyan Wu, Guowen Ye, Yongming Liu, Zhuanzhe Zhao, Zhibo Liu, Yu Chen . Application of Improved Jellyfish Search algorithm in Rotate Vector reducer fault diagnosis. Electronic Research Archive, 2023, 31(8): 4882-4906. doi: 10.3934/era.2023250 |
[9] | Jorge Rebaza . On a model of COVID-19 dynamics. Electronic Research Archive, 2021, 29(2): 2129-2140. doi: 10.3934/era.2020108 |
[10] | Liling Huang, Yong Tan, Jinzhu Ye, Xu Guan . Coordinated location-allocation of cruise ship emergency supplies under public health emergencies. Electronic Research Archive, 2023, 31(4): 1804-1821. doi: 10.3934/era.2023093 |
The emergence of COVID-19 has broken the silence of humanity and people are gradually becoming concerned about pneumonia-related diseases; thus, improving the recognition rate of pneumonia-related diseases is an important task. Neural networks have a remarkable effectiveness in medical diagnoses, though the internal parameters need to be set in accordance to different data sets; therefore, an important challenge is how to further improve the efficiency of neural network models. In this paper, we proposed a learning exponential distribution optimizer based on chaotic evolution, and we optimized Resnet50 for COVID classification, in which the model is abbreviated as IEDO-net. The algorithm introduces a criterion for judging the distance of the signal-to-noise ratio, a chaotic evolution mechanism is designed according to this criterion to effectively improve the search efficiency of the algorithm, and a rotating flight mechanism is introduced to improve the search capability of the algorithm. In the computed tomography (CT) image data of COVID-19, the accuracy, sensitivity, specificity, precision, and F1 score of the optimized Resnet50 were 94.42%, 93.40%, 94.92%, 94.29% and 93.84%, respectively. The proposed network model is compared with other algorithms and models, and ablation experiments and convergence and statistical analyses are performed. The results show that the diagnostic performance of IEDO-net is competitive, which validates the feasibility and effectiveness of the proposed network.
As the quality of human life improves, so do the diseases that need to be diagnosed using current technologies through early detection and treatment to reduce pain and suffering [1,2]. The most recent outbreak of COVID-19 has led to a worldwide crisis at various levels, which has not yet been eradicated; moreover other pneumonia-related diseases are attacking us, making it important to further improve the diagnosis of these diseases [3].
In recent years, scholars have proposed many methods to diagnose and identify diseases, and deep learning models are a powerful and beneficial part of effective disease diagnoses [4,5,6]. For example, Hussain et al. proposed a novel convolutional neural network (CNN) network of CoroDet to perform the diagnoses of multiple classifications from X-ray and CT scan images of the chest [7]. Ozdemir generated six-axis mapped images from electrocardiograph (ECG) data a via grey scale co-occurrence matrix (GLCM) and imported them into a new CNN for the diagnosis of COVID-19 [8]. Ismael fused multiple deep CNN models and multiple kernel functions of support vector machine (SVM) classifiers trained in an end-to-end manner to diagnose a dataset of X-ray chest images [9]. Kc et al. performed diagnostic experiments on eight pre-trained models for COVID-19 and introduced migration learning techniques [10]. Muhammad and Hossain proposed a new CNN model with fewer parameters and properties [11]. Wang et al. developed a weakly-supervised deep learning framework that could accurately predict the probability of infection and lesion areas in patients [12]. However, it has the problem that different hyperparameters need to be set for different data sets or experimental environments in order to maximise the benefits of the model itself, so the setting of hyperparameters is an important challenge. The metaheuristic algorithm is widely used for the optimization effect of depth models, and it has the advantage of improved robustness and global optimization capability [13]. In the past two decades, research on metaheuristics has been gradually advanced [14,15], and scholars have proposed classical algorithms such as particle swarm optimization (PSO) [16], differential evolution (DE) [17], ant colony optimization (ACO) [18], etc. Some of the more popular algorithms in recent years are grey wolf optimizer (GWO) [19], whale optimization algorithm (WOA) [20], sparrow search algorithm (SSA) [21], harris hawks optimization (HHO) [22], manta ray foraging optimization (MRFO) [23], exponential distribution optimizer (EDO) [24], etc. All these algorithms have been better used in the field of COVID-19 classification and diagnoses. For example, Dixit et al. incorporated DE and PSOs for optimal feature extraction, and fed the optimized features to an SVM to obtain an improved accuracy [25]. Júnior et al. used multiple CNN models to extract depth features and used PSO optimized eXtreme Gradient Boosting (XGBoost) for classification [26], which included feature extraction using mel frequency cepstral coefficients (MFCCs) and classification using an PSO optimized learning machine (ELM) [27]. Elaziz et al. fused DE with MRFO to optimize the k-nearest neighbor (KNN) classifier and obtained better results [28]. El-Kenawy et al. used an improved advanced squirrel search algorithm (ASSOA) to optimize the multilayer perceptron and achieved certain classification results [29]. Pathan et al. combined the whale algorithm with the Bat algorithm (BAT) to optimize the CNN and performed classification by ADaBoost [30]. Basu et al. proposed a local search based and acoustic search algorithm for feature extraction of COVID-19 and used multiple deep convolutional networks for classification [31]. Nadimi-Shahraki et al. proposed a binary enhanced WOA for COVID-19 feature extraction to obtain an improved recognition accuracy [32]. Elghamrawy and Hassanien proposed a diagnostic and prediction model optimized by a WOA [33]. Goel et al. optimized a CNN with a GWO to have an improved classification accuracy of chest X-ray images [34]. Hu et al. fused a CNN with an extreme learning machine (ELM) and used the Chimp optimization algorithm to improve the reliability of the net training [35]. Singh et al. proposed a multi-objective differential evolution algorithm to tune the CNN to find the maximum classification accuracy [36]. Iraji et al. used a binary differential evolution algorithm to extract features and classify them by SVM [37]. Sahan et al. used an artificial bee colony algorithm to extract features and classify them with a multilayer perceptron [38]. Sadeghi et al. proposed a novel deep learning framework with a novel multi-habitat migrating artificial bee colony (MHMABC) algorithm for optimized training [39]. Balaha et al. proposed a HHO to optimize multiple pre-trained networks and improve the classification accuracy of COVID-19 [40]. Bahgat et al. optimized 12 architectures of MRFO for CNN to improve the classification performance [41]. Currently, more and more optimized models are proposed, though the design of the algorithm may not take into account the reliability of the iterative optimization of the algorithm, as the time and resources spent in the optimization process are huge; on the other hand, the learning ability of the network model is based on a large number of samples trained, thus improving the learning ability of finite samples is an important task. We need to find a reliable solution at each iteration that the efficiency of the model can improve the efficiency of the model and the work can be meaningful.
Based on the aforementioned analyses, while considering the reliability and learning at each iteration, this paper proposes a learning exponential distribution optimizer with a chaotic evolutionary mechanism, introduces a signal-to-noise distance to judge the distance of individuals, selects a suitable chaotic evolutionary mechanism by distance, and introduces a rotating flight strategy to enhance the local search capability of the algorithm. The hyperparameters of the optimized Resnet50 network model were put into practice and diagnosed in COVID-19 images. The experimental results show that the classification accuracy using the optimized Resnet50 network model is high. The specific work and innovations are as follows:
· a balanced individual selection method based on signal-to-noise distance is proposed;
· a chaos-based evolutionary mechanism is proposed;
· an IEDO algorithm and optimize the hyperparameters of Resnet50 in proposed;
· the model is compared with a variety of other algorithms and models in the COVID-19 dataset.
The overall structure of this paper is as follows: Section 2 describes Resnet50 and the original EDO algorithm; Section 3 describes and analyses the proposed algorithm; Section 4 describes the entire experimental procedure and methodology; Section 5 performs the COVID-19 classification experiments and analysis; and the last section concludes the paper and presents future work.
