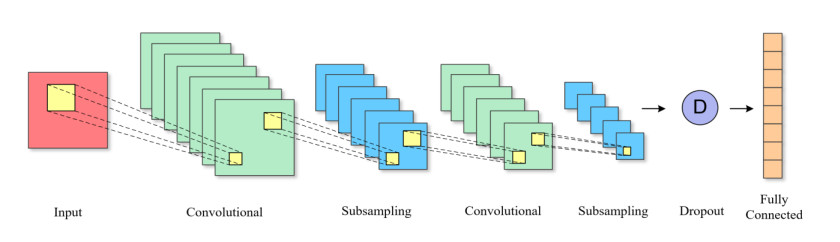
Algorithmic trading is one of the most concerned directions in financial applications. Compared with traditional trading strategies, algorithmic trading applications perform forecasting and arbitrage with higher efficiency and more stable performance. Numerous studies on algorithmic trading models using deep learning have been conducted to perform trading forecasting and analysis. In this article, we firstly summarize several deep learning methods that have shown good performance in algorithmic trading applications, and briefly introduce some applications of deep learning in algorithmic trading. We then try to provide the latest snapshot application for algorithmic trading based on deep learning technology, and show the different implementations of the developed algorithmic trading model. Finally, some possible research issues are suggested in the future. The prime objectives of this paper are to provide a comprehensive research progress of deep learning applications in algorithmic trading, and benefit for subsequent research of computer program trading systems.
Citation: Yongfeng Wang, Guofeng Yan. Survey on the application of deep learning in algorithmic trading[J]. Data Science in Finance and Economics, 2021, 1(4): 345-361. doi: 10.3934/DSFE.2021019
[1] | Jiawei He, Roman N. Makarov, Jake Tuero, Zilin Wang . Performance evaluation metric for statistical learning trading strategies. Data Science in Finance and Economics, 2024, 4(4): 570-600. doi: 10.3934/DSFE.2024024 |
[2] | Manveer Kaur Mangat, Erhard Reschenhofer, Thomas Stark, Christian Zwatz . High-Frequency Trading with Machine Learning Algorithms and Limit Order Book Data. Data Science in Finance and Economics, 2022, 2(4): 437-463. doi: 10.3934/DSFE.2022022 |
[3] | Habib Zouaoui, Meryem-Nadjat Naas . Option pricing using deep learning approach based on LSTM-GRU neural networks: Case of London stock exchange. Data Science in Finance and Economics, 2023, 3(3): 267-284. doi: 10.3934/DSFE.2023016 |
[4] | Yaya Su, Yi Qu, Yuxuan Kang . Online public opinion and asset prices: a literature review. Data Science in Finance and Economics, 2021, 1(1): 60-76. doi: 10.3934/DSFE.2021004 |
[5] | Wojciech Kuryłek . Can we profit from BigTechs' time series models in predicting earnings per share? Evidence from Poland. Data Science in Finance and Economics, 2024, 4(2): 218-235. doi: 10.3934/DSFE.2024008 |
[6] | Jawad Saleemi . Political-obsessed environment and investor sentiments: pricing liquidity through the microblogging behavioral perspective. Data Science in Finance and Economics, 2023, 3(2): 196-207. doi: 10.3934/DSFE.2023012 |
[7] | Mohamed F. Abd El-Aal . Analysis Factors Affecting Egyptian Inflation Based on Machine Learning Algorithms. Data Science in Finance and Economics, 2023, 3(3): 285-304. doi: 10.3934/DSFE.2023017 |
[8] | Sina Gholami, Erfan Zarafshan, Reza Sheikh, Shib Sankar Sana . Using deep learning to enhance business intelligence in organizational management. Data Science in Finance and Economics, 2023, 3(4): 337-353. doi: 10.3934/DSFE.2023020 |
[9] | Ming Li, Ying Li . Research on crude oil price forecasting based on computational intelligence. Data Science in Finance and Economics, 2023, 3(3): 251-266. doi: 10.3934/DSFE.2023015 |
[10] | Kazuo Sano . Intelligence and global bias in the stock market. Data Science in Finance and Economics, 2023, 3(2): 184-195. doi: 10.3934/DSFE.2023011 |
Algorithmic trading is one of the most concerned directions in financial applications. Compared with traditional trading strategies, algorithmic trading applications perform forecasting and arbitrage with higher efficiency and more stable performance. Numerous studies on algorithmic trading models using deep learning have been conducted to perform trading forecasting and analysis. In this article, we firstly summarize several deep learning methods that have shown good performance in algorithmic trading applications, and briefly introduce some applications of deep learning in algorithmic trading. We then try to provide the latest snapshot application for algorithmic trading based on deep learning technology, and show the different implementations of the developed algorithmic trading model. Finally, some possible research issues are suggested in the future. The prime objectives of this paper are to provide a comprehensive research progress of deep learning applications in algorithmic trading, and benefit for subsequent research of computer program trading systems.
The global financial market has greatly stimulated the rise of computer program trading and algorithmic trading systems. Algorithmic trading is an investment analysis method that combines computer technology and financial data. Compared with traditional trading methods, algorithmic trading is adept in finding short-term abnormalities of market prices, executing orders with program logic, and profiting from financial markets. Specially, deep learning technology provides powerful analytical technology for algorithmic trading. Deep learning has been proven to be useful for predicting price fluctuations in stocks and foreign exchange (Sirignano, 2019). Since the advantage of artificial intelligence, more and more investors use deep learning models to predict and analyze stock or foreign exchange prices. This article focuses on how to apply deep learning methods to algorithmic trading analysis and strategy design. We select different deep learning methods to classify papers, including convolutional neural networks (CNN), long short term memory networks (LSTM), deep multilayer perceptron (DMLP) and other deep learning methods, such as reinforcement learning (RL), restricted boltzmann machine (RBM) (Sutskever, 2009), recurrent neural network (RNN) (Mikolov, 2010), deep belief network (DBN) (Hinton, 2009), etc.
There are huge heterogeneous financial data in financial applications, including structured data, unstructured data, and semi-structured data. Not only are they complex in types and huge in volume, they are also mixed with a lot of noise. Financial big data is very important for organizations that need to collect large amounts of data (such as banks, securities companies), and one of the most important assets is the information discovered from the large amounts of data by using deep learning (Sohangir, 2018). Compared to traditional machine learning models, in general, the performance are significantly improved based on algorithmic trading strategies established by applying deep learning to analyze and predict financial data.
