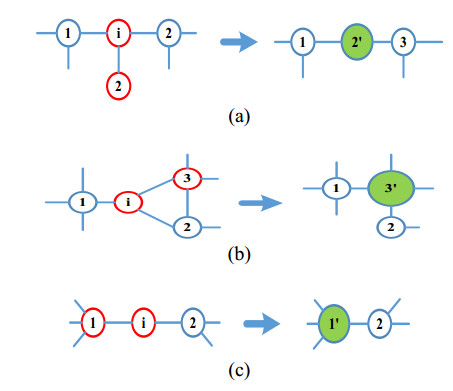
Citation: Abdelazeem A. Abdelsalam, Karim M. Hassanin, Almoataz Y. Abdelaziz, Hassan Haes Alhelou. Optimal PMUs placement considering ZIBs and single line and PMUs outages[J]. AIMS Energy, 2020, 8(1): 122-141. doi: 10.3934/energy.2020.1.122
[1] | Amandeep Gill, Pushpendra Singh, Jalpa H. Jobanputra, Mohan Lal Kolhe . Placement analysis of combined renewable and conventional distributed energy resources within a radial distribution network. AIMS Energy, 2022, 10(6): 1216-1229. doi: 10.3934/energy.2022057 |
[2] | Stefania Conti, Santi A. Rizzo, Nunzio Salerno, Giuseppe M. Tina . Distribution network topology identification based on synchrophasor. AIMS Energy, 2018, 6(2): 245-260. doi: 10.3934/energy.2018.2.245 |
[3] | Mikaeel Ahmadi, Mir Sayed Shah Danish, Mohammed Elsayed Lotfy, Atsushi Yona, Ying-Yi Hong, Tomonobu Senjyu . Multi-objective time-variant optimum automatic and fixed type of capacitor bank allocation considering minimization of switching steps. AIMS Energy, 2019, 7(6): 792-818. doi: 10.3934/energy.2019.6.792 |
[4] | Kunzhan Li, Fengyong Li, Baonan Wang, Meijing Shan . False data injection attack sample generation using an adversarial attention-diffusion model in smart grids. AIMS Energy, 2024, 12(6): 1271-1293. doi: 10.3934/energy.2024058 |
[5] | Habibullah Fedayi, Mikaeel Ahmadi, Abdul Basir Faiq, Naomitsu Urasaki, Tomonobu Senjyu . BESS based voltage stability improvement enhancing the optimal control of real and reactive power compensation. AIMS Energy, 2022, 10(3): 535-552. doi: 10.3934/energy.2022027 |
[6] | Gerardo Guerra, Juan A. Martinez-Velasco . Optimal sizing and operation of energy storage systems considering long term assessment. AIMS Energy, 2018, 6(1): 70-96. doi: 10.3934/energy.2018.1.70 |
[7] | Awatif Nadia, Abdul Hasib Chowdhury, Esheta Mahfuj, Md. Sanwar Hossain, Khondoker Ziaul Islam, Md. Istianatur Rahman . Determination of transmission reliability margin using AC load flow. AIMS Energy, 2020, 8(4): 701-720. doi: 10.3934/energy.2020.4.701 |
[8] | Pooja Khandare, Sanjay Deokar, Arati Dixit . Relay Coordination and Optimization techniques using DWT-Differentiation Algorithms for Fault Detection in Microgrid. AIMS Energy, 2020, 8(4): 563-579. doi: 10.3934/energy.2020.4.563 |
[9] | Minh Y Nguyen . Optimal voltage controls of distribution systems with OLTC and shunt capacitors by modified particle swarm optimization: A case study. AIMS Energy, 2019, 7(6): 883-900. doi: 10.3934/energy.2019.6.883 |
[10] | Katherin Indriawati, Bambang L. Widjiantoro, Ali Musyafa . Design of sensor and actuator fault tolerant control system on wind turbine benchmark for Region II. AIMS Energy, 2019, 7(2): 111-126. doi: 10.3934/energy.2019.2.111 |
Phasor measurement units (PMUs) based on the global positioning system (GPS) technique are considered to be key elements which are widely used in monitoring, controlling and state estimation of electrical power systems. PMU provides electrical power engineers with precise and immediate information about system states, as its measurements are being on the same datum of time that is defined by GPS. Stability and reliability of power system could be improved by applying synchronized measuring of phasors in several regions of electric power system like control, protection, estimation of system states and voltage fall downs [1]. It is unattainable to install PMU at all power system buses because of high cost and connection complexity. Hence, optimal PMUs placement (OPP) problem is required to be solved for better saving and full system observability. So, the capability of PMU to monitor the voltage of system bus and current phasors of its incident lines, requires to locate PMUs at the most appropriate buses to make the system full observable by using less PMUs. Full observability is obtained by monitoring the buses voltage and lines current of the system [2].
Recently, many different methods have been presented to identify the solution of PMUs allocations problem. Binary Integer Programming method is presented in [3] for PMUs allocation and flow measurements of faults in electrical power systems. Initially, the nonlinear programming problem formulation was unsolvable. So, its initial formulation results were transformed through Boolean implications to a corresponding integer-linear programming (ILP) problem. This transformation provided a high range of scalability. A modified version of binary cuckoo optimization algorithm (BCOA) was introduced in [4] to identify the solution of OPP problem. The algorithm structure is mainly based on the lifestyle as well as egg laying of the cuckoo bird. Major features of modified binary cuckoo optimization algorithm (MBCOA) were its capability to obtain the optimum solution and its fast convergence. All stages of cuckoo optimization algorithm (COA) consists of initial population generation, egg number, radius of egg laying and also immigration technique were rearranged in MBCOA to consider the binary form of OPP problem. In [5], authors considered hidden critical cost while presenting PMUs placement. In [6], authors presented Depth First Search (DFS), Simulated Annealing (SA) and minimum spanning tree methods to solve OPP problem at normal operation condition also under contingencies. In [7] the researchers proposed a binary search algorithm for system observability, that is able to find the global optimum solution, but it is computationally expensive. Furthermore, integer programming was used for problem of PMUs placement [8,9,10,11], where in [8], authors proposed integer quadratic programming, considering loss of PMUs and single line outages. However, the effect of zero-injection buses is not considered in the developed algorithm. The researchers in [9] used a generalized integer linear programming. The method considered power systems with and without the existence of conventional flow measurement; nevertheless, some modifications were done to use it in multi-stage planning. Furthermore, it did not study the contingencies. In [10], the authors have formulated the multi stage PMUs allocation using ILP. The failure of single PMU is incorporated while the loss of system line outage, is not considered. The authors proposed a binary integer linear programming (BILP) in [11] with two objectives, which are minimum metering economy and power system observability. In [12], the researchers have introduced an algorithm to solve this problem to achieve the system observability and detect the bad data. In [13] the authors have solved OPP problem using a mixed integer nonlinear programming (MINLP) algorithm with variables as binary values variables the observability check is done every time. In [14], the authors have solved the OPP based on the rules of topological observability and the ZIBs are taken into account. In [15], a genetic algorithm (GA) has been proposed to find the OPP. The authors extended the OPP problem formulation and defined new concepts which are maximizing measurements increase and observability assessment, for normal condition and contingencies. Moreover, the pre-allocation of PMUs is taken into account. The fuzzy algorithm is presented to find the solution of OPP problem with taking into account the lower number of PMU channels for achieving network full observability [16], this algorithm is used with normal operating conditions and system contingencies. The recursive tabu search (RTS) is a combination of numerical method and tabu search (TS) has been introduced in [17] to obtain a full observable power system with maximum measurement redundancy. A technique introduced in [18] for PMUs placement in multiple stages ensured complete system observability under a line loss or PMU failure. The proposed method is based on ILP and multi-criteria decision-making (MCDM) approach. The number of PMUs is identified by ILP for the complete observable system while MCDM has been used to prioritize the optimal locations of PMUs. A novel approach for state estimation of power system using optimal PMUs placement, is proposed in [19]. The optimal PMUs placement for full power system observability has been achieved using improved particle swarm optimization (IPSO). This paper has introduced an optimization technique for the state estimation measurements using the weighted least square (WLS) method. Also, it has proposed a numerical method to check the complete system observability that has been fulfilled. In [20,21], the authors have presented a flexible and simple, semi-definite programming (SDP) method, which minimizes installed PMUs number, ensures full observability of the power system with considering the availability of traditional measurements. The optimal solution is gotten by the minimization of the objective function with using the decision variables in binary form, subjected to inequality constraint that represents the observability.