ResNet is a deep CNN model that is used as the backbone of the model due to its excellent performance [42]. Resnet50 is a 50-layer version of ResNet, in which the residual connection and residual block structures are implemented, with each residual block containing two convolutional layers and one residual connection. By implementing the aforementioned structures, Resnet50 greatly alleviates the problem of gradient disappearance and gradient explosion during training, and has a high accuracy and generalisation capability [43].
The first 49 layers of the Resnet50 network are convolutional and the final layer is a fully connected layer, whose structure can be divided into seven parts. As shown in Figure 1, Stage 0 performs the computation of convolution, regularization, activation function and maximum pooling without residual blocks; Stage 1 through 4 contain residual blocks. At the beginning, an image of size 224 × 224 × 3 represents the input; after the first five convolution layers, the output is a feature map of size 7 × 7 × 2048. After a utilizing pooling layer to convert the features into feature vectors, the classifier calculates and outputs the category probabilities. Resnet50 has a complex structure, and the internal hyperparameters also affect the learning efficiency between layers when facing different datasets. An important work of this paper is to further improve the classification recognition rate of Resnet50.
The EDO algorithm can be briefly described as the following process:
a. Generate N sets of solutions using a random distribution technique, with D values in each set of solutions, and set the corresponding maximum number of iterations (termination condition).
b. Start the iteration by constructing a memoryless zero matrix to simulate the memoryless nature of the algorithm, with a size equal to the overall initial generation.
c. During the development phase, the memoryless matrix is used to simulate the memoryless properties in order to preserve the previously generated solutions, regardless of their history, which will become important members for updating the new solutions. As a result, these solutions were divided into two categories: winners and losers. In addition, some features of the exponential distribution are used, such as mean, exponential rate and variance, are used. The winners will move in the direction of the bootstrapped solution, while the losers will move in the direction of the winner, with the aim of finding a global optimum around it.
d. In the exploration phase, the new solution uses two winners chosen at random from the original population and updates the mean solution. Initially, both the mean solution and the variance are far from the global optimum. The distance between the mean solution and the global optimum gradually decreases until a minimum is reached through the optimization process.
e. A switch parameter is used to determine whether to perform the exploration phase or the exploitation phase, where a is a uniform random number between [0, 1]. If a<0.5, then the exploitation is carried out as follows:
Xt+1i={r1⋅(mlti−σ2)+r2⋅XtguideifXtwin,i=mltir2⋅(mlti−σ2)+log(∅)⋅Xtwin,iotherwise | (2.1) |
If a≥0.5, then the exploration is carried out as follows:
Xt+1i=Xtwin,i−Mt+(r3⋅Z1+(1−r−3)⋅Z2) | (2.2) |
Xtguide=Xtwin,best1+Xtwin,best2+Xtwin,best33 | (2.3) |
r3=d×q;d=1−tM | (2.4) |
Z1=M−D1+D2;Z2=M−D2+D1 | (2.5) |
D1=M−Xwin,RD1;D2=M−Xwin,RD2 | (2.6) |
f. In the above equation, r1=(q)10,r2=(q)5, q is a uniform random number between [-1, 1]. ∅ is a uniform random number between [0, 1]. M represents the mean value of the population, and mlti is the i-th winner at the current stage. Xtguide is the guiding solution at t iterations. Xtwin,best1, Xtwin,best2, Xtwin,best3 are the top three best solutions in the matrix. Xwin,RD1 and Xwin,RD2 denote randomly selected individuals within the population.
g. After generating the new solutions, check the boundaries of each solution. Then, the solutions are saved in a memoryless matrix.
h. Use greedy mechanisms to update new solutions from the development and exploration phases of the original population. If the new solution is good, it will be updated in the original population.
e. If the termination condition is reached, the algorithm ends and the final optimal solution and position are outputs; otherwise, it returns to the second step.
During an algorithmic search, it is important to be able to accurately determine the location distribution of individuals in order to accurately implement different search solutions. Most scholars currently use the Euclidean distance to measure the distance between individuals in a population, which is simple to calculate but has some uncertainty in high-dimensional situations. Therefore, scholars have introduced the determination of distance; for example, Yang et al. used the ratio to determine the location of the local and global optima in the particle swarm algorithm [44], and Zhu et al. used the signal-to-noise ratio (SNR) to determine the location information of the bare bones particle swarm optimization [45]. The experimental results show that the SNR-based distance metric can yield more discriminative features than the euclidean distance-based distance metric; therefore, this paper introduces the SNR distance to measure different situations between different data, and the SNR distance between data and is defined as follows:
ds(pi,pj)=v(hj−hi)v(hi)=v(nij)v(hi) | (2.7) |
where v(x)=∑ni=1(xi−μ)2)n denotes the variance of x, μ denotes the mean of x and n denotes the dimensionality of x. Longer SNR distances indicate larger differences between the anchor and the comparison data; therefore, the signal-to-noise ratio distance measure can be used as a similarity measure instead of the Euclidean distance measure in metric learning.
When the algorithm performs position updating, it often performs a certain range of searches based on the optimal position; if the optimal position falls into the trap of local extremes, then it will bring a great disturbance to the subsequent way of finding the optimum solution. The traditional way is to select an individual at random; however, such an individual cannot guarantee the trend of the individual searching for the optimum, as it may be the worse position, thus giving the algorithm a negative effect and finding an unreasonable solution. Kahraman et al. proposed the fitness-distance balance (FDB) method [46], which uses the difference in fitness values and the euclidean distance of positions to generate representative equilibrium individuals within a population. Different from FBD, this paper adopts SNR in the optimization process of the algorithm instead of the Euclidean distance. The Euclidean distance is related to the dimension: the larger the dimension, the higher the computational complexity. Compared with the Euclidean distance, the advantage of SNR lies within calculating the simpler, and the computational complexity of the lower, which can effectively improve the optimization efficiency of the algorithm. As mentioned above, the representation of the Euclidean distance in the high-dimensional case is uncertain; therefore this paper introduces a new selection of balanced individuals, using the SNR distance and the distance of fitness values to generate a new balanced individual for the subsequent search for superiority. First, calculate the distance DS between each individual and the optimal SNR ratio using the following equation:
DSi=v(xi−xbest)v(xbest) | (3.1) |
i denotes the i-th individual in the population, thus resulting in a DS that is an N*1 matrix. Then, the DS and F are normalized and multiplied separately to obtain an evaluation score:
scorei=norm(DSi)∗norm(Fi) | (3.2) |
The purpose of normalization is to normalize the SNR distances to a certain range, which improves the reliability of the balanced individuals by eliminating the influence of the anomalous positions on the positions of other individuals to some extent. Additionally, the obtained score is an N*1 matrix, where the individual with the highest score value is designated as the balanced individual BI.
When faced with a complex optimization environment, the algorithm is tested to a greater extent, and the inefficiency of the search in the iterative optimization increases. An important challenge is how to improve the efficiency of the algorithm at each step of the search. An example of this is the optimization process of the algorithm on the sphere algorithm. In medical image problems, the optimization efficiency of an algorithm is also of paramount importance, and an algorithm with a less invalid search is needed. Therefore, this paper designs a new evolutionary mechanism that dynamically adjusts the position of an individual by determining the relationship between the current local optimum, the previous local optimum and the global optimum.
The last local optimum is set as LX, the current local optimum is CX, and the last historical optimum Lbest. It is worth noting that the last historical optimum Lbest must be better than LX. Taking the minimization problem as an example, the settings are as follows:
a. If f(CX)<f(Lbest), then it indicates that the quality of the previous population was worse than that of the current population, thus indicating that the current optimization trend is reasonable. Therefore, it is necessary to conduct a search around CX.