The other parts of this article are arranged as follows. After a brief introduction, in Section 2, we introduce the definition of algorithmic trading. In Section 3, we provide working deep learning models used in algorithmic trading applications. Section 4 classifies papers on algorithmic trading according to deep learning methods. We discuss the work done so far in this field and the direction of the industry's development in Section 5. Finally, we summarize the findings and draw conclusions in Section 6.
Algorithmic trading is defined as buying and selling decisions made by algorithmic trading systems. These systems try to capture short-lived abnormalities in market prices, profit from statistical patterns within or between financial markets. Moreover, these systems try to disguise traders' intentions, detect and exploit competitors' strategies (Nuti, 2011). Most algorithmic trading focuses on the future price changes and fluctuations in highly liquid markets. Algorithmic trading system generates trading signals based on strategies when trading opportunities appear. Due to the stability and availability, algorithmic trading attracts more and more investors.
Compared with traditional human-defined strategy rules, algorithmic strategy systems are more stably due to the lack of negative emotions of human investors, such as panic and greed. High-frequency trading (HFT) researchers keep also shown a strong interest in this field since the many advantages of algorithmic trading based on deep learning. According to the summary of Treleaven et al. (2013), algorithmic trading accounted for more than 70% of American stocks trading volume in 2011. Hendershott and Riordan studied the role of algorithmic trading in price formation and pointed out the algorithmic trading was more useful for price flow than manual trading (Hendershott and Riordan, 2009). Boehmer et al. (2012) showed that algorithmic trading had an impact on market quality (such as liquidity, efficiency, and volatility). According to the summary of Treleaven et al. (2013), algorithmic trading accounted for more than 70% of American stocks trading volume in 2011. Therefore, algorithmic trading systems are the main focus of regulatory agencies.
There are several challenges that algorithmic trading faces. American stocks usually exhibit drastic fluctuations in end-of-day (EOD). Moreover, compared with the trading time points in the day, the trading volume is enlarged several times before the stock market closes, which may lead to mistake of the system models and cause loss of profits. In order to improve the security performance of the algorithmic trading system, a major challenge is proposed that how to build an effective real-time risk management model. Also, an algorithmic trading system may have several or many variables as input. Usually, the experimental result is catastrophically affected by a minor change in variable. There is diversity in the structure of the deep learning model, and which handles the input differently. Therefore, the selection of deep learning model keeps a decisive influence on the performance of the system. Furthermore, when financial data is employed as the data set of the system, how to ensure high-quality data is also one of the challenges faced by algorithmic trading.
Deep learning is a special algorithm of machine learning, which is composed of multiple artificial neural network layers. Deep learning extracts features multiple times by setting multiple feature layers to further obtain higher-dimensional features for classification (Chen, 2016). The key aspect of deep learning is that these feature layers are not designed by human engineers: they are learned from data using common learning procedures (LeCun, 2015). Three deep learning models, i.e., CNN (Convolutional Neural Network), LSTM (Long Short Term Memory), and DMLP (Deep Multi-Layer Perceptron), are introduced in the following.
CNN is mainly divided into three layers, i.e., convolutional layer, pooling layer and fully connected layer. Each convolution layer contains multiple convolution kernels, and each layer of convolution receives the output of the previous layer as input. Convolution extracts the main features of the data. However, the extracted feature is hard to be processed due to the high-dimensionality. To overcome the challenge, the pooling layer reduces the feature dimensionality to reduce the training cost of networks (Lu, 2020). Through continuous convolution pooling and continuous extraction of effective features, high-quality features and classification capabilities are be obtained (Chen, 2016). The structure of CNN model is shown in Figure 1.
LSTM is a branch of deep recurrent neural networks. LSTM introduces a memory unit, which is a computing unit that replaces the traditional artificial neurons in the hidden layer of the network (Chen, 2015). Its memory unit consists of three parts: forget gates, inputs, and output gates. LSTM controls the data transmission state through the gating state. It prevents the loss of important features and the entire sequence by using long-term memory, while retaining short-term memory (Kim, 2019). The LSTM model structure is shown in Figure 2.
The DMLP model is mainly composed of input layer, hidden layer and output layer. The number of layers and the number of neurons in each layer are the hyperparameters of DMLP. Generally speaking, each neuron in the hidden layer consists of input, weight, and bias terms. In addition, each neuron has a non-linear activation function, which produces the cumulative output of the previous neuron. Common activation functions are sigmoid function (Cybenko, 1989), tanh function (Kalman, 1992) and relu function (Nair, 2010). The DMLP structure is shown in Figure 3.
In addition to the deep learning models mentioned above, there are many well-known structures, such as Reinforcement Learning (RL), Deep Q-learning network (DQN), AutoEncoders (AE), and RNN (Recurrent neural network). These methods do not appear frequently in the papers selected in this article, but this does not mean that these models are not suitable for algorithmic trading. On the contrary, they provide great potential for the development of algorithmic trading and the research of researchers. For example, RL has been widely used in many fields of financial forecasting since AlphaGo defeated the best chess player (Chen, 2018). We discuss these models in subsection 4.4.
The different algorithmic trading systems based on the deep learning model are used for different training objectives. Some predict the price trends of financial assets (stocks, indices, bonds, currencies, etc.), some execute actual transactions based on buying and selling signals, and some obtain asset returns by implementing real-world financial scenarios. There are also some systems for other independent research (pair trading, buying and selling signals, etc.). We classify the investigated papers according to the deep learning model below, and further divide them according to the training objectives of the model.