In this paper, a modern optimization method which is a flower pollination algorithm (FPA), is adopted to find optimal PMUs placement (OPP) in which its robustness and efficiency as an optimization method is proven. The FPA is utilized to obtain the minimum number of PMUs needed to make the power system completely observable and increase measurements redundancy. The OPP problem is formulated to consider the practical and technical issues in reality. The proposed method has been tested with various cases that are normal case, loss of PMUs, single line outage and considering the existence of zero-injection buses (ZIBs) which helps in reducing the number of PMUs needed to verify the complete observability of the power system. Efficiency, robustness and performance of the proposed method have been practiced on IEEE 14-bus, 30-bus, 39-bus, 57-bus, and 118-bus test systems. The results verify the applicability of the proposed method to solve OPP problem in modern power systems considering the most severe technical issues in reality. The main novel, contributions of this paper are:
ⅰ)Proposing FPA for solving practical optimal phasor measurement unit placement problem.
ⅱ)The proposed method is applicable to large scale power systems with different topologies.
ⅲ)The proposed method considers all contingency issues such as loss of PMUs and line outages.
The rest of the paper is arranged as: section 2 introduces observability analysis and PMUs placement rules at various conditions. The problem formulation of PMUs placement and modeling of ZIB in the power system are discussed in section 3. The suggested optimization method (FPA) is explained briefly in section 4. Section 5 discusses different test cases of PMUs placement and their simulation results for different test system used. Finally, the conclusions of this work are provided in section 6.
The main reason of optimal allocation of PMUs in electrical power systems is the observability of the power system which means that all voltage phasors of system buses and all current phasors of system lines are known. Whatever the used method, the power system observability should be checked each time PMU is placed. If the system is observable, the placement process stops, else the process must be continued.
There are two methods for system observability analysis, topological observability and numerical observability. In the topological observability method, the decision is based on logical operation. So, some information about network topology and connection and measurement types with their locations are essential. The power system is observable when the spanning tree can be constructed and full ranked by current measurements set. It uses a graph theory and decoupled measurement model. Numerical observability is based on the numerical factorization of the gain matrix or measurement Jacobian of measurements information. The system is observable when at least one of the matrices has full rank. It uses either decoupled or fully coupled measurement models.
Z=Hx+V | (1) |
where:
Z: a column vector of length m,
H: Jacobian matrix with size m × (2N – 1),
x: a column vector of length (2N – 1),
V: a column vector of length m and represents the measurement noise,
N: represents the buses number,
m: represents the measurement samples,
In large systems, these matrices are complicated so the numerical algorithms are not appropriate.
The full observability of a system is achieved if all system buses are observable. A bus is observable when its voltage phasor is directly or indirectly measured.
In this work, the topological observability method is used to help in solving the PMUs placement according to following rules.
○ The bus with an installed PMU has its voltage and the current phasors of all its incident branches measured by PMU. This is called direct measurement.
○ For the buses which are connected to a bus with PMU, as the voltage and current phasors are known at one end, ohm’s law is utilized to find the voltage phasor of that bus. This is called pseudo-measurements.
○ If the voltages of two ends of a branch are known, ohm’s law is utilized to find the current between these two ends
A zero-injection bus (ZIB) is another factor which can help in reducing the number of required PMUs to make the power system fully observed. ZIB is a bus that has neither power generator to inject power in it, nor loads to consume power from it. Hence, the sum of all current phasors of its incident branches equals zero. Power system observability will be assessed with the existence of ZIBs using the below rules:
● When an observable ZIB has observable adjacent buses except one, the Kirchhoff’s current law (KCL) and Kirchhoff’s voltage law (KVL) can be applied at ZIB to make this bus observed.
● When ZIB is not observable and it has an adjacent observable buses, KCL and KVL can be applied to make ZIB observable.
● Finally, the node voltage equation is used to make group of ZIBs to be observable if they have adjacent observable buses.
The objective of solving the OPP problem is to find the minimum number of PMUs needed and their locations for achieving full observability of the power system. Thus, this objective function is formulated as follows:
Minimize∑Ni=1xi | (2) |
Subjectto:AX≥b | (3) |
where
N: number of buses,
X: the decision vector with binary values,
xi: an element in X and it show the feasibility of installing PMU at the ith bus as:
xi={1ifaPMUisinstalledatithbus0otherwise | (4) |
A is the binary bus connectivity matrix, and Eq 5 is used to define its entries.
Ai,j={1ifi=j1ifbusiisconnectedtobusj0otherwise | (5) |
where AX is a set of constraints that show the bus observability where its element is one if the bus is observable and otherwise is zero and b is a vector filled with ones which ensures the observability of the system and is given by:
b=[111…1]TN×1 | (6) |
There is another factor that is very useful because with increasing it, the bus remains observed if one of measurements lost. This factor is called the measurement redundancy and is defined as how many times the bus is observed by direct or indirect measurements. During optimization process to fulfil the objective function, the results give multiple solutions with multiple redundancy measurements for each solution. The decision is to choose the optimal solution that fulfils the objective function and with maximum redundancy. There is a term called redundancy index (R. index) that is used to identify the measurement redundancy. R. index is defined as sum of all buses observability as follows
R.index=sum(AX) | (7) |
The proposed method includes the action of merging ZIB with its incident bus. This merging action helps in reducing system buses by one, and this will help in decreasing PMUs number that are required to fulfil the complete observability of power system states. Moreover, the merging process causes modifications in the network topology of the power system and it needs to redefine the network equations to reflect that changes.
The results obtained by using the merging process change for each bus available to be merged with ZIB. The following are rules of the merging process:
○ Merge ZIB with its incident radial bus, Figure 1(a) shows the merging between ZIB which is incident to radial bus.
○ The adjacent bus of ZIB which has the greatest number of connected buses is merged with ZIB as shown in Figure 1(b).
○ ZIB is merged with neighbor bus of the greatest number of connected buses. Figure 1(c) shows this rule.
Figure 2 shows a sample network, which is part of a larger system. In this network, the buses 1, 4, 5 and 6 are observable by indirectly method. Buses 2 and 3 are a group of adjacent zero injected buses and their phasors can be computed using KVL and KCL equations as follows:
V1−V2Z12+V5−V2Z52+V4−V3Z43+V6−V3Z63=0 | (8) |
V2=V3−(V4−V3Z43+V6−V3Z63)Z23 | (9) |
Using Eqs 8 and 9, the voltages V2 and V3 can be computed if the voltages V1, V4, V5 and V6 are known.
The flower pollination algorithm (FPA) is a meta-heuristic search algorithm that was developed recently by Xin-She Yang and Deb [22,23,24,25]. It is inspired by the sexual reproduction of flowering plants through pollination process. For simplicity, the four rules are used as follows. First rule: global pollination is represented in cross- pollination and biotic pollination and motion of pollinators obeys Le´vy flights. Second rule: a combination of self-pollination and abiotic pollination represents local pollination. The process does not need any pollinators. Third rule: pollinators improve flower stability. Flower stability is equal to reproduction probability. This probability depends on the resemblance of two involved flowers. Rule 4: switching between local pollination and global pollination is achieved by changing the probability switch, p ∈[0, 1].
The previous rules should be formulated into suitable equations. Thus, in the global pollination rule, pollinators load flower pollen gamete. So, the pollen is moving over long distances. Subsequently, the global pollination with flower stability rules is formulated as:
Xt+1i=Xti+γL(g∗−xti) | (10) |
where Xit is a vector represents the pollen i or the solution xi at the t iteration and g* is the optimal solution found among all solutions at the current generation/iteration.
L is a Lévy flights based step size which correspond to the pollination strength. As long distance can be wrapped through many distance jumps, A Lévy flight is utilized to simulate efficiently the characteristic. Where, L > 0 is taken from a Lévy function.
L∼λΓ(λ)sin(πλ/2)π∗1s1+λ,(S≫S0>0) | (11) |
where γ is a rescaling coefficient, Γ(λ) is gamma function, and this distribution is suitable for large steps S > 0. S0 is the initial step size. Two Gaussian distributions U and V are used to draw the step size S by using Mantegna algorithm by the following transformation:
S=U|V|1/λ | (12) |
U∼N(0,σ2),V∼N(0,1) | (13) |
U~N(0, σ2) denotes that Gaussian normal distribution is used to draw the samples that have zero mean and their variance is σ2.
The equation of variance calculation is:
![]() |
(14) |
To find local pollination, second and third rules are used to formulate the following:
Xt+1i=Xti+ε(xtj−xtk) | (15) |
where xjt and xkt are pollens of various flowers that have the same plant types and ε is selected from the uniform distributed range [0, 1].