Xt(t+1)=CX+logistic⋅(Xm(t)−mli(t)) | (3.3) |
logistic represents a logistic sequence of 1 × dim, where the logistic sequence generation schematic is shown in Figure 2. The logistic sequence is able to produce a more uniform distribution of values, which makes the algorithm search more comprehensive and enables it to balance the global exploration of the algorithm with local exploitation to better explore solutions compared to CX. Xm represents the average position of the population.
b. If f(LX)>f(CX)>f(Lbest), then it means that a solution better than Lbest is not found twice in a row, but the quality of the position in CX is higher than that of LX, and a local search between CX and Lbest needs to be considered.
Xi(t+1)=CX+tent⋅(Lbest−CX) | (3.4) |
Tent denotes a tent sequence of 1 × dim, and the tent sequence generation schematic is shown in Figure 3. Most of the distribution produced by the tent sequence is concentrated above 0.5. The current population is not able to find a better solution, and it needs to improve a certain global exploration ability to effectively improve the situation; therefore, the tent sequence can better meet this need, which can gradually make CX close to Lbest, and further get rid of the current dilemma.
c. If f(Lbest)<f(LX)<f(CX), then it indicates that the interval between two searches is invalid, and the quality of the current population is worse than that of the previous generation, thus indicating that the population may fall into a local extreme state. It is necessary to consider improving the global search ability of the population and finding a reasonable optimal position. The specific formula is as follows:
Xi(t+1)=BI+gaussian⋅(Lbest−mli(t)) | (3.5) |
Gaussian represents a gaussian sequence of 1 × dim, and the gaussian sequence generation schematic is shown in Figure 4. BI is the balanced individual produced earlier, thus causing the population to converge to different positions and improving the search ability through the difference between Lbest and the current individual. The gaussian sequence produces slightly more values below 0.5, which allows the current individual to approach the optimal solution faster and improve the accuracy of the solution.
Taking the aforementioned analyses into account, the generation of three chaotic sequences is able to produce different search methods, which sufficiently improves the accuracy of the search. In order to verify that this mechanism can improve the search efficiency of the algorithm, this section compares the original EDO algorithm with EDO (C+EDO) with the addition of the chaotic evolution mechanism using the Sphere function for the experiment; the number of population iterations are both at 50, and the optimal objective function values obtained from each of them are presented in Figure 5. Figure 5 shows that the search of the C+EDO algorithm at each iteration is better than the previous one, or at least is not worse than the previous solution; EDO appears to be an invalid search, though it is impossible to avoid the occurrence of invalid search, and the least possible while improving the search efficiency of the algorithm. Through the aforementioned analysis, the feasibility of the chaotic evolution mechanism can be verified, so as to improve the efficiency of the iterative search of the algorithm. It is worth noting that the aforementioned judgement conditions do not require an additional number of calculations, as they are automatically obtained during the algorithm optimization process.
The algorithm is required to enhance the local exploration capability when searching for a pair of directions, so as to improve the accuracy of the solution. RFS is a flight mechanism proposed by Zheng et al. to enhance the diversity of the candidate solutions [47]. The specific equation is as follows:
Zi(t+1)={K1iff(K1)<f(K2)K2otherwise | (3.6) |
{K1=Zbest−H1,K2=Zbest−H2rand<0.5K1=Zbest+H1,K2=Zbest+H2otherwise | (3.7) |
{H1=rand×((Zbest−Zi(t)×Fi(t))×cos(2×Zi(t)×Zbest)H2=rand×((Zbest−Zi(t)×Fi(t))×sin(2×Zi(t)×Zbest) | (3.8) |
Fi(t)=(2×rand+1)×a×(1−tT)+b | (3.9) |
b=c×(sin2.5(π2×tT)+cos((π2×tT)−1) | (3.10) |
In the above equation, T is the maximum number of iterations; a is [-1, 1], c is [-2, 2], and both are random numbers. Zbest is the optimal individual. Zi(t+1) is the position of the newly generated individual. The above equation shows that RFS is dynamically adjusted to the candidate solutions by the sin and cos functions, which facilitates the exploration of local solutions.The change of F fluctuates with the number of iterations, which has a better global and local search ability. From Eqs (14) and (15), the newly generated individual positions are adjusted by F to adjust the size of the spiral search around the optimal position and better retain the solution through the greedy mechanism, so it can improve the diversity of the solution and speed up the search efficiency.
In order to improve the search efficiency of the algorithm during the search process and reduce the probability of the algorithm falling into a local optimum, this paper proposes a learning EDO algorithm based on chaotic evolution, which combines the balanced individual of signal-to-noise distance selection with the chaotic evolution mechanism, and finally introduces a RFS, so as to further improve the global and local search capability of the algorithm. The specific pseudo-code is as follows:
Algorithm 1 IEDO |
Input: Population size (N), dimensionality of the problem (d), and upper and lower bounds. |
Output: Optimal solution position Xtwin,best and optimal solution fmin |
1: Random initialization to obtain a Xtwin matrix |
2: Find the optimal solution fmin and the optimal location of the optimal solution Xtwin according to the fitness function |
3: t = 1 |
4: Generate a memoryless matrix ml = Xwin |
5: while (t≤T) do |
6: rank the populations according to their fitness values to obtain the top three best individuals |
7: Calculate Eq (2.3) |
8: Balancing the selection of individuals |
9: Generate sequences of logistic, tent, gaussian, respectively based on dimensions |
10: if f(x)<f(Lbest) then |
11: update the Eq (3.3) % logistic sequence |
12: else if f(Lx)>f(Cx)>f(Lbest) then |
13: update the Eq (3.4) % tent sequence |
14: else |
15: update the Eq (3.5) % gaussian sequence |
16: end if |
17: for i = 1:N do |
18: if α<0.5 then |
19: update the Eq (2.1) |
20: else |
21: update the Eq (2.2) |
22: end if |
23: end for |
24: for i = 1:N do |
25: update the Eq (3.6) % RFS |
26: end for |
27: Calculate the fitness values within the population and obtain the optimal solution fmin and Xtwin,best |
28: t = t + 1 |
29: end while |
Return Outputs |
In meta-heuristic algorithms, a time complexity analysis is one of the indicators to verify the effectiveness of the algorithms, which can reflect the time efficiency of the algorithms. Taking the most classical PSO and DE algorithms as examples, their time complexity is equal to the product of the number of populations and the number of iterations and dimensions; therefore, their time complexity is O(TND). EDO is the same as them, and the time complexity is also O(TND). In the proposed algorithm, the time complexity of the selection process of balancing individuals is O(ND), the time complexity of each calculation of the evolution mechanism is O(ND), the time complexity of the calculation of the spiral flight mechanism is O(ND), and the whole process does not add additional iterations. Then, the final time complexity of IEDO is O(T(ND+ND+ND))=O(TND). Therefore, the time complexity of IEDO is increased in a microscopic way, but it does not cause an order of magnitude change, so the improvement in effectiveness is of some significance and worth [48].
Resnet50 is one of the DL models with a unique feature extraction for input images during image classification [49]. The extraction of features in Resnet50 is preformed by several convolutional and pooling layers. A fully connected softmax layer performs the classification. The setting of hyperparameters affects the training capability of the network; therefore, IEDO needs to be used to more rationally optimize these parameters to maximize the classification capability of the network as much as possible. The hyperparameters to be optimized in this paper include Momentum, InitialLearnRate, MaxEpochs, and ValidationFrequency, which are first optimized using IEDO and then trained and tuned using a learning gradient with momentum (SGDM) algorithm. The flow chart of the hyperparameters using the IEDO algorithm Resnet50 is shown in Figure 6. The general process is described as follows:
a. Initialize the population and obtain a solution with random hyperparameters.
b. Use the classification error rate of the model as the objective function, keeping the identification labels corresponding to the minimum error rate.
c. Import the above solution into the SGDM; then train and optimize through it to obtain the classification results.
d. Update the IEDO flow (lines 5–28 in Algorithm 1).
e. Obtain the final accuracy and the corresponding recognition labels.