Prediction. To predict price trends and price fluctuations, Doering et al. (2017) used CNN to train on market microstructure data. They converted the high-frequency market microstructure data from the London Stock Exchange into four input channels based on market events, and trained six deep networks for the task. The results showed their model improved the forecast accuracy for both limit order book and order flow information. Gudelek et al. (2017) developed a CNN-based stock trend prediction model, which is different from other stock prediction models based on computational intelligence. This specific model analyzed time series values based on the technical analysis of the x-axis, and the clustering and ranking characteristics of the y-axis. Values (such as RSI, SMA, EMA, MACD, etc.) generated a two-dimensional image. They evaluated the model using a trading system based on test data, and the results showed that they could predict the next day's price with 72% accuracy. In addition, the model was profitable during the test period and outperformed the Buy-and-Hold (BaH) model. Selvin et al. (2017) used CNN, LSTM, and RNN architectures to predict the prices of National Stock Exchange (NSE) listed companies and compare their performance. They applied a sliding window method to predict short-term future values, and used percentage errors to measure the performance of the model. The results showed that the results given by CNN are more accurate than LSTM and RNN. Compared with LSTM and RNN, CNN is more aware of the dynamic changes and patterns that occur in the current window. As a result, it can identify the trend of direction changes. Hoseinzade et al. (2019) introduced and applied two variants based on deep CNN, one is 2D-CNNpred and the other is 3D-CNNpred. The number of prediction days and the number of predictors per day constitute the two dimensions of 2D-CNNpred. The initial daily variable, the date of the historical record, and the market of the collected data constitute the three dimensions of the 3D-CNNpred input tensor. By using CNNpred, higher-level features are extracted from the rich initial variables. The results show that in terms of F-measure, CNNpred can improve the prediction performance of all five indicators by about 3% to 11% over the baseline algorithm.
Arbitrage. Sezer et al. (2018) analyzed Dow30 stock price and Exchange Traded Fund (ETF) using 15 different technical indicators, and each indicator instance generated 15-day period data. Then, they converted these data into 15 × 15 two-dimensional images using image processing characteristics based Two-dimensional convolutional neural network for training prediction. They used CNN to determine the entry and exit points of time series values as the "buy", "sell" and "hold" markers in algorithmic trading. Finally, a trading operation was performed on each stock in the data set according to the predicted mark. The results showed that the average annualized return of their proposed model was 12%, and the percentage of successful transactions was 71%. Chen et al. (2018) improved the CNN model based on knowledge in the financial field and filter bank mechanism, and proposed Filter-bank CNN. They used an improved time series visualization method to convert historical volatility with different time ranges into two-dimensional images that captured arbitrage signals, and extract high-quality features by replacing randomly generated filters with arbitrage knowledge filters. Compared with the traditional rule-based strategy, the model obtained the accuracy rate of 67%, which improved the accuracy rate by 15%. Sezer et al. (2019) proposed a new algorithmic trading model, CNN-BI (CNN with striped images), using two-dimensional CNN. Based on a data set of Dow30 stock, Sezer et al. generated a two-dimensional image of a 30-day bar chart sliding window from the chart image without any other time series data. Based on the trained deep CNN model, they designed the algorithmic trading strategy to identify buying and selling decisions using stock bar graph images. The result showed that their strategy implemented higher annualized profit return than the BaH strategy based on market conditions.
Other training target. To find the optimal trading strategy, Luo et al. (2019) constructed an actor-critic RL algorithm, named DDPG (Deep Deterministic Policy Gradient), based on AI-trader. DDPG consists of two different CNN-based function approximators. To use the image as the input of the CNN, DDPG converts the technical indicators into multi-channels. The DDPG-base trading strategy generates triple −1, 0, 1 (short, neutral, long) trading signals, where "1" means the strategy closes out a short position and takes a long position, "−1" the strategy closes out the long position and takes a short position if the label is, and "0" means to hold the position. During the testing period, the DDPG-base trading strategy achieved a considerable annual rate of return compared with random trading strategies. Gunduz et al. (2017) proposed a CNN architecture to predict the intraday direction of Borsa Istanbul 100 stocks. They trained logistic regression (LR) and CNN classifiers, and measured the performance of the classifiers according to the macro-averaged (MA) F-Measure index, which can measure how well a classifier can distinguish among different classes. To check the influence of feature correlation by training two CNNs with different feature orders, one is CNN-Rand and the other is CNN-Corr. CNN-Rand employs random-ordered features in input data and CNN-Corr employs re-ranked features based on cluster feature correlation. The results show CNN-Corr achieves a higher F-Measure rate than CNN-Rand.
Table 1 shows the related algorithmic trading research using the CNN method.
Publication | Data set | Period | Method | Main training target |
Doering et al. (2017) | London stock exchange | 2007–2008 | CNN | Prediction |
Sezer et al. (2018) | ETFs and stocks in Dow 30 | 2007–2017 | CNN | Arbitrage |
Gudelek et al. (2017) | 17 ETFs | 2000–2017 | CNN | Prediction |
Selvin et al. (2017) | Stocks in Infosys, TCS, Cipla | 2014–2014 | CNN sliding-window | Prediction |
Chen et al. (2018) | Taiwan Stock Index Futures and Mini Index Futures | 2012–2014 | CNN + visualization method | Arbitrage |
Sezer et al. (2019) | Stocks in Dow 30 | 1997–2017 | CNN with feature imaging | Arbitrage |
Luo et al. (2019) | Stock-index future in China financial market | 2017–2018 | CNN with RL | Buy-sell-hold Signal |
Gunduz et al. (2017) | Borsa Istanbul 100 stocks | 2011–2015 | CNN, LR | Features correlation |
Hoseinzade et al. (2019) | Daily direction of the close of S & P 500 index, NASDAQ Composite, Dow Jones Industrial Average, NYSE Composite, and RUSSELL 2000 | 2010–2017 | CNN | Prediction |
Prediction. Mudassir et al. (2020) proposed a new method for predicting the price of Bitcoin in the short and medium term by using classification and regression models based on high-performance machine learning. To predict Bitcoin trends and prices, they used LSTM, Artificial Neural Network (ANN), Stacked Artificial Neural Network (SANN) and Support Vector Machine (SVM) to analyze the characteristics of Bitcoin price fluctuations. To train and test their models, they firstly processed the technical indicators and original characteristics of Bitcoin, and then, divided the data into three intervals based on the date. They used three indicators, i.e., mean absolute error (MAE), root mean square error (RMSE), and mean absolute percentage error (MAPE), to comprehensively evaluate the performance of these models. The results show that their models keep good performance, and the LSTM is the best. For daily price forecast, the MAPE is as low as 1.44%, while the seven to ninety days MAPE varies from 2.88% to 4.10%. Lin et al. (2018) proposed a neural network model including two layers of LSTM. To predict the trend of 10 stocks, they employed 13 features as input data. By comparing the RMSE, it is found that one stock has greater volatility, which means that there may be too many external factors that affect the stock price. Baek et al. (2018) proposed a framework, ModAugNet, based on LSTM, which is a new data enhancement method for stock market index prediction. The framework consists of two modules: the overfitting prevention LSTM module and the predictive LSTM module. They used the comprehensive evaluation framework with mean squared error (MSE), MAPE and MAE to access how well the data fits. Compared with the traditional models, their model is robust to overfit problems and does not need to generate artificial time series to enhance the available training data. The detection MSE, MAPE, and MAE of S & P500 dropped to 54%, 35%, and 32% of the S & P500 prediction error corresponding to the comparative model, respectively. Shah et al. (2018) used LSTM and DNN to predict the daily and weekly activities of the Indian BSE Sensex index. They used RMSE and forecast bias to compare the performance of the two models. It turns out that LSTM outperforms DNN in weekly forecasting. Zhao et al. (2017) proposed a time-weighted model for stock trend prediction. Based on the closeness of the data to the data to be predicted in time, they employed a time-weighted function to carefully assign weights to data. An LSTM-based model was established in the experiment to predict the redefined trend, and an accuracy of 83% was achieved on the CSI 300. Nelson et al. (2017) proposed a classification model based on LSTM to predict the price trend of stocks (forecast whether the stock will be higher than the current price in the next 15 minutes). They perform a logarithmic transformation on the data as a method of normalization and stabilize the mean and variance along the time series. The experimental results show that the average accuracy rate is as high as 55.9% when predicting whether the price of a particular stock will rise in the near future.