Flower Pollination Algorithm (FPA) is improved by the concept of sexual reproduction of flowering plants via pollination process. In this algorithm, two parameters, population dimension n, and probability coefficient p∈[0, 1] are used to improve the performance of FPA. The moment that n is fixed, p can essentially controls the balance of randomization, pollinators and the local search. Hence, few parameters make the algorithm potentially more generic and less complex. In this paper, the population size n = 20, rescaling coefficient γ = 1.5, the probability p = 0.8 [22] and the maximum iteration = 500. The flowchart of the proposed FPA is shown in Figure 3. For each generated solution, before applying this solution, the constraint expressed in Eq 3 must be verified. If this solution does not verify the constraint, this solution is escaped and another solution is generated and this is explained in the following flow chart.
The performance of the proposed method is assessed by applying it on different standard networks such as IEEE 14-bus, IEEE 30-bus, IEEE 57-bus, IEEE 118-bus [25], and the New England 39-bus networks [26] to find the optimal solution of OPP. Figures 4 depicts single line diagrams of these standard networks. Table 1 shows the number and locations of the zero-injection buses and radial buses for each test network. All the test networks contain two types of synchronous machines; synchronous generators and synchronous motors work as synchronous condensers. Where 14-bus network contains two synchronous generators and three synchronous condensers and its single line diagram is shown in Figure 4(a). 30-bus network contains two generators and four synchronous condensers used for supporting reactive power, and the other two are used for generation and its single line diagram is shown in Figure 4(c). the 57-bus system has four generators and three synchronous condensers and its single line diagram is shown in Figure 4(b). NE 39-bus system has 10 synchronous machines which are generators. Its single line diagram is shown in Figure 4(d). IEEE 118-bus system consists of 54 synchronous machines, 20 of which are synchronous compensators used only for reactive power support and 15 of which are motors, and the other 19 are generators where its single line diagram is given in [26].
Test systems | Zero injection buses | Radial buses | ||
No. | Locations | No. | Locations | |
14-bus | 1 | 7 | 1 | 8 |
30-bus | 7 | 6, 9, 22, 25, 27, 28 | 3 | 11, 13, 26 |
NE 39-bus | 12 | 1, 2, 5, 6, 9, 10, 11, 13, 14, 17, 19, 22 | 9 | 30, 31, 32, 33, 34, 35, 36, 37, 38 |
57-bus | 15 | 4, 7, 11, 21, 22, 24, 26, 34, 36, 37, 39, 40, 45, 46, 48 | 1 | 33 |
118-bus | 10 | 5, 9, 30, 37, 38, 63, 64, 68, 71, 81 | 7 | 10, 73, 87,111,112,116,117 |
The performance of FPA is tested by four test cases. These cases are conducted in details on 14-bus IEEE standard network that is shown in Figure 4(a) the explanation of these test cases are:
In this case, the objective is to achieve full system observability without considering the existence of ZIB and without losing any PMUs. By using Eq 5, the binary bus connectivity matrix is created for the IEEE 14-bus as shown in (16). The inequality constraints of the A-matrix are formulated as shown in (17).
[A]=[110010000000011111000000000011100000000000111101010000011011100000000000011000011100001001110000000000011000000000100101100010000000011100000000100011000000001000001100000010000011100000000100011] | (16) |
(x)={f1=x1+x2+x5≥1(a)f2=x1+x2+x3+x4+x5≥1(b)f3=x2+x3+x4≥1(c)f4=x2+x3+x4+x5+x7+x9≥1(d)f5=x1+x2+x4+x5+x6≥1(e)f6=x5+x6+x11+x12+x13≥1(f)f7=x4+x7+x8+x9≥1(g)f8=x7+x8≥1(h)f9=x4+x7+x9+x10+x14≥1(i)f10=x9+x10+x11≥1(j)f11=x6+x10+x11≥1(k)f12=x6+x12+x13≥1(l)f13=x6+x12+x13+x14≥1(m)f14=x9+x13+x14≥1(n) | (17) |
These constraints mean that according to Eq 17e, installing PMU at bus 5, buses 1, 2, 4, 5 and 6 will be observable.
Based on Figure 4a, bus 7 and bus 8 are ZIB and radial bus, respectively. According to the first rule of merging process of ZIBs, bus 8 will be merged with bus 7. This process leads to removing the constraint of bus 7 and then connect bus 8 with buses 4 and 9 directly. Then the constraints of buses 4, 8 and 9 are updated to reflect the merging process and reformulated as follows:
f(x)={f4=x2+x3+x4+x5+x8+x9≥1(a)f8′=x4+x8′+x9≥1(b)f9=x4+x8′+x9+x10+x14≥1(c) | (18) |
The merging process is shown below in Figure 5.
In order to improve the power system reliability and ensure system complete observability under failure of PMU, each bus in the power system must be observed by at least two PMUs. This is reflected in the constraints by multiplying Eq 6 right term by 2 to find Eq 19.
Then, we have
b=[222…….2]TN×1 | (19) |
It is a common fault in the power systems. If a bus is being observed by two PMUs, then a related line loss will not affect the complete observability of the power system. This can be achieved by constraints that are given in Eq 19. In this case, every radial bus has only one incident branch. So when losing that incident branch, that radial bus will be unobservable. Wherefore, these radial buses should be excluded from the system as well as those inequality constraints. As it is impossible to observe it through two lines.
The effectiveness and performance of the proposed optimization method is assessed by applying it on the IEEE 14-bus, IEEE 30-bus, IEEE 57-bus, IEEE 18-bus and NE 39-bus test systems under the previous three case studies.
The results obtained for the normal case without considering neither ZIBs nor contingencies are shown in Table 2. As noticed from shown results, the FPA obtained many PMUs locations’ sets for the same number of PMUs. That proves the ability of the proposed FPA to give variety of solutions of minimum PMUs number.
Test System | Normal Case | ||
No. of PMUs | Locations sets | R. Index | |
14-bus | 4 | 2, 6, 7, 9 | 19 |
2, 6, 8, 9 | 17 | ||
30-bus | 10 | 2, 4, 6, 9, 10, 12, 15, 18, 25, 27 | 52 |
2, 4, 6, 9, 10, 12, 19, 24, 25, 27 | 51 | ||
NE 39-bus | 13 | 2, 6, 9, 10, 13, 14, 17, 19, 20, 22, 23, 25, 29 | 52 |
2, 6, 9, 10, 11, 14, 17, 19, 22, 23, 25, 29, 34 | 51 | ||
57-bus | 17 | 1, 4, 6, 9, 15, 20, 24, 28, 31, 32, 36, 38, 41, 47, 51, 53, 57 | 72 |
1, 4, 7, 9, 15, 20, 24, 28, 31, 32, 36, 38, 41, 46, 50, 53, 57 | 71 | ||
118-bus | 32 | 1, 5, 9, 11, 12, 17, 21, 23, 28, 30, 34, 37, 42, 45, 49, 53, 56, 62, 64, 68, 71, 75, 77, 80, 85, 87, 91, 94,101,105,110,115 | 160 |
3, 5, 10, 12, 13, 17, 21, 24, 25, 28, 34, 37, 40, 45, 49, 53, 56, 62, 63, 68, 71, 75, 77, 80, 85, 86, 91, 94,101,105,110,114 | 157 |
The results obtained for the second case which includes considering ZIBs are shown in Table 3. As depicted in Table 3, it is obvious that considering ZIBs helps in decreasing the number of PMUs that is required to fulfil complete system observability. It saves PMUs and in turn saves money as the overall cost will be decreased because of decreasing PMUs number and their needed connections. For example, it saves a PMU for 14-bus network, three PMUs for 30-bus network, five PMUs for 57-bus network and NE 39-bus network.
Test systems | Considering ZIB | ||
No. PMUs | Locations sets | R. Index | |
14-bus | 3 | 2, 6, 9 | 15 |
30-bus | 7 | 1, 7, 10, 12, 18, 24, 29 | 29 |
3, 5, 10, 12, 18, 24, 29 | 29 | ||
NE 39-bus | 8 | 3, 8, 12, 16, 20, 23, 25, 29 | 32 |
57-bus | 12 | 1, 6, 9, 14, 19, 25, 29, 32, 38, 41, 50, 53 | 52 |
2, 6, 12, 14, 19, 25, 29, 32, 38, 41, 51, 54 | 49 | ||
118-bus | 29 | 2, 8, 11, 12, 17, 20, 23, 28, 33, 34, 40, 45, 49, 53, 56, 61, 66, 72, 75, 77, 80, 85, 86, 91, 94,102,105,110,114 | 143 |
The results obtained for the third case that includes considering a loss of PMUs are shown in Table 4. It is obvious that more benefits need more cost. So, to improve the system reliability, the PMUs number should be increased which in turn increases cost. In that case, the PMUs number depends on the radial buses and the buses that have only two incident buses. The more these buses in the system, the more PMUs needed. And that is depicted in Table 4, the number of PMUs is increased by 5 for 14-bus network, 11 for 30-bus network, 16 for 57-bus network and NE 39-bus network, and finally increased by 37 for 118-bus network.