In this paper, CT images (https://github.com/UCSD-AI4H/COVID-CT) were used for classification. The experimental environment is matlab2019a. Matlab 2019a is capable of being able to achieve faster computational speeds, as well as being able to support multiple languages and multiple deep learning model toolkit calls, so our tool of choice is matlab 2019a with a 13th Gen Intel(R) Core(TM) i9-13900KF 3.00 GHz processor, and 32.0 GB of onboard RAM. IEDO is used to optimize Resnet50 and perform COVID-19 classification. The CT dataset is a balanced dataset, there are a total of 349 COVID images and 397 non-COVID images in CT images, and the dataset is divided into a ratio of 0.7. the image size is adjusted to 224 × 224 × 3, and SGDM is used to train these parameters tuning.
To further illustrate the improved optimization efficiency of the proposed algorithm compared to EDO, the optimal accuracy found for each iteration is shown in Figure 7 (solid line). The number of populations is set to 10 and the maximum number of evaluations is set to 100. The number of evaluations can simply be understood as the number of times the objective function is calculated, and it is set to ensure a fair comparison between the algorithms.
As can be seen in Figure 4, IEDO converges faster and with a higher convergence accuracy; it reaches its highest accuracy at 60 evaluations. On the other hand, IEDO is more stable than EDO in terms of the recognition accuracy, although invalid searches occur, but improves the latter search accuracy. For example, IEDO reaches about 0.86 at 20 evaluations, but reaches 0.92 at 30+ evaluations, which is better than the previous search; there is a decline in the recognition accuracy at 40+ evaluations as compared to +50 evaluations for IEDO. On the contrary, EDO is still in an invalid state at 30+ evaluation times, which is smaller than the previous recognition accuracy, and only finds the highest recognition accuracy of the process at 50+ evaluation times. Due to its improved convergence efficiency, Resnet50 after IEDO can find a better recognition accuracy faster and improve the recognition efficiency of the model.
In this section, EDO and IEDO are independently run for 10 times, and the best value, mean value and worst value of the data are each recorded for 10 times. Meanwhile, the Wilkerson test will be used to analyze the difference between the data of 10 times, with a significance level of 0.05; if the P-value is less than 0.05, it means that the performance of the two algorithms method is different, and the specific results are shown in the Table 1.
Method | Best | Mean | Worst | P |
EDO | 90.63% | 90.23% | 89.73% | 0.01% |
IEDO | 94.42% | 93.08% | 91.52% | - |
From Table 1, the average value and the worst value of IEDO are better, which verifies that IEDO has a certain stability and competitiveness; on the other hand, the P-value of the two algorithms is 0.01%, which indicates that there is a certain difference in the performance of the two algorithms. Through the statistical results, it can be seen that EDO has a clear advantage in the value, which proves that the improvement of IEDO is somewhat effective.
To further verify the effectiveness of the selected network, this section compares the optimized Resnet with various basic neural network models (i.e., Inception [50], Vgg16 [51,52], Vgg19 [53], Alexnet [54]). A comparison was made as a way to see the advantages of Resnet. The Momentum, InitialLearnRate, MaxEpochs, and ValidationFrequency of these networks are 0.9, 0.01, 20, and 30, respectively. These networks are the most widely researched models, and there are a number of continually improved versions proposed that are valuable to compare with these models.The classification result table for each model is shown in Table 2.
Algorithms | Inception | Vgg16 | Vgg19 | Alexnet | Resnet | IEDO-net |
Accuracy | 73.21% | 53.13% | 53.13% | 67.41% | 87.95% | 94.42% |
From Table 2, we can see that the recognition accuracy of IEDO-net is the highest, with a recognition rate of 94.42%. The recognition accuracy of Resnet without optimization is 87.95%, and the recognition accuracy of other models is lower; thus, it can be seen that it is more meaningful to optimize Resnet.
In order to better demonstrate the effectiveness of the algorithms and models for classification, the performance of the technique must be validated using metrics such as accuracy, sensitivity, specificity, precision, F1 score values, confusion matrix (CM) and receiver operating characteristic (ROC) [55,56].
To demonstrate the optimization capability of IEDO, IEDO was compared with the basic algorithms and algorithms used in recent years for pneumonia diagnoses, including PSO, WOA, MRFO, DE, EDO, GWO, DEPSO [25], WOABAT [30], ASSOA [29], and the experimental parameters required to be set for each algorithm are shown in Table 3. To further verify the effectiveness of IEDO, the algorithms were individually arranged, combined and experimented with separately. The algorithm with the chaotic evolution mechanism alone was set as EDO-1, and the algorithm with RFS was set as EDO-2. The diagnostic results for each model are tabulated in Table 4. The confusion matrix and ROC diagram of each algorithm are shown in Figures 8 and 9, respectively. In the ROC plot, the true negative rate (TNR) represents the proportion of samples that were predicted to be positive and were actually positive as a percentage of all positive samples, and the false positive rate (FPR) represents the proportion of samples that were predicted to be positive but were actually negative as a percentage of all negative samples.
Algorithms | Parameters |
PSO | c1=c2=2;ω=0.729 |
DE | F=0.5;CR=0.3 |
DEPSO | Iω=0.9;a1=a2=2.05;Fmin=0.5;Fmax=1;CRmax=1.0;CRmin=0.8;α=0.15 |
Method | Accuracy | Sensitivity | Specificity | Precision | F1 score |
PSO | 86.61% | 89.47% | 84.50% | 80.95% | 85.00 |
WOA | 91.07% | 92.08% | 90.24% | 88.57% | 90.29% |
MRFO | 87.95% | 86.79% | 88.98% | 87.62% | 87.20% |
DE | 83.93% | 83.50% | 84.30% | 81.90% | 82.69% |
EDO | 89.73% | 85.96% | 93.64% | 93.33% | 89.50% |
GWO | 89.73% | 91.00% | 88.71% | 86.67% | 88.78% |
DEPSO [25] | 91.52% | 89.81% | 93.10% | 92.38% | 91.08% |
WOABAT [30] | 90.18% | 91.92% | 88.80% | 86.67% | 89.22% |
ASSOA [29] | 91.07% | 92.08% | 90.24% | 88.57% | 90.29% |
EDO-1 | 91.52% | 93.88% | 89.68% | 87.62% | 90.64% |
EDO-2 | 91.96% | 89.91% | 93.91% | 93.33% | 91.59% |
IEDO | 94.42% | 93.40% | 94.92% | 94.29% | 93.84% |
It can be seen from Figure 5 and Table 3 that IEDO and the other basic algorithms for pneumonia diagnoses are tested under the same circumstances, and the algorithm is ranked from the highest accuracy to the lowest accuracy. It can be concluded that the accuracy of the DE algorithm in the diagnoses of pneumonia was 83.93%, the number of correct diagnoses of normal was 102, and the number of correct diagnoses of COVID was 86. The accuracy of the PSO algorithm in the diagnoses of pneumonia was 86.61%, the number of correct diagnoses of normal was 109, and the number of correct diagnoses of COVID was 85. The accuracy of the MRFO algorithm in diagnoses of pneumonia was 87.95%, the number of correct diagnoses of normal was 105, and the number of correct diagnoses of COVID was 92. The accuracy of the EDO algorithm in the diagnoses of pneumonia was 89.73%, the number of correct diagnoses of normal was 103, and the number of correct diagnoses of COVID was 98. The accuracy of the GWO algorithm in the diagnoses of pneumonia was 89.73%, the number of correct diagnoses of normal was 110, and the number of correct diagnoses of COVID was 91. The accuracy of the WOA algorithm in the diagnoses of pneumonia was 91.07%, the number of correct diagnoses of normal was 111, and the number of correct diagnoses of COVID was 93. The accuracy of the ASSOA [24] algorithm in the diagnoses of pneumonia was 91.07%, the number of correct diagnoses as normal was 111, and the number of correct diagnoses as COVID was 93. The accuracy of the WOABAT [25] algorithm in the diagnoses of pneumonia was 90.18%, the number of correct diagnoses as normal was 111, and the number of correct diagnoses as COVID was 91. The accuracy of the DEPSO [20] algorithm in the diagnoses of pneumonia was 91.52%, the number of correct diagnoses as normal was 108, and the number of correct diagnoses of COVID was 97. The accuracy of the IEDO algorithm in the diagnoses of pneumonia was 94.20%, the number of correct diagnoses of normal was 112, and the number of correct diagnoses of COVID was 96.