Arbitrage. Fang et al. (2019) set 50 ETF options in the options market with high transaction complexity as the research objective. According to the actual trading situation, they selected a 15-minute trading frequency that is more in line with the actual trading situation, and then introduced the delta hedging concept of options to control the risk quantitative investment strategy and realize the 15-minute hedging strategy. They used two different LSTM-SVR (support vector regression) models to predict the final trading price of 50ETF in the next time period, and selected the model with a better result to the trading strategy. Zou et al. (2020) constructed three deep learning models and a traditional time series model to forecast the stock prices for the next day. Three deep learning models included Autoregressive Integrated Moving Average (ARIMA), Stacked-LSTM, and Attention-Based LSTM (Attention-LSTM). According to the prediction result, they proposed two trading strategies: Long-Only and Long-Short. For the Long-Only strategy, the stock is bought at the opening price of the day and sold at the closing price of the day when the forecast is positive. Otherwise, no action is taken. Long-Short strategy means the operation strategy is the same as Long-Only when the forecast is positive. If the forecast is negative, short the stock at the opening price and close the short at the closing price. In the experiment, they achieve the annual return of 266% based on Long-Only strategy attention-LSTM and the annual return of 263% based on Long-Short strategy attention-LSTM. The results show the attention-LSTM is superior to other models.
Other training target. To study the relationship between market indicators and the entering/exiting decision, Troiano et al. (2018) used LSTM to establish multiple controlled experiments. They used MSE and cross entropy (CE) as performance indicators, and found the performance of the model decreased rapidly with the number of technical indicators increasing. The reason is that the increasing number of features make the training time shorter, and lead to the overfitting model.
Table 2 shows the related algorithmic trading research using the LSTM method.
Publication | Data set | Period | Method | Main training target |
Mudassir et al. (2020) | BTC features and price | 2013–2019 | LSTM, ANN, AVM, SANN | Prediction |
Fang et al. (2019) | Shanghai 50 ETF options | 2015–2018 | LSTM, RF, LSTM-SVRs | Arbitrage |
Troiano et al. (2018) | Dow30 stocks | 2012–2016 | LSTM | Long-short-hold position |
Lin et al. (2018) | Taiwan stock exchange corporation | – | LSTM, RNN | Prediction |
Baek et al. (2018) | Korea composite stock price index 200 and S & P500 | 2010–2016 | LSTM with prevention module, prediction module | Prediction |
Shah et al. (2018) | Indian BSE Sensex index and Tech Mahindra stock price | 1997–2017 | LSTM, DNN | Prediction |
Zhao et al. (2017) | Stock indexes in SSE, SSE50, CSI500, CSI300 and SZSE | 2002–2017 | LSTM | Prediction |
Nelson et al. (2017) | 5 stocks in Brazilian stock exchange | 2008–2015 | LSTM | Prediction |
Zou et al. (2020) | Stocks in SP500 and the accounting and corporate statistics for the stocks | 2004–2013 | LSTM, RNN, ARIMA | Arbitrage |
Prediction. To predict the price of Chicago Mercantile Exchange (CME) listed futures, Dixon et al. (2017) applied 43 CME commodity prices and FX futures as a data set and used DNN to train all trading varieties together. In order to classify effective sample, they firstly introduced F1-scores to measure the robustness of performance. Then based on the experiment prediction results, their strategy-based DNN generated trading labels of 1 or −1 for the sample. Finally, they executed the transaction and compared the results of the transaction. Singh et al. (2017) compared 2-Directional 2-Dimensional Principal Component Analysis ((2D)²PCA) + DNN method with (2D)²PCA + Radial Basis Function Neural Network (RBFNN) method. They found that the performance of the proposed method is better than that of RBFNN. The correlation coefficient between the actual revenue and the predicted revenue of DNN is 17.1% higher than RBFNN, and 43.4% higher than that of RNN. Ji et al. (2019) studied regression problems and classification problems by combining DMLP, LSTM, CNN, deep residual network (ResNet) to predict the price of Bitcoin. Regression predicts the future bitcoin price, and classification predicts whether the future bitcoin price will rise or fall. The results show that although the LSTM-based prediction model performs slightly better than other prediction models in Bitcoin price prediction (regression), the DNN-based model performs best in price increase and decrease prediction (classification).