Test systems | Loss of PMU | ||
No. of PMUs | Locations sets | R. Index | |
14-bus | 9 | 2, 4, 5, 6, 7, 8, 9, 11, 13 | 39 |
1, 2, 4, 6, 7, 8, 9, 11, 13 | 37 | ||
30-bus | 21 | 1, 2, 3, 5, 6, 9, 10, 11, 12, 13, 15, 17, 18, 20, 22, 24, 25, 26, 27, 28, 29 | 83 |
2, 3, 4, 6, 7, 8, 9, 10, 11, 12, 13, 15, 17, 19, 20, 21, 23, 25, 26, 27, 30 | 82 | ||
NE 39-bus | 29 | 2, 3, 4, 6, 8, 9, 10, 11, 12, 14, 16, 17, 19, 20, 22, 23, 25, 26, 29, 30, 31, 32, 33, 34, 35, 36, 37, 38, 39 | 99 |
57-bus | 33 | 1, 3, 4, 6, 9, 11, 12, 15, 19, 20, 22, 24, 25, 27, 28, 29, 30, 32, 33, 35, 36, 38, 39, 41, 45, 46, 47, 50, 51, 53, 54, 56, 57 | 129 |
118-bus | 69 | 1, 3, 5, 7, 9, 10, 11, 12, 15, 17, 19, 21, 22, 24, 25, 27, 29, 30, 31, 32, 34, 36, 37, 40, 42, 44, 45, 46, 49, 51, 52, 54, 56, 57, 59, 62, 64, 65, 66, 68, 70, 71, 73, 75, 76, 77, 79, 80, 82, 83, 85, 86, 87, 89, 91, 92, 94, 96,100,102,105,106,109,110,111,112,114,116,117 | 313 |
The optimal number in case of considering the failure of a single line between two buses is depicted in Table 5.
Test systems | Single Line Outage | ||
No. of PMUs | Locations sets | R. Index | |
IEEE 14-bus | 7 | 2, 4, 5, 6, 9, 10, 13 | 33 |
IEEE 30-bus | 16 | 1, 2, 4, 5, 6, 10, 12, 15, 16, 18, 19, 22, 24, 27, 28, 30 | 71 |
NE 39-bus | 15 | 2, 3, 6, 8, 9, 11, 13, 14, 16, 17, 22, 23, 26, 29, 39 | 63 |
1, 2, 3, 6, 8, 9, 11, 13, 14, 16, 17, 22, 23, 26, 29 | 63 | ||
IEEE 57-bus | 32 | 1, 2, 4, 6, 9, 11, 12, 15, 19, 20, 22, 24, 25, 27, 28, 29, 30, 32, 34, 36, 37, 38, 39, 41, 45, 46, 47, 50, 51, 53, 54, 56 | 127 |
IEEE 118-bus | 61 | 1, 3, 5, 7, 8, 11, 12, 15, 17, 19, 21, 22, 24, 25, 27, 29, 30, 31, 32, 34, 36, 37, 40, 42, 44, 45, 46, 49, 51, 53, 54, 56, 57, 59, 62, 64, 65, 66, 68, 70, 71, 75, 76, 77, 79, 80, 82, 83, 85, 89, 91, 92, 94, 96,100,102,105,106,109,110,114 | 297 |
To prove that the suggested FPA has a higher performance in solving the OPP, it must be compared with other algorithms that have been used in solving the OPP. Comparison are conducted based on two phases; results and performance. Tables 6–9 depict the comparisons between FPA and other algorithms with respect to the best results. Table 6 depicts a comparison between FPA and other optimization algorithms for solving OPP for normal case. FPA gives the same optimal numbers of PMUs like other methods but with different locations and with greater R. index. Table 7 depicts a comparison between the suggested FPA and other optimization algorithms for solving OPP with considering ZIBs. The results of using FPA are the best in networks 14-bus, 30-bus and NE-39-bus, respectively, while FPA gives the better redundancy R. index in networks 57-bus and 118-bus. Table 8 depicts a comparison between the suggested FPA and other optimization algorithms for solving OPP for normal case with considering loss of PMU. The results of FPA are the same other methods but with greater R. index number in networks 14-bus, 30-bus and 57-bus and it has the gratest R. index in case of NE 39-bus and 118-bus. Finally, the comparison in case of considering the loss of single branch between busses is shown in Table 9 and the results of FPA are the best. From the results depicted in Tables 6-9, it is noticed that the suggested FPA is one of the best algorithms to be used in solving OPP problem as it has the ability to fulfil a complete observability of the system with lower number of needed PMUs and a variety of PMUs locations sets.
Algorithms | IEEE 14-bus | IEEE30-bus | NE39-bus | IEEE57-bus | IEEE118-bus |
Exhaustive Search Algorithm (ES) [7] | 4, R19 | 10, R50 | - | - | - |
Integer Quadratic Programming (IQP) [8] | 4, R19 | 10, R49 | - | 17, R69 | 32, R159 |
Binary Integer Linear Programming (BILP) [27] | 4, R18 | 10, R49 | - | 17, R69 | 32, R158 |
Topology Transformation Method (TT) [28] | 4, R19 | 10, R51 | - | 17, R71 | 32, R160 |
Staged ES [34] | 4, R18 | 10, R50 | 13, R49 | 17, R70 | 32, R159 |
Taguchi binary bat algorithm (TBBA) [37] | 4, R19 | 10, R51 | - | 17, R71 | 32, R160 |
Proposed FPA | 4, R19 | 10, R52 | 13, R52 | 17, R72 | 32, R160 |
Algorithms | 14-bus | 30-bus | NE 39-bus | 57-bus | 118-bus |
ES [7] | 3, R15 | 7, R28 | 8, R30 | - | - |
Integer Linear Programming (ILP) [11] | 3, R14 | 7, R28 | 8, R29 | 11, R50 | 28, R141 |
GA [15] | 3, R15 | 7, R29 | - | 12, R52 | 29, R142 |
TT [28] | 3, R15 | 7, R29 | 8, R31 | 11, R51 | 28, R143 |
ILP [29] | 3, R14 | - | - | 12, R53 | 29, R142 |
Binary Particle Swarm (BPSO) [30] | 3, R15 | 7, R29 | - | 13, R54 | 29, R143 |
Branch and Bound (B & B) [31] | - | 7, R27 | 9, R33 | 12, R53 | 29, R141 |
Differential Evolution (DE) [32] | 3, R15 | 7, R29 | 8, R31 | 11, R50 | 29, R143 |
Improved PSO (IPSO) [33] | - | 7, R29 | 8, R30 | 11, R52 | - |
Staged ES [34] | 3, R15 | 7, R29 | 8, R31 | 11, R49 | 28, R140 |
Proposed FPA | 3, R15 | 7, R29 | 8, R32 | 12, R52 | 29, R143 |
Algorithms | 14-bus | 30-bus | NE 39-bus | 57-bus | 118-bus |
IQP[8] | 9, R37 | 21, R80 | - | 33, R128 | 68, R310 |
Integer Programming (IP) [10] | 9, R39 | 22, R88 | - | 35, R133 | 72, R318 |
Modified Binary PSO (MBPSO) [35] | 9, R39 | 21, R82 | 28, R96 | 33, R129 | 68, R310 |
Binary Semi-Definite Programming (BSDP) [36] | 9, R38 | - | - | - | 72, R318 |
TBBA [37] | 9, R39 | 21, R82 | - | 33, R128 | 68, R312 |
Proposed FPA | 9, R39 | 21, R83 | 29, R99 | 33, R129 | 69, R313 |
Algorithms | 14-bus | 30-bus | NE 39-bus | 57-bus | 18-bus |
IQP [8] | 9, R37 | 21, R80 | - | 33, R128 | 68, R310 |
ILP [38] | 9, R36 | 21, R79 | 28, R97 | 33, R129 | 68, R307 |
MBPSO [39] | 9, R39 | - | - | - | 72, R317 |
Proposed FPA | 7, R33 | 16, R71 | 15, R63 | 32, R127 | 61, R297 |
The second phase of comparison is shown in Figure 6 and Table 10. Figure 6 shows a comparison between FPA, GA [15] and BPSO [30] under normal case for the tested systems. The results show that FPA is the fastest algorithm in reaching to the optimal solution where it reaches to the optimal solution after 6, 10, 7 and 10 iterations for IEEE 14-bus, 30-bus, 57-bus and 118-bus, respectively. Also the computational time based on the used computer is compared for FPA and other method as shown in Table 10. The results show that the FPA has the minimum computational time but with high specifications computer.