It can be seen that compared with the other basic algorithms used for pneumonia diagnoses, the IEDO algorithm has the highest accuracy, surpassing all the other algorithms. The IEDO algorithm has the largest number of correct diagnoses. It can be seen from Table 3 that IEDO performs better than the other basic algorithms for pneumonia diagnosis in other aspects of performance, which can well reflect the superiority of IESO algorithm.
To further verify the effectiveness of IEDO using both chaotic evolution and spiral flight, we compared IEDO with an algorithm that does not use chaotic evolution (called EDO-1) and an algorithm that does not use spiral flight (called EDO-2). The accuracy of the EDO-1 algorithm in the diagnoses of pneumonia was 91.52%, the number of correct diagnoses of normal was 113, and the number of correct diagnoses of COVID was 92. The accuracy of the EDO-2 algorithm in the diagnosis of pneumonia was 91.96%, the number of correct diagnoses of normal was 108, and the number of correct diagnoses of COVID was 98. Comparing the above data with the data obtained by the IEDO algorithm, it can be concluded that the accuracy of EDO-1 and EDO-2 are lower than that of the IEDO algorithm. Although the EDO-1 algorithm has higher sensitivity, other performances have regressed. In the EDO-2 algorithm, all performances regressed. The simultaneous use of the two mechanisms can improve the performance of IEDO. Although some of the performance will be reduced, the number of performance decreases is small and not obvious, while most of the performances are improved, which reflects the rationality and performance superiority of the IEDO algorithm using the mixed mechanism.
The ROC curve shows that the curve of IEDO is closer to the (0, 1) position, thus indicating a better predictive capability of the IEDO optimized network: the higher the sensitivity and the lower the false positive rate, the better the performance of the diagnostic method.
To show that the IEDO optimized ground Resnet50 is more competitive, this subsection compares the recently proposed neural networks with it alongside the number of image samples. These neural network models include DRE-Net [57], MADE-DBM [58], DTL [59], Trans-CNN [60], CLAHE transform [61], and 8-CLF [62]. The specific recognition results are shown in Table 5.
Method | No. of images | Accuracy | Sensitivity | Specificity | Precision | F1 score |
DRE-Net | 1990 | 93.00% | 93.00% | 93.00% | 93.00% | 93.00% |
MADE-DBM | 1790 | 96.20% | 96.23% | 96.17% | - | 96.17% |
DTL | 852 | 93.02% | 91.46% | 94.78% | 95.19% | 93.29% |
Trans-CNN Net | 194922 | 96.73% | 97.76% | 96.01% | 97.45% | 96.36% |
CLAHE transform | 2482 | 94.56% | 91.00% | - | 95.00% | 93.00% |
8-CLF | 746 | 93.33% | 93.17% | 88.71% | 93.17% | 93.29% |
IEDO-net | 746 | 94.42% | 93.40% | 94.92% | 94.29% | 93.84% |
From Table 5, we can see that the IEDO network is better able to recognise the 746 CT images in the dataset, and purely in terms of metrics, the IEDO network ranks third in terms of its classification performance. The best result is the Trans–CNN network, which is first in all metrics, but has a large sample size. The next best result is MADE-DBM, which has a sample image size of 1790. The size of the number affects the accuracy of the model, which intuitively serves to increase the number of learning samples, but also increases the noise of the image; therefore, the strengths and weaknesses of the model cannot be analyzed purely in terms of recognition accuracy. Taken together, the IEDO network achieves reliable classification accuracy with a small number of samples, which is competitive and has some value and significance in intelligent healthcare.
In order to further improve the classification recognition rate of COVID, the paper proposed an optimized Resnet50 network model by IEDO (IEDO-net). IEDO introduces a signal-to-noise ratio distance determination selection on the basis of EDO to select a reasonable equilibrium individual and to reduce the probability of falling into a local optimum; then, it proposes a chaotic evolutionary mechanism to improve the efficiency of the algorithm search; finally, it introduces a spiral flight mechanism to improve the local search ability of the algorithm. In the CT dataset of COVID, the IEDO-net has a high classification accuracy and is compared with other networks to verify the feasibility of the IEDO-net, and the effectiveness of the algorithm is verified by ablation experiments.
Although IEDO-net has achieved some achievements, it has some problems. First, as we all know, there are many kinds of diseases, and the same disease may also have different categories; the classification experiment designed in this paper is relatively simple, while the network considered is more general. Second, the diagnostic accuracy of the model is high, but there is still an error rate, and it cannot completely replace the judgement of the treating professional doctor. Meanwhile, the training data is completely dependent on the given images, and the initial labels are also subject to human-set errors. Third, there is less privacy protection for the patients. Taken together, our next step is to consider maximizing privacy protection based on federated learning and improving the correct diagnosis rate of different diseases to greatly improve the reliability of smart healthcare. The main work is divided into the following three areas:
· designing more efficient meta-heuristic optimization algorithms;
· optimizing up-to-date and rational networks for diagnosis of more classes of diseases;
· and incorporating federated learning to maximize the efficiency of network models while protecting data privacy.
The authors declare they have not used Artificial Intelligence (AI) tools in the creation of this article.
The authors declare no conflict of interest.