Arbitrage. Sezer et al. (2017) used optimized techniques to analyze feature parameter values as the input features of the neural network stock trading system, and used genetic algorithms to optimize the RSI parameters of uptrend and downtrend market conditions. They used the optimized feature points as trigger points for buying and selling of the MLP data set, and used Dow 30 stocks to verify the model. In the experiment they used financial evaluation to access the strategy based on criteria (annualized return, annualized number of transactions, percentage of success, etc.). Experiment results show that the GA + DMLP strategy obtained 11.9% average annualized return, and percent of success is 71.63%. The GA-only strategy obtained 15.8% average annualized return, and percent of success is 70.8%. Krauss et al. (2017) proposed a statistical arbitrage strategy based on DNN, gradient-boosted-trees (GBT), random forests (RF) and different integrations. According to the strategy, the daily trading signals of the previous day are generated based on the probability of a stock outperforming the market. Then convert the stock with highest probability to long position and the stock with lowest probability to short position. They establish the set of k = 10 (a diversified portfolio with 10 long positions and 10 short positions) in the experiment, and found that out-of-sample returns exceeding 0.45 percent per day for k = 10, prior to transaction costs. The results show that the performance of RF processing feature space noise in the application is better than GBT and DNN, and equal weights The integrated approach also process a good performance. Wang et al. (2021) proposed a parallel network continuous quantitative trading model with Generalized Autoregressive Conditional Heteroskedasticity (GARCH) and proximal policy optimization (PPO). In order to match the deep learning algorithms, they formulate the stock trading process into a Markov Decision Process (MDP) and employ multi-frequency data (5 minutes, 1 day, GARCH and one week) of historical stock trading data as state space of MDP. The experiment compared 4 different DL methods based on PPO, including DNN, DNN-GARCH (DNN with GARCH), MCT (Multi-frequency continuous-share trading model) and MCTG (Multi-frequency continuous-share trading model with GARCH), The results show that MCTG and MCT have successively achieved higher returns than DNN, and DNN also achieved higher returns than the benchmark.
Other training target. Lv et al. (2019) used multiple machine learning methods and multiple deep learning methods to quantify stock data sets. They constructed 44 technical indicators as input features, and then used the forecast of the price trend of each stock as a trading signal to formulate a trading strategy and finally conduct a backtest. Experiments show that, compared with the traditional ML algorithm, the DNN model keeps better performance in considering transaction costs. Day et al. (2016) proposed the DMLP analysis methods to predict the stock price trend of financial news sources. In order to filter out ineffective news sources, they evaluated news sources based on return on investment (ROI). In the future 20 days situation, the ROI values of MoneyDJ performs best efficiency among the prediction models.
Table 3 shows the related algorithmic trading research using the DMLP method.
Publication | Data set | Period | Method | Main training target |
Dixon et al. (2017) | CME listed commodity and FX futures | 1991–2014 | DMLP | Prediction |
Sezer et al. (2017) | Stocks in Dow 30 | 1997–2017 | DMLP + genetic algorithms (GA) | Arbitrage |
Singh et al. (2017) | Stocks in NASDAQ | 2004–2015 | (2D)²PCA + DMLP | Prediction |
Ji et al. (2019) | Bitcoin price | 2011–2018 | DMLP, LSTM, CNN, ResNet | Prediction |
Krauss et al. (2017) | Stocks in the S & P500 | 1992–2015 | DMLP, RF, GBT | Arbitrage |
Wang et al. (2021) | 5 stocks in Chinese stock market | 2011–2020 | DMLP with GARCH, PPO | Arbitrage |
Lv et al. (2019) | 424 stocks in S & P500 and 185 stocks in CSI300 | 2010–2017 | DMLP, ML models | Trading signal |
Day et al. (2016) | News from NowNews, AppleDaily, LTN, MoneyDJ | 2013–2014 | DMLP | Filter data set |
Prediction. Chen et al. (2020) studied China's Shanghai and Shenzhen 300 stock index futures, establishing the corresponding prediction model by using CNN and Deep Belief Network (DBN). Through experimental comparison, the accuracy of the results obtained by using the CNN model alone is lower than the result of using CNN and DBN together. Xie et al. (2020) constructed a forecasting model that only predicts the rise and fall of the short-term price of stock index futures. They applied the data available at the current moment of stock index futures to predict the rise or fall of the closing price of stock index futures at the next moment. The results show that compared with the single hidden layer neural network, the deep learning model has improved overall prediction accuracy and rise and fall prediction accuracy. Hossain et al. (2018) proposed a stock price prediction model based on a deep recurrent neural network. The model integrates LSTM and Gated Recurrent Unit (GRU), which is essentially a hybrid model. In the research, firstly the input is passed to the LSTM network and the first-level prediction is generated by the LSTM. Then the output of the LSTM layer is passed to the GRU layer to obtain the final prediction.
Arbitrage. Liu et al. (2017) proposed a CNN-LSTM hybrid neural network model to analyze the quantitative strategy of the stock market. First of all, they used CNN to formulate a quantitative stock selection strategy for judging stock trends, and further, using LSTM to predict stock prices with high accuracy. The result shows that the annualized rate of return of the strategy based on CNN-LSTM neural network model is more twice the annualized rate of return than the BaH strategy based on the Benchmark index. At the same time, the maximum drawdowns of the CNN-LSTM neural network model are 34% and 54% of the maximum drawdowns of the basic Momentum strategy and Benchmark indicators, respectively. Li et al. (2019) proposed a new type of transaction agent based on deep reinforcement learning. They solved the noise problem of financial data by using stacked denoising autoencoders (SDAEs). They also used LSTM to extend value-based DQN and asynchronous advantage actor-critic (A3C). Through experimental comparison, the performance of the trading agent is better than the benchmark, and the A3C expansion algorithm generates more profits than the DQN expansion algorithm. Serrano et al. (2018) employed a combinational model which consists of DMLP, RL, and GA to construct an intelligent investment model with a new learning algorithm based on a genome model. They use reinforcement learning to make quick decisions. In addition, deep learning clusters provide identity and memory and deep learning management clusters make final strategic decisions. Finally genetic learning transmits the learned information to future generations. The results show that the GA algorithm has a minimum error and it was able to exactly encodes the deep learning management cluster. Jeong et al. (2019) proposed a trading system that can predict the number of stocks to be traded. They designed an automated system that combined RL and DNN by adding DNN to DQN to predict the number of stocks. In addition, they study various behavioral strategies that use Q-values and analyze those behavioral strategies that are beneficial to profit in the chaotic market. Finally, a transfer learning method is proposed to prevent overfitting caused by insufficient financial data. The results showed that the strategy to postpone buying or selling in a chaotic market could effectively control the situation. The strategy increases the total profit of the S & P500 by 18% and the KOSPI index by 24%. The EuroStoxx50 index increased by 49%. Deng et al. (2017) combined DNN with RL to build a new learning framework. In this framework, the DNN part automatically perceives dynamic market conditions for information feature learning, and then the RL part interacts with the DNN and makes transaction decisions to accumulate final rewards in an unknown environment. In addition, to reduce the uncertainty in the original time series, they extended fuzzy learning models to deep learning models.