Algorithms | Computer specifications | 14-bus | 30-bus | 57-bus | 118-bus |
IQP [8] | Intel Xeon 3.4-GHz CPU with 2 GB RAM | 3.09 | 2.6 | 4.24 | 3.09 |
Integer Linear Programming (ILP) [11] | 2.13-GHz Intel(R) Core(TM) 2 CPU with 2 GB RAM | 0.05 | - | 30.41 | 1625.30 |
ILP [29] | Intel Core2 Duo CPU E7500 running at 2.93-GHz with 2 GB RAM | 0.04 | - | 1.93 | 6.37 |
Differential Evolution (DE) [32] | Intel Xeon 3.4-GHz CPU with 2 GB RAM | 2 | 4 | 2 | 3 |
MBPSO [39] | Centrino 1.6-GHz CPU with 512 MBRAM | 0.052 | 0.071 | 0.106 | 0.169 |
Proposed FPA | Intel Core i7 2.67-GHz CPU with 6-GB RAM | 0.02 | 0.51 | 0.1 | 0.1 |
The results obtained confirms that the proposed optimization method (FPA) can solve the OPP problem to fulfil the complete power system at different case studies such as normal case, then considering of ZIBs which helps in decreasing the PMUs number that is required to make the power system complete observable and contingencies such as the case of loss of PMU and the case of single line failure to improve the power system reliability and guarantee complete observability of the power during losing of PMU. FPA is also has the ability of finding variety of PMUs location sets for the same number of PMUs which help in increasing the measurement redundancy of the system. And by comparing the obtained results of the suggested FPA with results of other optimization algorithms used to solve the OPP, it is clear that the suggested FPA is considered to be one of the methods that having high performance and efficiency in solving the OPP to find the minimum PMUs number to fulfil the power system observability at different case studies and different test systems with different number of buses and with different connections.
The authors declare no conflict of interest.
[1] | Schweitzer EO, Whitehead DE (2009) Real world synchrophasors solutions. 62nd Annual Conference for Protective Relay Engineers, 536-547. |
[2] | Lu Z, Xu Z, Shi Z, et al. (2000) State estimation of voltage phasors based on part of voltage and current phasors measurement. Autom Electr Power Syst 24: 42-44. |
[3] |
Kavasseri R, Srinivasa SK (2011) Joint placement of phasor and conventional power flow measurements for fault observability of power systems. IET Gener, Transm Distrib 5: 1019-1024. doi: 10.1049/iet-gtd.2010.0791
![]() |
[4] |
Dalali M, Karegar HK (2016) Optimal PMU placement for full observability of the power network with maximum redundancy using modified binary cuckoo optimization algorithm. IET Gener, Transm Distrib 10: 2817-2824. doi: 10.1049/iet-gtd.2016.0287
![]() |
[5] |
Rather ZH, Chen Z, Thøgersen P, et al. (2015) Realistic Approach for Phasor Measurement Unit Placement: Consideration of Practical Hidden Costs. IEEE Trans Power Delivery 30: 3-15. doi: 10.1109/TPWRD.2015.2478297
![]() |
[6] | Abdelaziz AY, Ibrahim AM, Salem RH (2013) Power system observability with minimum phasor measurement units placement. Int J Eng, Sci Technol, 5: 1-18. |
[7] |
Chakrabarti S, Kyriakides E (2008) Optimal placement of phasor measurement units for power system observability. IEEE Trans Power Syst 23: 1433-1440. doi: 10.1109/TPWRS.2008.922621
![]() |
[8] | Chakrabarti S, Kyriakides E, Eliades D (2009) Placement of synchronized measurements for power system observability. IEEE Trans Power Delivery 24: 12-19. |
[9] | Gou JB (2008) Generalized integer linear programming formulation for optimal PMU placement IEEE Trans Power Syst 23: 1099-1104. |
[10] | Dua D, Dambhare S, Gajbhiye R, et al. (2006) Optimal multistage scheduling of PMU placement: An ILP approach. IEEE Trans Power Delivery 23: 1812-1820. |
[11] | Abbasy NH, Ismail HM (2009) A unified approach for the optimal PMU location for power system state estimation. IEEE Trans Power Syst 24: 806-813. |
[12] |
Gou B, Kavasseri RG (2014) Unified PMU placement for observability and bad data detection in state estimation. IEEE Trans Power Syst 29: 2573-2580. doi: 10.1109/TPWRS.2014.2307577
![]() |
[13] | Babu R, Bhattacharyya B (2018) Strategic placements of PMUs for power network observability considering redundancy measurement. Measurement 134: 606-623. |
[14] | Razavi SE, Falaghi H, Singh C, et al. (2018) A novel linear framework for Phasor Measurement Unit placement considering the effect of adjacent zero-injection buses. Measurement 133: 532-540. |
[15] |
Müller HH, Castro CA (2016) Genetic algorithm-based phasor measurement unit placement method considering observability and security criteria. IET Gener, Transm Distrib 10: 270-280. doi: 10.1049/iet-gtd.2015.1005
![]() |
[16] | El-Sehiemy RA, Abdel Aleem SHE, Abdelaziz AY, et al. (2017) A new fuzzy framework for the optimal placement of phasor measurement units under normal and abnormal conditions. Resour-Effic Technol 3: 542-549. |
[17] |
Koutsoukis NC, Manousakis NM, Georgilakis PS, et al. (2013) Numerical observability method for optimal phasor measurement units placement using recursive Tabu search method. IET Gener, Transm Distrib 7: 347-356. doi: 10.1049/iet-gtd.2012.0377
![]() |
[18] |
Sodhi R, Srivastava SC, Singh SN (2011) Multi-criteria decision making approach for multistage optimal placement of phasor measurement units. IET Gener, Transm Distrib 5: 181-190. doi: 10.1049/iet-gtd.2009.0709
![]() |
[19] |
Saleh AA, Adail AS, Wadoud AA (2017) Optimal phasor measurement units placement for full observability of power system using improved particle swarm optimization. IET Gener, Transm Distrib 11: 1794-1800. doi: 10.1049/iet-gtd.2016.1636
![]() |
[20] |
Korres GN, Manousakis NM, Xygkis TC, et al. (2015) Optimal phasor measurement unit placement for numerical observability in the presence of conventional measurements using semidefinite programming. IET Gener, Transm Distrib 9: 2427-2436. doi: 10.1049/iet-gtd.2015.0662
![]() |
[21] |
Ram JP, Rajasekar N (2017) A novel flower pollination based global maximum power point method for solar maximum power point tracking'. IEEE Trans power Electron. 32: 8486-8499. doi: 10.1109/TPEL.2016.2645449