[1] |
Q. Yuan, K. Chen, Y. Yu, N. Q. K. Le, M. C. H. Chua, Prediction of anticancer peptides based on an ensemble model of deep learning and machine learning using ordinal positional encoding, Briefings Bioinf., 24 (2023), bbac630. https://doi.org/10.1093/bib/bbac630 doi: 10.1093/bib/bbac630
![]() |
[2] |
N. Q. K. Le, Potential of deep representative learning features to interpret the sequence information in proteomics, Proteomics, 22 (2022), 2100232. https://doi.org/10.1002/pmic.202100232 doi: 10.1002/pmic.202100232
![]() |
[3] |
P. Aggarwal, N. K. Mishra, B. Fatimah, P. Singh, A. Gupta, S. D. Joshi, COVID-19 image classification using deep learning: Advances, challenges and opportunities, Comput. Biol. Med., 144 (2022), 105350. https://doi.org/10.1016/j.compbiomed.2022.105350 doi: 10.1016/j.compbiomed.2022.105350
![]() |
[4] |
O. S. Albahri, A. A. Zaidan, A. S. Albahri, B. B. Zaidan, K. H. Abdulkareem, Z. T. Al-qaysi, et al., Systematic review of artificial intelligence techniques in the detection and classification of COVID-19 medical images in terms of evaluation and benchmarking: Taxonomy analysis, challenges, future solutions and methodological aspects, J. Infect. Public Health, 13 (2020), 1381–1396. https://doi.org/10.1016/j.jiph.2020.06.028 doi: 10.1016/j.jiph.2020.06.028
![]() |
[5] |
T. W. Cenggoro, B. Pardamean, A systematic literature review of machine learning application in COVID-19 medical image classification, Procedia Comput. Sci., 216 (2023), 749–756. https://doi.org/10.1016/j.procs.2022.12.192 doi: 10.1016/j.procs.2022.12.192
![]() |
[6] |
Y. Hu, K. Liu, K. Ho, D. Riviello, J. Brown, A. R. Chang, et al., A simpler machine learning model for acute kidney injury risk stratification in hospitalized patients, J. Clin. Med., 11 (2022), 5688. https://doi.org/10.3390/jcm11195688 doi: 10.3390/jcm11195688
![]() |
[7] |
E. Hussain, M. Hasan, M. A. Rahman, I. Lee, T. Tamanna, M. Z. Parvez, CoroDet: A deep learning based classification for COVID-19 detection using chest X-ray images, Chaos, Solitons Fractals, 142 (2021), 110495. https://doi.org/10.1016/j.chaos.2020.110495 doi: 10.1016/j.chaos.2020.110495
![]() |
[8] |
M. A. Ozdemir, G. D. Ozdemir, O. Guren, Classification of COVID-19 electrocardiograms by using hexaxial feature mapping and deep learning, BMC Med. Inf. Decis. Making, 21 (2021), 170. https://doi.org/10.1186/s12911-021-01521-x doi: 10.1186/s12911-021-01521-x
![]() |
[9] |
A. M. Ismael, A. Şengür, Deep learning approaches for COVID-19 detection based on chest X-ray images, Expert Syst. Appl., 164 (2021), 114054. https://doi.org/10.1016/j.eswa.2020.114054 doi: 10.1016/j.eswa.2020.114054
![]() |
[10] |
K. Kc, Z. Yin, M. Wu, Z. Wu, Evaluation of deep learning-based approaches for COVID-19 classification based on chest X-ray images, Signal, Image Video Process., 15 (2021), 959–966. https://doi.org/10.1007/s11760-020-01820-2 doi: 10.1007/s11760-020-01820-2
![]() |
[11] |
G. Muhammad, M. S. Hossain, COVID-19 and non-COVID-19 classification using multi-layers fusion from lung ultrasound images, Inf. Fusion, 72 (2021), 80–88. https://doi.org/10.1016/j.inffus.2021.02.013 doi: 10.1016/j.inffus.2021.02.013
![]() |
[12] |
X. Wang, X. Deng, Q. Fu, Q. Zhou, J. Feng, H. Ma, et al., A weakly-supervised framework for COVID-19 classification and lesion localization from chest CT, IEEE Trans. Med. Imaging, 39 (2020), 2615–2625. https://doi.org/10.1109/TMI.2020.2995965 doi: 10.1109/TMI.2020.2995965
![]() |
[13] |
M. Riaz, M. Bashir, I. Younas, Metaheuristics based COVID-19 detection using medical images: A review, Comput. Biol. Med., 2022 (2022), 105344. https://doi.org/10.1016/j.compbiomed.2022.105344 doi: 10.1016/j.compbiomed.2022.105344
![]() |
[14] |
D. Zhu, S. Wang, C. Zhou, S. Yan, J. Xue, Human memory optimization algorithm: A memory-inspired optimizer for global optimization problems, Expert Syst. Appl., 237 (2024), 121597. https://doi.org/10.1016/j.eswa.2023.121597 doi: 10.1016/j.eswa.2023.121597
![]() |
[15] |
D. Zhu, S. Wang, J. Shen, C. Zhou, T. Li, S. Yan, A multi-strategy particle swarm algorithm with exponential noise and fitness-distance balance method for low-altitude penetration in secure space, J. Comput. Sci., 74 (2023), 102149. https://doi.org/10.1016/j.jocs.2023.102149 doi: 10.1016/j.jocs.2023.102149
![]() |
[16] | J. Kennedy, R. Eberhart, Particle swarm optimization, in Proceedings of ICNN'95-International Conference on Neural Networks, IEEE, (1995), 1942–1948. https://doi.org/10.1109/ICNN.1995.488968 |
[17] | K. V. Price, Differential evolution, in Handbook of Optimization: From Classical to Modern Approach, Springer, (2013), 187–214. https://doi.org/10.1007/978-3-642-30504-7_8 |
[18] | M. Dorigo, M. Birattari, T. Stutzle, Ant colony optimization, IEEE Comput. Intell. Mag., 1 (2006), 28–39. https://doi.org/10.1109/MCI.2006.329691 |
[19] | S. Mirjalili, S. M. Mirjalili, A. Lewis, Grey wolf optimizer, Adv. Eng. Software, 69 (2014), 46–61. https://doi.org/10.1016/j.advengsoft.2013.12.007 |
[20] |
S. Mirjalili, A. Lewis, The whale optimization algorithm, Adv. Eng. Software, 95 (2016), 51–67. https://doi.org/10.1016/j.advengsoft.2016.01.008 doi: 10.1016/j.advengsoft.2016.01.008
![]() |
[21] |
J. Xue, B. Shen, A novel swarm intelligence optimization approach: Sparrow search algorithm, Syst. Sci. Control Eng., 8 (2020), 22–34. https://doi.org/10.1080/21642583.2019.1708830 doi: 10.1080/21642583.2019.1708830
![]() |
[22] |
A. A. Heidari, S. Mirjalili, H. Faris, I. Aljarah, M. Mafarja, H. Chen, Harris hawks optimization: Algorithm and applications, Future Gener. Comput. Syst., 97 (2019), 849–872. https://doi.org/10.1016/j.future.2019.02.028 doi: 10.1016/j.future.2019.02.028
![]() |
[23] |
W. Zhao, Z. Zhang, L. Wang, Manta ray foraging optimization: An effective bio-inspired optimizer for engineering applications, Eng. Appl. Artif. Intell., 87 (2020), 103300. https://doi.org/10.1016/j.engappai.2019.103300 doi: 10.1016/j.engappai.2019.103300
![]() |
[24] |
M. Abdel-Basset, D. El-Shahat, M. Jameel, M. Abouhawwash, Exponential distribution optimizer (EDO): A novel math-inspired algorithm for global optimization and engineering problems, Artif. Intell. Rev., 56 (2023), 9329–9400. https://doi.org/10.1007/s10462-023-10403-9 doi: 10.1007/s10462-023-10403-9
![]() |
[25] |
A. Dixit, A. Mani, R. Bansal, CoV2-Detect-Net: Design of COVID-19 prediction model based on hybrid DE-PSO with SVM using chest X-ray images, Inf. Sci., 571 (2021), 676–692. https://doi.org/10.1016/j.ins.2021.03.062 doi: 10.1016/j.ins.2021.03.062