Other training target. Théate et al. (2021) proposed Trading Deep Q-Network algorithm (TDQN), which is a solution based on deep reinforcement learning (DRL) to determine the best trading position at any point in stock market trading activities Algorithmic trading problem. They compared 4 benchmark strategies with TDRL and set evaluation indicators to access the performance of these strategies. In the experiment, according to the execution of trading position generated by TDQN, they obtained better performance results than benchmark strategies.
Other models-based studies are shown in Table 4.
Publication | Data set | Period | Method | Main training target |
Liu et al. (2017) | Chinese stocks from the SINA FINANCE web | 2007–2017 | CNN-LSTM | Arbitrage |
Chen et al. (2020) | Shanghai and Shenzhen 300 stock index | 2017–2018 | CNN + DBN | Prediction |
Li et al. (2019) | APPL, IBM, PG, ES, IF | 2008–2018 | LSTM, DQN SDAE, A3C |
Arbitrage |
Serrano et al. (2018) | 8 assets from Bond Market | – | RL, DMLP, GA | Arbitrage |
Théate et al. (2021) | 30 stocks with diverse characteristics | 2012–2019 | RL + DNN+ DQN | Long-short position |
Jeong et al. (2019) | S & P500, KOSPI, HIS and EuroStoxx50 | 2007–2017 | DNN + DQN | Arbitrage |
Deng et al. (2017) | Stock-IF contract | 2014–2015 | DMLP + RL | Arbitrage |
Xie et al. (2020) | Shanghai and Shenzhen 300 stock index futures | – | RBM, PCA, AE | Prediction |
Hossain et al. (2018) | S & P500 index | 1950–2016 | LSTM + GRU | Prediction |
Algorithmic trading needs to identify non-steady changing price signals, determine the entry and exit points of the transaction, and generate profitable financial transactions. Since algorithmic trading model and the data set have time characteristics, the above mentioned models are very suitable for this scenario. CNN-based models are more suitable for classification problems, and a specific work area of CNN is image recognition problems. Therefore, in algorithmic trading studies, researchers convert one-dimensional time series financial data into static two-dimensional image classes so that they can use the powerful functions of CNN. However, compared with CNN models, LSTM may capture and process time series data more effectively, due to their special architecture. LSTM has a perfect financial time series data prediction structure, and is suitable for the scenarios of algorithmic trading. In the paper, we review the current application of DMLP model in algorithmic trading. We summarize that DMLP is suitable for regression and classification problems. In addition, to obtain better stability, some preprocessing of the data may be required in DMLP. At the same time, it has attracted much attention because its core structure has a long history of being widely accepted in the deep learning field. Recently, deep reinforcement learning and genetic algorithms attract more and more researchers of algorithmic trading, and show the huge potential in algorithmic trading applications. In the survey, we try to evaluate the current state of research and propose our ideas on the relationship between deep learning models and algorithmic trading. This is also the research direction of our future work. In our opinion, there are some open issues for the algorithmic trading as follows.
Structural parameters optimization. Although the performance of many models is promising, even greater improvements can be achieved. If the structural parameters of the deep learning model can be improved, the model may perform better on algorithmic trading applications. Some articles initialize the parameters in the model according to their experience. However, due to different application scenarios and some interference, manually initialized parameters may add complexity to the training process. The further study is to use an effective computer technology to initialize the structural parameters and optimize the training model.
Feature parameter selection. Feature selection often plays an important role in model training results. Some literature is to be artificially selected appropriate technical features favored by traders for training (Gudelek, 2017; Sezer, 2018; Lin, 2018). However, there may be correlations between different features (Gunduz, 2017; Mudassir, 2020), which has an unhealthy impact on model performance. There are researches using deep learning analysis methods to predict stock prices for financial reports, news, trader comments and other investment information that carries human subjective consciousness. However, it is necessary to apply machine learning methods or deep learning methods to select and sort feature parameters, or even use feature-free model training.
Hybrid method. Currently, there are very few model methods combine information analysis and feature analysis, and the possible integration methods still need to be deeply analyzed. The hybrid method can even show better performance than a single model in some complex scenarios. To solve complex trading applications, the deep learning models need to be adaptable for different application scenarios. Therefore, establishing a diversified and accurate hybrid model to solve complex trading problems is worthy of further study.
Algorithmic trading security. With the rapid development of algorithmic trading, the cost of technology has declined, which has led to the widespread adoption of algorithmic trading in the financial market. Hendershott et al. (2011) found that algorithmic trading reduces transaction costs and increases the amount of information for quotes. Therefore, it will increase market liquidity, and lead to a couple of caveats. Financial markets show fragility to a large number of algorithmic trading systems. With advancing in the algorithmic trading, we should pay attention to the risks of algorithmic trading and prevent major financial accidents caused by algorithmic trading.
In this article, 35 papers on algorithmic trading applications based on deep learning methods since 2016 are reviewed in detail. Some deep learning techniques adopted massively in algorithmic trading are discussed, such as CNN, LSTM, DMLP, and some hybrid models. We make a brief introduction to the data set, model methods, evaluation criteria and experimental results in these existing studies. For the algorithmic trading applications, deep learning methods show better performance than machine learning methods in many evaluation indicators. At the same time, deep learning methods can process huge amount of data.
Algorithmic trading has been one of the most popular research objects in the financial industry for many years. With the introduction of deep learning technology, the research on algorithmic trading attracts a lot of attention, and many new research results have been produced. Recently, many innovative modeling methods and systems are presented. In this survey, we highlight the most advanced deep learning technology in the field of algorithmic trading, and try to encourage researchers who are interested in this field to continue exploring.
This work is supported by the Guangzhou Science and Technology Planning Project of China (No. 202102010395). Besides that, authors would like to thank the editor and anonymous reviewers for their patient and valuable comments on earlier versions of this paper.
All authors declare no conflicts of interest in this paper.