![]() |
[22] | Yang XS (2012) Flower pollination algorithm for global optimization. Unconv comput nat Comput, 240-249. |
[23] |
Yang XS, Karamanoglu M, He X (2013) Multi-objective Flower Algorithm for Optimization. Proc Comput Sci 18: 861-868. doi: 10.1016/j.procs.2013.05.251
![]() |
[24] |
Yang XS, Karamanoglu M, He X (2014) Flower pollination algorithm, 'a novel approach for multiobjective optimization. Eng Optim 46: 1222-1237. doi: 10.1080/0305215X.2013.832237
![]() |
[25] | Yang XS (2014) Nature-inspired Optimization Algorithms, Elsevier. |
[26] | Christie R (1999) Power System Test Archive. Available from: http://www.ee.washington.edu/research/pstca. |
[27] | Billakanti S, Venkaiah C (2014) An effective binary integer linear programmed approach for optimal placement of PMUs in power systems. IEEE International Conference on Smart Electric Grid (ISEG), 1-8. |
[28] |
Rahman NHA, Zobaa AF (2016) Optimal PMU placement using topology transformation method in power systems. J Adv Res 7: 625-634. doi: 10.1016/j.jare.2016.06.003
![]() |
[29] | Xu B, Abur A (2004) Observability analysis and measurement placement for systems with PMUs. IEEE PES Power Systems Conference and Exposition, 1472-1475. |
[30] |
Ahmadim A, Alinejad-beromi Y, Moradi M (2011) Optimal PMU placement for power system observability using binary particle swarm optimization and considering measurement redundancy. Expert Syst Appl 38: 7263-7269. doi: 10.1016/j.eswa.2010.12.025
![]() |
[31] | Mohammadi-Ivatloo B, Hosseini SH (2008) Optimal PMU placement for power system observability considering secondary voltage control. IEEE Canadian Conference on Electrical and Computer Engineering, 365-368. |
[32] | Al-Mohammed AH, Abido MA, Mansour MM (2011) Optimal placement of synchronized phasor measurement units based on differential evolution algorithm. IEEE PES Conference on Innovative Smart Grid Technologies-Middle East, 1-9. |
[33] | Rather ZH, Liu C, Chen Z, et al. (2013) Optimal PMU placement by improved particle swarm optimization. IEEE Innovative Smart Grid Technologies-Asia (ISGT Asia), 1-6. |
[34] |
Saha BK, Sinha AK, Pradhan AK (2012) An optimal PMU placement technique for power system observability. Int J Electr Power Energy Syst 42: 71-77. doi: 10.1016/j.ijepes.2012.03.011
![]() |
[35] |
Nadia HA, Zobaa AF (2017) Integrated Mutation Strategy with Modified Binary PSO Algorithm for Optimal PMUs Placement. IEEE Trans Ind Inf 13: 3124-3133. doi: 10.1109/TII.2017.2708724
![]() |
[36] | Manousakis NM, Korres GN (2017) Optimal allocation of PMUs in the presence of conventional measurements considering contingencies. IEEE Trans Power Delivery, 1-8. |
[37] |
Basetti V, Chandel AK (2017) Optimal PMU placement for power system observability using Taguchi binary bat algorithm. Measurement 95: 8-20. doi: 10.1016/j.measurement.2016.09.031
![]() |
[38] |
Aminifar F, Khodaei A, Fotuhi-Firuzabad M, et al. (2010) Contingency-constrained PMU placement in power networks. IEEE Trans Power Syst 25: 516-523. doi: 10.1109/TPWRS.2009.2036470
![]() |
[39] |
Hajian M, Ranjbar AM, Amraee T, et al. (2011) Optimal placement of PMUs to maintain network observability using a modified BPSO algorithm. Electr Power Energy Syst 33: 28-34. doi: 10.1016/j.ijepes.2010.08.007
![]() |
1. | Subhashree Priyadarshini, Chinmoy Kumar Panigrahi, 2021, Optimal Allocation of PMUs in the Eastern Region Indian Power Grid with Maximum Redundancy, 978-1-7281-8774-7, 1, 10.1109/ICPEE50452.2021.9358489 | |
2. | N. V. Phanendrababu, 2021, Chapter 5, 978-3-030-54274-0, 127, 10.1007/978-3-030-54275-7_5 | |
3. | Subhashree Priyadarshini, Chinmoy Kumar Panigrahi, 2020, Comparative Analysis between Binary Grey Wolf and Binary Bat Optimization for Optimal PMU Placement with Complete Observability, 978-1-6654-1917-8, 422, 10.1109/WIECON-ECE52138.2020.9397947 | |
4. | Lourdusamy Ramya Hyacinth, Venugopal Gomathi, Optimal PMU Placement Technique to Maximize Measurement Redundancy Based on Closed Neighbourhood Search, 2021, 14, 1996-1073, 4782, 10.3390/en14164782 | |
5. | Bishal Rimal, Nirmal Paudel, Aayush Bhattarai, 2022, Optimal Placement of Phasor Measurement Units Ensuring Power System Observability, 978-1-6654-9334-5, 7, 10.1109/SKIMA57145.2022.10029514 | |
6. | Beytullah Bozalı, Ali Öztürk, Salih Tosun, Designation of Optimal Location of Phasor Measurement Units by Comparing Different Methods for Türkiye 400 kV Power Systems, 2022, 50, 1532-5008, 1051, 10.1080/15325008.2022.2145390 | |
7. | V. Yuvaraju, S. Thangavel, Optimal phasor measurement unit placement for power system observability using teaching–learning based optimization, 2022, 137, 01420615, 107775, 10.1016/j.ijepes.2021.107775 | |
8. | Edwin Otieno Okendo, Cyrus Wabuge Wekesa, Michael Juma Saulo, Optimal placement of Phasor Measurement Unit considering System Observability Redundancy Index: case study of the Kenya power transmission network, 2021, 7, 24058440, e07670, 10.1016/j.heliyon.2021.e07670 | |
9. | Onkemetse Tshenyego, Ravi Samikannu, Bokani Mtengi, Modisa Mosalaosi, Tshiamo Sigwele, A Graph-Theoretic Approach for Optimal Phasor Measurement Units Placement Using Binary Firefly Algorithm, 2023, 16, 1996-1073, 6550, 10.3390/en16186550 |
Test systems | Zero injection buses | Radial buses | ||
No. | Locations | No. | Locations | |
14-bus | 1 | 7 | 1 | 8 |
30-bus | 7 | 6, 9, 22, 25, 27, 28 | 3 | 11, 13, 26 |
NE 39-bus | 12 | 1, 2, 5, 6, 9, 10, 11, 13, 14, 17, 19, 22 | 9 | 30, 31, 32, 33, 34, 35, 36, 37, 38 |
57-bus | 15 | 4, 7, 11, 21, 22, 24, 26, 34, 36, 37, 39, 40, 45, 46, 48 | 1 | 33 |
118-bus | 10 | 5, 9, 30, 37, 38, 63, 64, 68, 71, 81 | 7 | 10, 73, 87,111,112,116,117 |
Test System | Normal Case | ||
No. of PMUs | Locations sets | R. Index | |
14-bus | 4 | 2, 6, 7, 9 | 19 |
2, 6, 8, 9 | 17 | ||
30-bus | 10 | 2, 4, 6, 9, 10, 12, 15, 18, 25, 27 | 52 |