![]() |
[26] |
D. A. D. Júnior, L. B. da Cruz, J. O. B. Diniz, G. L. F. da Silva, G. B. Junior, A. C. Silva, et al., Automatic method for classifying COVID-19 patients based on chest X-ray images, using deep features and PSO-optimized XGBoost, Expert Syst. Appl., 183 (2021), 115452. https://doi.org/10.1016/j.eswa.2021.115452 doi: 10.1016/j.eswa.2021.115452
![]() |
[27] |
M. A. A. Albadr, S. Tiun, M. Ayob, F. T. AL-Dhief, Particle swarm optimization-based extreme learning machine for COVID-19 detection, Cognit. Comput., 2022 (2022), 1–16. https://doi.org/10.1007/s12559-022-10063-x doi: 10.1007/s12559-022-10063-x
![]() |
[28] |
M. A. Elaziz, K. M. Hosny, A. Salah, M. M. Darwish, S. Lu, A. T. Sahlol, New machine learning method for image-based diagnosis of COVID-19, PLoS One, 15 (2020), e0235187. https://doi.org/10.1371/journal.pone.0235187 \newpage doi: 10.1371/journal.pone.0235187
![]() |
[29] |
E. S. M. El-Kenawy, S. Mirjalili, A. Ibrahim, M. Alrahmawy, M. El-Said, R. M. Zaki, et al., Advanced meta-heuristics, convolutional neural networks, and feature selectors for efficient COVID-19 X-ray chest image classification, IEEE Access, 9 (2021), 36019–36037. https://doi.org/10.1109/ACCESS.2021.3061058 doi: 10.1109/ACCESS.2021.3061058
![]() |
[30] |
S. Pathan, P. C. Siddalingaswamy, P. Kumar, M. M. M. Pai, T. Ali, U. R. Acharya, Novel ensemble of optimized CNN and dynamic selection techniques for accurate COVID-19 screening using chest CT images, Comput. Biol. Med., 137 (2021), 104835. https://doi.org/10.1016/j.compbiomed.2021.104835 doi: 10.1016/j.compbiomed.2021.104835
![]() |
[31] |
A. Basu, K. H. Sheikh, E. Cuevas, R. Sarkar, COVID-19 detection from CT scans using a two-stage framework, Expert Syst. Appl., 193 (2022), 116377. https://doi.org/10.1016/j.eswa.2021.116377 doi: 10.1016/j.eswa.2021.116377
![]() |
[32] |
M. H. Nadimi-Shahraki, H. Zamani, S. Mirjalili, Enhanced whale optimization algorithm for medical feature selection: A COVID-19 case study, Comput. Biol. Med., 148 (2022), 105858. https://doi.org/10.1016/j.compbiomed.2022.105858 doi: 10.1016/j.compbiomed.2022.105858
![]() |
[33] | S. Elghamrawy, A. E. Hassanien, Diagnosis and prediction model for COVID-19 patient's response to treatment based on convolutional neural networks and whale optimization algorithm using CT images, preprint, MedRxiv, 2020. https://doi.org/10.1101/2020.04.16.20063990 |
[34] |
T. Goel, R. Murugan, S. Mirjalili, D. K. Chakrabartty, OptCoNet: An optimized convolutional neural network for an automatic diagnosis of COVID-19, Appl. Intell., 51 (2021), 1351–1366. https://doi.org/10.1007/s10489-020-01904-z doi: 10.1007/s10489-020-01904-z
![]() |
[35] |
T. Hu, M. Khishe, M. Mohammadi, G. Parvizi, S. H. T. Karim, T. A. Rashid, Real-time COVID-19 diagnosis from X-Ray images using deep CNN and extreme learning machines stabilized by chimp optimization algorithm, Biomed. Signal Process. Control, 68 (2021), 102764. https://doi.org/10.1016/j.bspc.2021.102764 doi: 10.1016/j.bspc.2021.102764
![]() |
[36] |
D. Singh, V. Kumar, M. Kaur, Classification of COVID-19 patients from chest CT images using multi-objective differential evolution–based convolutional neural networks, Eur. J. Clin. Microbiol. Infect. Dis., 39 (2020), 1379–1389. https://doi.org/10.1007/s10096-020-03901-z doi: 10.1007/s10096-020-03901-z
![]() |
[37] | M. S. Iraji, M. Feizi-Derakhshi, J. Tanha, Deep learning for COVID-19 diagnosis based feature selection using binary differential evolution algorithm, preprint, 2021, arXiv: PPR343118. |
[38] |
A. M. Sahan, A. S. Al-Itbi, J. S. Hameed, COVID-19 detection based on deep learning and artificial bee colony, Periodicals Eng. Nat. Sci., 9 (2021), 29–36. http://doi.org/10.21533/pen.v9i1.1774 doi: 10.21533/pen.v9i1.1774
![]() |
[39] | F. Sadeghi, O. Rostami, M. K. Yi, A deep learning approach for detecting COVID-19 using the chest X-ray images, CMC-Comput. Mater. Continua, 75 (2023), 751–768. |
[40] |
H. M. Balaha, E. M. El-Gendy, M. M. Saafan, CovH2SD: A COVID-19 detection approach based on Harris Hawks Optimization and stacked deep learning, Expert Syst. Appl., 186 (2021), 115805. https://doi.org/10.1016/j.eswa.2021.115805 doi: 10.1016/j.eswa.2021.115805
![]() |
[41] |
W. M. Bahgat, H. M. Balaha, Y. AbdulAzeem, M. M. Badawy, An optimized transfer learning-based approach for automatic diagnosis of COVID-19 from chest x-ray images, PeerJ Comput. Sci., 7 (2021), e555. https://doi.org/10.7717/peerj-cs.555 doi: 10.7717/peerj-cs.555
![]() |
[42] | K. He, X. Zhang, S. Ren, J. Sun, Deep residual learning for image recognition, in Proceedings of the IEEE Conference on Computer Vision and Pattern Recognition, IEEE, (2016), 770–778. |
[43] |
L. Wen, X. Li, L. Gao, A transfer convolutional neural network for fault diagnosis based on ResNet-50, Neural Comput. Appl., 32 (2020), 6111–6124. https://doi.org/10.1007/s00521-019-04097-w doi: 10.1007/s00521-019-04097-w
![]() |
[44] |
J. Yang, J. Yu, C. Huang, Adaptive multistrategy ensemble particle swarm optimization with Signal-to-Noise ratio distance metric, Inf. Sci., 612 (2022), 1066–1094. https://doi.org/10.1016/j.ins.2022.07.165 doi: 10.1016/j.ins.2022.07.165
![]() |
[45] |
D. Zhu, Z. Huang, S. Liao, C. Zhou, S. Yan, G. Chen, Improved bare bones particle swarm optimization for DNA sequence design, IEEE Trans. Nanobiosci., 22 (2022), 603–613. https://doi.org/10.1109/TNB.2022.3220795 doi: 10.1109/TNB.2022.3220795
![]() |
[46] |
H. T. Kahraman, S. Aras, E. Gedikli, Fitness-distance balance (FDB): A new selection method for meta-heuristic search algorithms, Knowledge-Based Syst., 190 (2020), 105169. https://doi.org/10.1016/j.knosys.2019.105169 doi: 10.1016/j.knosys.2019.105169
![]() |
[47] |
R. Zheng, A. G. Hussien, R. Qaddoura, H. Jia, L. Abualigah, S. Wang, A multi-strategy enhanced African vultures optimization algorithm for global optimization problems, J. Comput. Des. Eng., 10 (2023), 329–356. https://doi.org/10.1093/jcde/qwac135 doi: 10.1093/jcde/qwac135
![]() |
[48] |
D. Zhu, S. Wang, C. Zhou, S. Yan, Manta ray foraging optimization based on mechanics game and progressive learning for multiple optimization problems, Appl. Soft Comput., 145 (2023), 110561. https://doi.org/10.1016/j.asoc.2023.110561 doi: 10.1016/j.asoc.2023.110561
![]() |
[49] |