[1] |
Baek Y, Kim H (2018) ModAugNet: A new forecasting framework for stock market index value with an overfitting prevention LSTM module and a prediction LSTM module. Expert Syst Appl 113: 457–480. doi: 10.1016/j.eswa.2018.07.019
![]() |
[2] | Boehmer E, Fong K, Wu J (2012) International evidence on algorithmic trading. In AFA 2013 San Diego Meetings Paper. |
[3] |
Chen C, Zhang P, Liu Y, et al. (2020) Financial quantitative investment using convolutional neural network and deep learning technology. Neurocomputing 390: 384–390. doi: 10.1016/j.neucom.2019.09.092
![]() |
[4] | Chen J, Chen W, Huang C, et al. (2016) Financial Time-Series Data Analysis Using Deep Convolutional Neural Networks. In 2016 7th International Conference on Cloud Computing and Big Data (CCBD), 87–92. |
[5] | Chen K, Zhou Y, Dai F (2015) A LSTM-based method for stock returns prediction: A case study of China stock market. In 2015 IEEE International Conference on Big Data (Big Data), 2823–2824. |
[6] | Chen S, He H (2018) Stock Prediction Using Convolutional Neural Network. In IOP Conference Series: Materials Science and Engineering, 435: 012026. |
[7] | Chen Y, Chen W, Huang S (2018) Developing Arbitrage Strategy in High-frequency Pairs Trading with Filterbank CNN Algorithm. In 2018 IEEE International Conference on Agents (ICA), 113–116. |
[8] |
Cybenko G (1989) Approximation by superpositions of a sigmoidal function. Math Control Signals Syst 2: 303–314. doi: 10.1007/BF02551274
![]() |
[9] | Day M, Lee C (2016) Deep learning for financial sentiment analysis on finance news providers. In 2016 IEEE/ACM International Conference on Advances in Social Networks Analysis and Mining (ASONAM), 1127–1134. |
[10] |
Deng Y, Bao F, Kong Y, et al. (2017) Deep Direct Reinforcement Learning for Financial Signal Representation and Trading. IEEE Trans Neural Networks Learn Syst 28: 653–664. doi: 10.1109/TNNLS.2016.2522401
![]() |
[11] |
Dixon M, Klabjan D, Bang J (2017) Classification-based financial markets prediction using deep neural networks. Algorithmic Financ 6: 67–77. doi: 10.3233/AF-170176
![]() |
[12] | Doering J, Fairbank M, Markose S (2017) Convolutional neural networks applied to high-frequency market microstructure forecasting. In 2017 9th Computer Science and Electronic Engineering (CEEC), 31–36. |
[13] |
Fang Y, Chen J, Xue Z (2019) Research on quantitative investment strategies based on deep learning. Algorithms 12: 35. doi: 10.3390/a12020035
![]() |
[14] | Gudelek M, Boluk S, Ozbayoglu A (2017) A deep learning based stock trading model with 2-D CNN trend detection. In 2017 IEEE Symposium Series on Computational Intelligence (SSCI), 1–8. |
[15] |
Gunduz H, Yaslan Y, Cataltepe Z (2017) Intraday prediction of borsa Istanbul using convolutional neural networks and feature correlations. Knowl Based Syst 137: 138–148. doi: 10.1016/j.knosys.2017.09.023
![]() |
[16] |
Hendershott T, Jones C, Menkveld A (2011) Does algorithmic trading improve liquidity? J Financ 66: 1–33. doi: 10.1111/j.1540-6261.2010.01624.x
![]() |
[17] | Hendershott T, Riordan R (2009) Algorithmic trading and information. University of California, Berkeley. |
[18] |
Hinton G, Salakhutdinov R (2006) Reducing the Dimensionality of Data with Neural Networks. Science 313: 504–507. doi: 10.1126/science.1127647
![]() |
[19] |
Hochreiter S, Schmidhuber J (1997) Long Short-Term Memory. Neural Comput 9: 1735–1780. doi: 10.1162/neco.1997.9.8.1735
![]() |
[20] | Lee H, Grosse R, Ranganath R, et al. (2009) Convolutional deep belief networks for scalable unsupervised learning of hierarchical representations. In Proceedings of the 26th annual international conference on machine learning, 609–616. |
[21] |
Hoseinzade E, Haratizadeh S (2019) CNNpred: CNN-based stock market prediction using a diverse set of variables. Expert Syst Appl 129: 273–285. doi: 10.1016/j.eswa.2019.03.029
![]() |
[22] | Hossain M, Karim R, Thulasiram R, et al. (2018) Hybrid Deep Learning Model for Stock Price Prediction. In 2018 IEEE Symposium Series on Computational Intelligence (SSCI), 1837–1844. |
[23] |
Jeong G, Kim H (2019) Improving financial trading decisions using deep Q-learning: Predicting the number of shares, action strategies, and transfer learning. Expert Syst Appl 117: 125–138. doi: 10.1016/j.eswa.2018.09.036
![]() |
[24] |
Ji S, Kim J, Im H (2019) A Comparative Study of Bitcoin Price Prediction Using Deep Learning. Mathematics 7: 898. doi: 10.3390/math7100898
![]() |
[25] | Kalman B, Kwasny S (1992) Why tanh: choosing a sigmoidal function. In [Proceedings 1992] IJCNN International Joint Conference on Neural Networks, 4: 578–581. |
[26] | Kim S, Kang M (2019) Financial series prediction using Attention LSTM. arXiv preprint arXiv: 1902.10877. |
[27] |
Krauss C, Do X, Huck N (2017) Deep neural networks, gradient-boosted trees, random forests: Statistical arbitrage on the S & P 500. Eur J Oper Res 259: 689–702. doi: 10.1016/j.ejor.2016.10.031
![]() |
[28] |
LeCun Y, Bengio Y, Hinton G (2015) Deep learning. Nature 521: 436-44. doi: 10.1038/nature14539
![]() |
[29] |
Li Y, Zheng W, Zheng Z (2019) Deep Robust Reinforcement Learning for Practical Algorithmic Trading. IEEE Access 7: 108014–108022. doi: 10.1109/ACCESS.2019.2932789
![]() |
[30] | Lin B, Chu W, Wang C (2018) Application of Stock Analysis Using Deep Learning. In 2018 7th International Congress on Advanced Applied Informatics (ⅡAI-AAI), 612–617. |