2, 4, 6, 9, 10, 12, 19, 24, 25, 27 | 51 | ||
NE 39-bus | 13 | 2, 6, 9, 10, 13, 14, 17, 19, 20, 22, 23, 25, 29 | 52 |
2, 6, 9, 10, 11, 14, 17, 19, 22, 23, 25, 29, 34 | 51 | ||
57-bus | 17 | 1, 4, 6, 9, 15, 20, 24, 28, 31, 32, 36, 38, 41, 47, 51, 53, 57 | 72 |
1, 4, 7, 9, 15, 20, 24, 28, 31, 32, 36, 38, 41, 46, 50, 53, 57 | 71 | ||
118-bus | 32 | 1, 5, 9, 11, 12, 17, 21, 23, 28, 30, 34, 37, 42, 45, 49, 53, 56, 62, 64, 68, 71, 75, 77, 80, 85, 87, 91, 94,101,105,110,115 | 160 |
3, 5, 10, 12, 13, 17, 21, 24, 25, 28, 34, 37, 40, 45, 49, 53, 56, 62, 63, 68, 71, 75, 77, 80, 85, 86, 91, 94,101,105,110,114 | 157 |
Test systems | Considering ZIB | ||
No. PMUs | Locations sets | R. Index | |
14-bus | 3 | 2, 6, 9 | 15 |
30-bus | 7 | 1, 7, 10, 12, 18, 24, 29 | 29 |
3, 5, 10, 12, 18, 24, 29 | 29 | ||
NE 39-bus | 8 | 3, 8, 12, 16, 20, 23, 25, 29 | 32 |
57-bus | 12 | 1, 6, 9, 14, 19, 25, 29, 32, 38, 41, 50, 53 | 52 |
2, 6, 12, 14, 19, 25, 29, 32, 38, 41, 51, 54 | 49 | ||
118-bus | 29 | 2, 8, 11, 12, 17, 20, 23, 28, 33, 34, 40, 45, 49, 53, 56, 61, 66, 72, 75, 77, 80, 85, 86, 91, 94,102,105,110,114 | 143 |
Test systems | Loss of PMU | ||
No. of PMUs | Locations sets | R. Index | |
14-bus | 9 | 2, 4, 5, 6, 7, 8, 9, 11, 13 | 39 |
1, 2, 4, 6, 7, 8, 9, 11, 13 | 37 | ||
30-bus | 21 | 1, 2, 3, 5, 6, 9, 10, 11, 12, 13, 15, 17, 18, 20, 22, 24, 25, 26, 27, 28, 29 | 83 |
2, 3, 4, 6, 7, 8, 9, 10, 11, 12, 13, 15, 17, 19, 20, 21, 23, 25, 26, 27, 30 | 82 | ||
NE 39-bus | 29 | 2, 3, 4, 6, 8, 9, 10, 11, 12, 14, 16, 17, 19, 20, 22, 23, 25, 26, 29, 30, 31, 32, 33, 34, 35, 36, 37, 38, 39 | 99 |
57-bus | 33 | 1, 3, 4, 6, 9, 11, 12, 15, 19, 20, 22, 24, 25, 27, 28, 29, 30, 32, 33, 35, 36, 38, 39, 41, 45, 46, 47, 50, 51, 53, 54, 56, 57 | 129 |
118-bus | 69 | 1, 3, 5, 7, 9, 10, 11, 12, 15, 17, 19, 21, 22, 24, 25, 27, 29, 30, 31, 32, 34, 36, 37, 40, 42, 44, 45, 46, 49, 51, 52, 54, 56, 57, 59, 62, 64, 65, 66, 68, 70, 71, 73, 75, 76, 77, 79, 80, 82, 83, 85, 86, 87, 89, 91, 92, 94, 96,100,102,105,106,109,110,111,112,114,116,117 | 313 |
Test systems | Single Line Outage | ||
No. of PMUs | Locations sets | R. Index | |
IEEE 14-bus | 7 | 2, 4, 5, 6, 9, 10, 13 | 33 |
IEEE 30-bus | 16 | 1, 2, 4, 5, 6, 10, 12, 15, 16, 18, 19, 22, 24, 27, 28, 30 | 71 |
NE 39-bus | 15 | 2, 3, 6, 8, 9, 11, 13, 14, 16, 17, 22, 23, 26, 29, 39 | 63 |
1, 2, 3, 6, 8, 9, 11, 13, 14, 16, 17, 22, 23, 26, 29 | 63 | ||
IEEE 57-bus | 32 | 1, 2, 4, 6, 9, 11, 12, 15, 19, 20, 22, 24, 25, 27, 28, 29, 30, 32, 34, 36, 37, 38, 39, 41, 45, 46, 47, 50, 51, 53, 54, 56 | 127 |
IEEE 118-bus | 61 | 1, 3, 5, 7, 8, 11, 12, 15, 17, 19, 21, 22, 24, 25, 27, 29, 30, 31, 32, 34, 36, 37, 40, 42, 44, 45, 46, 49, 51, 53, 54, 56, 57, 59, 62, 64, 65, 66, 68, 70, 71, 75, 76, 77, 79, 80, 82, 83, 85, 89, 91, 92, 94, 96,100,102,105,106,109,110,114 | 297 |
Algorithms | IEEE 14-bus | IEEE30-bus | NE39-bus | IEEE57-bus | IEEE118-bus |
Exhaustive Search Algorithm (ES) [7] | 4, R19 | 10, R50 | - | - | - |
Integer Quadratic Programming (IQP) [8] | 4, R19 | 10, R49 | - | 17, R69 | 32, R159 |
Binary Integer Linear Programming (BILP) [27] | 4, R18 | 10, R49 | - | 17, R69 | 32, R158 |
Topology Transformation Method (TT) [28] | 4, R19 | 10, R51 | - | 17, R71 | 32, R160 |
Staged ES [34] | 4, R18 | 10, R50 | 13, R49 | 17, R70 | 32, R159 |
Taguchi binary bat algorithm (TBBA) [37] | 4, R19 | 10, R51 | - | 17, R71 | 32, R160 |
Proposed FPA | 4, R19 | 10, R52 | 13, R52 | 17, R72 | 32, R160 |
Algorithms | 14-bus | 30-bus | NE 39-bus | 57-bus | 118-bus |
ES [7] | 3, R15 | 7, R28 | 8, R30 | - | - |
Integer Linear Programming (ILP) [11] | 3, R14 | 7, R28 | 8, R29 | 11, R50 | 28, R141 |
GA [15] | 3, R15 | 7, R29 | - | 12, R52 | 29, R142 |
TT [28] | 3, R15 | 7, R29 | 8, R31 | 11, R51 | 28, R143 |
ILP [29] | 3, R14 | - | - | 12, R53 | 29, R142 |
Binary Particle Swarm (BPSO) [30] | 3, R15 | 7, R29 | - | 13, R54 | 29, R143 |
Branch and Bound (B & B) [31] | - | 7, R27 | 9, R33 | 12, R53 | 29, R141 |
Differential Evolution (DE) [32] | 3, R15 | 7, R29 | 8, R31 | 11, R50 | 29, R143 |
Improved PSO (IPSO) [33] | - | 7, R29 | 8, R30 | 11, R52 | - |
Staged ES [34] | 3, R15 | 7, R29 | 8, R31 | 11, R49 | 28, R140 |
Proposed FPA | 3, R15 | 7, R29 | 8, R32 | 12, R52 | 29, R143 |
Algorithms | 14-bus | 30-bus | NE 39-bus | 57-bus | 118-bus |
IQP[8] | 9, R37 | 21, R80 | - | 33, R128 | 68, R310 |
Integer Programming (IP) [10] | 9, R39 | 22, R88 | - | 35, R133 | 72, R318 |
Modified Binary PSO (MBPSO) [35] | 9, R39 | 21, R82 | 28, R96 | 33, R129 | 68, R310 |
Binary Semi-Definite Programming (BSDP) [36] | 9, R38 | - | - | - | 72, R318 |
TBBA [37] | 9, R39 | 21, R82 | - | 33, R128 | 68, R312 |
Proposed FPA | 9, R39 | 21, R83 | 29, R99 | 33, R129 | 69, R313 |
Algorithms | 14-bus | 30-bus | NE 39-bus | 57-bus | 18-bus |
IQP [8] | 9, R37 | 21, R80 | - | 33, R128 | 68, R310 |
ILP [38] | 9, R36 | 21, R79 | 28, R97 | 33, R129 | 68, R307 |
MBPSO [39] | 9, R39 | - | - | - | 72, R317 |
Proposed FPA | 7, R33 | 16, R71 | 15, R63 | 32, R127 | 61, R297 |
Algorithms | Computer specifications | 14-bus | 30-bus | 57-bus | 118-bus |
IQP [8] | Intel Xeon 3.4-GHz CPU with 2 GB RAM | 3.09 | 2.6 | 4.24 | 3.09 |
Integer Linear Programming (ILP) [11] | 2.13-GHz Intel(R) Core(TM) 2 CPU with 2 GB RAM | 0.05 | - | 30.41 | 1625.30 |
ILP [29] | Intel Core2 Duo CPU E7500 running at 2.93-GHz with 2 GB RAM | 0.04 | - | 1.93 | 6.37 |
Differential Evolution (DE) [32] | Intel Xeon 3.4-GHz CPU with 2 GB RAM | 2 | 4 | 2 | 3 |
MBPSO [39] | Centrino 1.6-GHz CPU with 512 MBRAM | 0.052 | 0.071 | 0.106 | 0.169 |
Proposed FPA | Intel Core i7 2.67-GHz CPU with 6-GB RAM | 0.02 | 0.51 | 0.1 | 0.1 |
Test systems | Zero injection buses | Radial buses | ||
No. | Locations | No. | Locations | |
14-bus | 1 | 7 | 1 | 8 |
30-bus | 7 | 6, 9, 22, 25, 27, 28 | 3 | 11, 13, 26 |
NE 39-bus | 12 | 1, 2, 5, 6, 9, 10, 11, 13, 14, 17, 19, 22 | 9 | 30, 31, 32, 33, 34, 35, 36, 37, 38 |