R. Murugan, T. Goel, S. Mirjalili, D. K. Chakrabartty, WOANet: Whale optimized deep neural network for the classification of COVID-19 from radiography images, Biocybern. Biomed. Eng., 41 (2021), 1702–1718. https://doi.org/10.1016/j.bbe.2021.10.004 doi: 10.1016/j.bbe.2021.10.004
![]() |
[50] | C. Szegedy, W. Liu, Y. Jia, Going deeper with convolutions, in Proceedings of the IEEE Conference on Computer Vision and Pattern Recognition, IEEE, (2015), 1–9. |
[51] | K. Simonyan, A. Zisserman, Very deep convolutional networks for large-scale image recognition, preprint, arXiv: 1409.1556. |
[52] |
L. Kong, J. Cheng, Classification and detection of COVID-19 X-Ray images based on DenseNet and VGG16 feature fusion, Biomed. Signal Process. Control, 77 (2022), 103772. https://doi.org/10.1016/j.bspc.2022.103772 doi: 10.1016/j.bspc.2022.103772
![]() |
[53] |
A. Karacı, VGGCOV19-NET: Automatic detection of COVID-19 cases from X-ray images using modified VGG19 CNN architecture and YOLO algorithm, Neural Comput. Appl., 34 (2022), 8253–8274. https://doi.org/10.1007/s00521-022-06918-x doi: 10.1007/s00521-022-06918-x
![]() |
[54] |
A. Krizhevsky, I. Sutskever, G. E. Hinton, Imagenet classification with deep convolutional neural networks, Commun. ACM, 60 (2017), 84–90. https://doi.org/10.1145/3065386 doi: 10.1145/3065386
![]() |
[55] |
G. Muhammad, M. S. Hossain, COVID-19 and non-COVID-19 classification using multi-layers fusion from lung ultrasound images, Inf. Fusion, 72 (2021), 80–88. https://doi.org/10.1016/j.inffus.2021.02.013 doi: 10.1016/j.inffus.2021.02.013
![]() |
[56] | I. Bankman, Handbook of Medical Image Processing and Analysis, Elsevier, 2008. |
[57] |
Y. Song, S. Zheng, L. Li, X. Zhang, X. Zhang, Z. Huang, et al., Deep learning enables accurate diagnosis of novel coronavirus (COVID-19) with CT images, IEEE/ACM Trans. Comput. Biol. Bioinf., 18 (2021), 2775–2780. https://doi.org/10.1109/TCBB.2021.3065361 doi: 10.1109/TCBB.2021.3065361
![]() |
[58] |
Y. Pathak, P. K. Shukla, K. V. Arya, Deep bidirectional classification model for COVID-19 disease infected patients, IEEE/ACM Trans. Comput. Biol. Bioinf., 18 (2020), 1234–1241. https://doi.org/10.1109/TCBB.2020.3009859 doi: 10.1109/TCBB.2020.3009859
![]() |
[59] |
Y. Pathak, P. K. Shukla, A. Tiwari, S. Stalin, S. Singh, P. K. Shukla, Deep transfer learning based classification model for COVID-19 disease, IRBM, 43 (2022), 87–92. https://doi.org/10.1016/j.irbm.2020.05.003 doi: 10.1016/j.irbm.2020.05.003
![]() |
[60] |
X. Fan, X. Feng, Y. Dong, H. Hou, COVID-19 CT image recognition algorithm based on transformer and CNN, Displays, 72 (2022), 102150. https://doi.org/10.1016/j.displa.2022.102150 doi: 10.1016/j.displa.2022.102150
![]() |
[61] |
A. S. Ebenezer, S. D. Kanmani, M. Sivakumar, S. J. Priya, Effect of image transformation on EfficientNet model for COVID-19 CT image classification, Mater. Today Proc., 51 (2022), 2512–2519. https://doi.org/10.1016/j.matpr.2021.12.121 doi: 10.1016/j.matpr.2021.12.121
![]() |
[62] |
N. S. Shaik, T. K. Cherukuri, Transfer learning based novel ensemble classifier for COVID-19 detection from chest CT-scans, Comput. Biol. Med., 141 (2022), 105127. https://doi.org/10.1016/j.compbiomed.2021.105127 doi: 10.1016/j.compbiomed.2021.105127
![]() |
Method | Best | Mean | Worst | P |
EDO | 90.63% | 90.23% | 89.73% | 0.01% |
IEDO | 94.42% | 93.08% | 91.52% | - |
Algorithms | Inception | Vgg16 | Vgg19 | Alexnet | Resnet | IEDO-net |
Accuracy | 73.21% | 53.13% | 53.13% | 67.41% | 87.95% | 94.42% |
Algorithms | Parameters |
PSO | c1=c2=2;ω=0.729 |
DE | F=0.5;CR=0.3 |
DEPSO | Iω=0.9;a1=a2=2.05;Fmin=0.5;Fmax=1;CRmax=1.0;CRmin=0.8;α=0.15 |
Method | Accuracy | Sensitivity | Specificity | Precision | F1 score |
PSO | 86.61% | 89.47% | 84.50% | 80.95% | 85.00 |
WOA | 91.07% | 92.08% | 90.24% | 88.57% | 90.29% |
MRFO | 87.95% | 86.79% | 88.98% | 87.62% | 87.20% |
DE | 83.93% | 83.50% | 84.30% | 81.90% | 82.69% |
EDO | 89.73% | 85.96% | 93.64% | 93.33% | 89.50% |
GWO | 89.73% | 91.00% | 88.71% | 86.67% | 88.78% |
DEPSO [25] | 91.52% | 89.81% | 93.10% | 92.38% | 91.08% |
WOABAT [30] | 90.18% | 91.92% | 88.80% | 86.67% | 89.22% |
ASSOA [29] | 91.07% | 92.08% | 90.24% | 88.57% | 90.29% |
EDO-1 | 91.52% | 93.88% | 89.68% | 87.62% | 90.64% |
EDO-2 | 91.96% | 89.91% | 93.91% | 93.33% | 91.59% |
IEDO | 94.42% | 93.40% | 94.92% | 94.29% | 93.84% |
Method | No. of images | Accuracy | Sensitivity | Specificity | Precision | F1 score |
DRE-Net | 1990 | 93.00% | 93.00% | 93.00% | 93.00% | 93.00% |
MADE-DBM | 1790 | 96.20% | 96.23% | 96.17% | - | 96.17% |
DTL | 852 | 93.02% | 91.46% | 94.78% | 95.19% | 93.29% |
Trans-CNN Net | 194922 | 96.73% | 97.76% | 96.01% | 97.45% | 96.36% |
CLAHE transform | 2482 | 94.56% | 91.00% | - | 95.00% | 93.00% |
8-CLF | 746 | 93.33% | 93.17% | 88.71% | 93.17% | 93.29% |
IEDO-net | 746 | 94.42% | 93.40% | 94.92% | 94.29% | 93.84% |
Method | Best | Mean | Worst | P |
EDO | 90.63% | 90.23% | 89.73% | 0.01% |
IEDO | 94.42% | 93.08% | 91.52% | - |
Algorithms | Inception | Vgg16 | Vgg19 | Alexnet | Resnet | IEDO-net |
Accuracy | 73.21% | 53.13% | 53.13% | 67.41% | 87.95% | 94.42% |
Algorithms | Parameters |
PSO | c1=c2=2;ω=0.729 |
DE | F=0.5;CR=0.3 |
DEPSO | Iω=0.9;a1=a2=2.05;Fmin=0.5;Fmax=1;CRmax=1.0;CRmin=0.8;α=0.15 |
Method | Accuracy | Sensitivity | Specificity | Precision | F1 score |
PSO | 86.61% | 89.47% | 84.50% | 80.95% | 85.00 |
WOA | 91.07% | 92.08% | 90.24% | 88.57% | 90.29% |
MRFO | 87.95% | 86.79% | 88.98% | 87.62% | 87.20% |
DE | 83.93% | 83.50% | 84.30% | 81.90% | 82.69% |
EDO | 89.73% | 85.96% | 93.64% | 93.33% | 89.50% |
GWO | 89.73% | 91.00% | 88.71% | 86.67% | 88.78% |
DEPSO [25] | 91.52% | 89.81% | 93.10% | 92.38% | 91.08% |
WOABAT [30] | 90.18% | 91.92% | 88.80% | 86.67% | 89.22% |
ASSOA [29] | 91.07% | 92.08% | 90.24% | 88.57% | 90.29% |
EDO-1 | 91.52% | 93.88% | 89.68% | 87.62% | 90.64% |
EDO-2 | 91.96% | 89.91% | 93.91% | 93.33% | 91.59% |
IEDO | 94.42% | 93.40% | 94.92% | 94.29% | 93.84% |
Method | No. of images | Accuracy | Sensitivity | Specificity | Precision | F1 score |
DRE-Net | 1990 | 93.00% | 93.00% | 93.00% | 93.00% | 93.00% |
MADE-DBM | 1790 | 96.20% | 96.23% | 96.17% | - | 96.17% |
DTL | 852 | 93.02% | 91.46% | 94.78% | 95.19% | 93.29% |
Trans-CNN Net | 194922 | 96.73% | 97.76% | 96.01% | 97.45% | 96.36% |
CLAHE transform | 2482 | 94.56% | 91.00% | - | 95.00% | 93.00% |
8-CLF | 746 | 93.33% | 93.17% | 88.71% | 93.17% | 93.29% |
IEDO-net | 746 | 94.42% | 93.40% | 94.92% | 94.29% | 93.84% |