57-bus | 15 | 4, 7, 11, 21, 22, 24, 26, 34, 36, 37, 39, 40, 45, 46, 48 | 1 | 33 |
118-bus | 10 | 5, 9, 30, 37, 38, 63, 64, 68, 71, 81 | 7 | 10, 73, 87,111,112,116,117 |
Test System | Normal Case | ||
No. of PMUs | Locations sets | R. Index | |
14-bus | 4 | 2, 6, 7, 9 | 19 |
2, 6, 8, 9 | 17 | ||
30-bus | 10 | 2, 4, 6, 9, 10, 12, 15, 18, 25, 27 | 52 |
2, 4, 6, 9, 10, 12, 19, 24, 25, 27 | 51 | ||
NE 39-bus | 13 | 2, 6, 9, 10, 13, 14, 17, 19, 20, 22, 23, 25, 29 | 52 |
2, 6, 9, 10, 11, 14, 17, 19, 22, 23, 25, 29, 34 | 51 | ||
57-bus | 17 | 1, 4, 6, 9, 15, 20, 24, 28, 31, 32, 36, 38, 41, 47, 51, 53, 57 | 72 |
1, 4, 7, 9, 15, 20, 24, 28, 31, 32, 36, 38, 41, 46, 50, 53, 57 | 71 | ||
118-bus | 32 | 1, 5, 9, 11, 12, 17, 21, 23, 28, 30, 34, 37, 42, 45, 49, 53, 56, 62, 64, 68, 71, 75, 77, 80, 85, 87, 91, 94,101,105,110,115 | 160 |
3, 5, 10, 12, 13, 17, 21, 24, 25, 28, 34, 37, 40, 45, 49, 53, 56, 62, 63, 68, 71, 75, 77, 80, 85, 86, 91, 94,101,105,110,114 | 157 |
Test systems | Considering ZIB | ||
No. PMUs | Locations sets | R. Index | |
14-bus | 3 | 2, 6, 9 | 15 |
30-bus | 7 | 1, 7, 10, 12, 18, 24, 29 | 29 |
3, 5, 10, 12, 18, 24, 29 | 29 | ||
NE 39-bus | 8 | 3, 8, 12, 16, 20, 23, 25, 29 | 32 |
57-bus | 12 | 1, 6, 9, 14, 19, 25, 29, 32, 38, 41, 50, 53 | 52 |
2, 6, 12, 14, 19, 25, 29, 32, 38, 41, 51, 54 | 49 | ||
118-bus | 29 | 2, 8, 11, 12, 17, 20, 23, 28, 33, 34, 40, 45, 49, 53, 56, 61, 66, 72, 75, 77, 80, 85, 86, 91, 94,102,105,110,114 | 143 |
Test systems | Loss of PMU | ||
No. of PMUs | Locations sets | R. Index | |
14-bus | 9 | 2, 4, 5, 6, 7, 8, 9, 11, 13 | 39 |
1, 2, 4, 6, 7, 8, 9, 11, 13 | 37 | ||
30-bus | 21 | 1, 2, 3, 5, 6, 9, 10, 11, 12, 13, 15, 17, 18, 20, 22, 24, 25, 26, 27, 28, 29 | 83 |
2, 3, 4, 6, 7, 8, 9, 10, 11, 12, 13, 15, 17, 19, 20, 21, 23, 25, 26, 27, 30 | 82 | ||
NE 39-bus | 29 | 2, 3, 4, 6, 8, 9, 10, 11, 12, 14, 16, 17, 19, 20, 22, 23, 25, 26, 29, 30, 31, 32, 33, 34, 35, 36, 37, 38, 39 | 99 |
57-bus | 33 | 1, 3, 4, 6, 9, 11, 12, 15, 19, 20, 22, 24, 25, 27, 28, 29, 30, 32, 33, 35, 36, 38, 39, 41, 45, 46, 47, 50, 51, 53, 54, 56, 57 | 129 |
118-bus | 69 | 1, 3, 5, 7, 9, 10, 11, 12, 15, 17, 19, 21, 22, 24, 25, 27, 29, 30, 31, 32, 34, 36, 37, 40, 42, 44, 45, 46, 49, 51, 52, 54, 56, 57, 59, 62, 64, 65, 66, 68, 70, 71, 73, 75, 76, 77, 79, 80, 82, 83, 85, 86, 87, 89, 91, 92, 94, 96,100,102,105,106,109,110,111,112,114,116,117 | 313 |
Test systems | Single Line Outage | ||
No. of PMUs | Locations sets | R. Index | |
IEEE 14-bus | 7 | 2, 4, 5, 6, 9, 10, 13 | 33 |
IEEE 30-bus | 16 | 1, 2, 4, 5, 6, 10, 12, 15, 16, 18, 19, 22, 24, 27, 28, 30 | 71 |
NE 39-bus | 15 | 2, 3, 6, 8, 9, 11, 13, 14, 16, 17, 22, 23, 26, 29, 39 | 63 |
1, 2, 3, 6, 8, 9, 11, 13, 14, 16, 17, 22, 23, 26, 29 | 63 | ||
IEEE 57-bus | 32 | 1, 2, 4, 6, 9, 11, 12, 15, 19, 20, 22, 24, 25, 27, 28, 29, 30, 32, 34, 36, 37, 38, 39, 41, 45, 46, 47, 50, 51, 53, 54, 56 | 127 |
IEEE 118-bus | 61 | 1, 3, 5, 7, 8, 11, 12, 15, 17, 19, 21, 22, 24, 25, 27, 29, 30, 31, 32, 34, 36, 37, 40, 42, 44, 45, 46, 49, 51, 53, 54, 56, 57, 59, 62, 64, 65, 66, 68, 70, 71, 75, 76, 77, 79, 80, 82, 83, 85, 89, 91, 92, 94, 96,100,102,105,106,109,110,114 | 297 |
Algorithms | IEEE 14-bus | IEEE30-bus | NE39-bus | IEEE57-bus | IEEE118-bus |
Exhaustive Search Algorithm (ES) [7] | 4, R19 | 10, R50 | - | - | - |
Integer Quadratic Programming (IQP) [8] | 4, R19 | 10, R49 | - | 17, R69 | 32, R159 |
Binary Integer Linear Programming (BILP) [27] | 4, R18 | 10, R49 | - | 17, R69 | 32, R158 |
Topology Transformation Method (TT) [28] | 4, R19 | 10, R51 | - | 17, R71 | 32, R160 |
Staged ES [34] | 4, R18 | 10, R50 | 13, R49 | 17, R70 | 32, R159 |
Taguchi binary bat algorithm (TBBA) [37] | 4, R19 | 10, R51 | - | 17, R71 | 32, R160 |
Proposed FPA | 4, R19 | 10, R52 | 13, R52 | 17, R72 | 32, R160 |
Algorithms | 14-bus | 30-bus | NE 39-bus | 57-bus | 118-bus |
ES [7] | 3, R15 | 7, R28 | 8, R30 | - | - |
Integer Linear Programming (ILP) [11] | 3, R14 | 7, R28 | 8, R29 | 11, R50 | 28, R141 |
GA [15] | 3, R15 | 7, R29 | - | 12, R52 | 29, R142 |
TT [28] | 3, R15 | 7, R29 | 8, R31 | 11, R51 | 28, R143 |
ILP [29] | 3, R14 | - | - | 12, R53 | 29, R142 |
Binary Particle Swarm (BPSO) [30] | 3, R15 | 7, R29 | - | 13, R54 | 29, R143 |
Branch and Bound (B & B) [31] | - | 7, R27 | 9, R33 | 12, R53 | 29, R141 |
Differential Evolution (DE) [32] | 3, R15 | 7, R29 | 8, R31 | 11, R50 | 29, R143 |
Improved PSO (IPSO) [33] | - | 7, R29 | 8, R30 | 11, R52 | - |
Staged ES [34] | 3, R15 | 7, R29 | 8, R31 | 11, R49 | 28, R140 |
Proposed FPA | 3, R15 | 7, R29 | 8, R32 | 12, R52 | 29, R143 |
Algorithms | 14-bus | 30-bus | NE 39-bus | 57-bus | 118-bus |
IQP[8] | 9, R37 | 21, R80 | - | 33, R128 | 68, R310 |
Integer Programming (IP) [10] | 9, R39 | 22, R88 | - | 35, R133 | 72, R318 |
Modified Binary PSO (MBPSO) [35] | 9, R39 | 21, R82 | 28, R96 | 33, R129 | 68, R310 |
Binary Semi-Definite Programming (BSDP) [36] | 9, R38 | - | - | - | 72, R318 |
TBBA [37] | 9, R39 | 21, R82 | - | 33, R128 | 68, R312 |
Proposed FPA | 9, R39 | 21, R83 | 29, R99 | 33, R129 | 69, R313 |
Algorithms | 14-bus | 30-bus | NE 39-bus | 57-bus | 18-bus |
IQP [8] | 9, R37 | 21, R80 | - | 33, R128 | 68, R310 |
ILP [38] | 9, R36 | 21, R79 | 28, R97 | 33, R129 | 68, R307 |
MBPSO [39] | 9, R39 | - | - | - | 72, R317 |
Proposed FPA | 7, R33 | 16, R71 | 15, R63 | 32, R127 | 61, R297 |
Algorithms | Computer specifications | 14-bus | 30-bus | 57-bus | 118-bus |
IQP [8] | Intel Xeon 3.4-GHz CPU with 2 GB RAM | 3.09 | 2.6 | 4.24 | 3.09 |
Integer Linear Programming (ILP) [11] | 2.13-GHz Intel(R) Core(TM) 2 CPU with 2 GB RAM | 0.05 | - | 30.41 | 1625.30 |
ILP [29] | Intel Core2 Duo CPU E7500 running at 2.93-GHz with 2 GB RAM | 0.04 | - | 1.93 | 6.37 |
Differential Evolution (DE) [32] | Intel Xeon 3.4-GHz CPU with 2 GB RAM | 2 | 4 | 2 | 3 |
MBPSO [39] | Centrino 1.6-GHz CPU with 512 MBRAM | 0.052 | 0.071 | 0.106 | 0.169 |
Proposed FPA | Intel Core i7 2.67-GHz CPU with 6-GB RAM | 0.02 | 0.51 | 0.1 | 0.1 |