[31] | Liu S, Zhang C, Ma J (2017) CNN-LSTM neural network model for quantitative strategy analysis in stock markets. In international conference on neural information processing, Springer, 198–206. |
[32] | Lu W, Li J, Li Y, et al. (2020) A CNN-LSTM-Based Model to Forecast Stock Prices. Complexity 2020: 6622927. |
[33] |
Luo S, Lin X, Zheng Z (2019) A novel CNN-DDPG based AI-trader: Performance and roles in business operations. Transp Res Part E Logist Transp Rev 131: 68–79. doi: 10.1016/j.tre.2019.09.013
![]() |
[34] | Lv D, Yuan S, Li M, et al. (2019) An Empirical Study of Machine Learning Algorithms for Stock Daily Trading Strategy. Math Probl Eng 2019: 7816154. |
[35] | Mikolov T, Karafiát M, Burget L, et al. (2010) Recurrent neural network based language model. In Interspeech, Makuhari, 1045–1048. |
[36] | Mudassir M, Bennbaia S, Unal D, et al. (2020) Time-series forecasting of Bitcoin prices using high-dimensional features: a machine learning approach. Neural Comput Appl 2020: 1–15. |
[37] | Nair V, Hinton G (2010) Rectified linear units improve restricted boltzmann machines. In Icml. |
[38] | Nelson D, Pereira A, de Oliveira R (2017) Stock market's price movement prediction with LSTM neural networks. In 2017 International Joint Conference on Neural Networks (IJCNN), 1419–1426. |
[39] |
Nuti G, Mirghaemi M, Treleaven P, et al. (2011) Algorithmic Trading. Computer 44: 61–69. doi: 10.1109/MC.2011.31
![]() |
[40] |
Ozbayoglu A, Gudelek M, Sezer O (2020) Deep learning for financial applications: A survey. Appl Soft Comput 93: 106384. doi: 10.1016/j.asoc.2020.106384
![]() |
[41] | Selvin S, Vinayakumar R, Gopalakrishnan E, et al. (2017) Stock price prediction using LSTM, RNN and CNN-sliding window model. In 2017 International Conference on Advances in Computing, Communications and Informatics (ICACCI), 1643–1647. |
[42] |
Serrano W (2018) Fintech Model: The Random Neural Network with Genetic Algorithm. Proced Comput Sci 126: 537–546. doi: 10.1016/j.procs.2018.07.288
![]() |
[43] |
Sezer O, Ozbayoglu M, Dogdu E (2017) A Deep Neural-Network Based Stock Trading System Based on Evolutionary Optimized Technical Analysis Parameters. Proced Comput Sci 114: 473–480. doi: 10.1016/j.procs.2017.09.031
![]() |
[44] |
Sezer O, Ozbayoglu A (2018) Algorithmic financial trading with deep convolutional neural networks: Time series to image conversion approach. Appl Soft Comput 70: 525–538. doi: 10.1016/j.asoc.2018.04.024
![]() |
[45] | Sezer O, Ozbayoglu A (2019) Financial trading model with stock bar chart image time series with deep convolutional neural networks. arXiv preprint arXiv: 1903.04610. |
[46] | Shah D, Campbell W, Zulkernine F (2018) A Comparative Study of LSTM and DNN for Stock Market Forecasting. In 2018 IEEE International Conference on Big Data (Big Data), 4148–4155. |
[47] |
Singh R, Srivastava S (2017) Stock prediction using deep learning. Multimed Tools Appl 76: 18569–18584. doi: 10.1007/s11042-016-4159-7
![]() |
[48] |
Sirignano J, Cont R (2019) Universal features of price formation in financial markets: perspectives from deep learning. Quant Financ 19: 1449–1459. doi: 10.1080/14697688.2019.1622295
![]() |
[49] |
Sohangir S, Wang D, Pomeranets A, et al. (2018) Big Data: Deep Learning for financial sentiment analysis. J Big Data 5: 1–25. doi: 10.1186/s40537-017-0111-6
![]() |
[50] | Sutskever I, Hinton G, Taylor G (2009) The recurrent temporal restricted boltzmann machine. In Advances in neural information processing systems, 1601–1608. |
[51] |
Théate T, Ernst D (2021) An application of deep reinforcement learning to algorithmic trading. Expert Syst Appl 173: 114632. doi: 10.1016/j.eswa.2021.114632
![]() |
[52] |
Treleaven P, Galas M, Lalchand V (2013) Algorithmic trading review. Commun ACM 56: 76–85. doi: 10.1145/2500117
![]() |
[53] |
Troiano L, Villa E, Loia V (2018) Replicating a Trading Strategy by Means of LSTM for Financial Industry Applications. IEEE Trans Ind Inf 14: 3226–3234. doi: 10.1109/TII.2018.2811377
![]() |
[54] | Wang Z, Lu W, Zhang K, et al. (2021) MCTG: Multi-frequency continuous-share trading algorithm with GARCH based on deep reinforcement learning. arXiv preprint arXiv: 2105.03625. |
[55] |
Xie M, Li H, Zhao Y (2020) Blockchain financial investment based on deep learning network algorithm. J Comput Appl Math 372: 112723. doi: 10.1016/j.cam.2020.112723
![]() |
[56] | Zhao Z, Rao R, Tu S, et al. (2017) Time-Weighted LSTM Model with Redefined Labeling for Stock Trend Prediction. In 2017 IEEE 29th International Conference on Tools with Artificial Intelligence (ICTAI), 1210–1217. |
[57] | Zou Z, Qu Z (2020) Using LSTM in Stock prediction and Quantitative Trading. CS230: Deep Learning, Winter. |
1. | Iromie K. Samarasekara, Oshan K. Mendis, Sapumal Ahangama, Ajantha S. Atukorale, 2022, Dynamic Stop-Loss Approach for Short Term Trades using Deep Learning, 978-1-6654-8045-1, 3537, 10.1109/BigData55660.2022.10020535 | |
2. | Fatima Tfaily, Mohamad M. Fouad, Multi-level stacking of LSTM recurrent models for predicting stock-market indices, 2022, 2, 2769-2140, 147, 10.3934/DSFE.2022007 | |
3. | Santosh Kumar Sahu, Anil Mokhade, Neeraj Dhanraj Bokde, An Overview of Machine Learning, Deep Learning, and Reinforcement Learning-Based Techniques in Quantitative Finance: Recent Progress and Challenges, 2023, 13, 2076-3417, 1956, 10.3390/app13031956 | |
4. | Zericho R. Marak, Anand J. Kulkarni, Sarthak Sengupta, 2024, Chapter 29, 978-981-97-3819-9, 577, 10.1007/978-981-97-3820-5_29 | |
5. | Zericho R. Marak, Anand J. Kulkarni, Sarthak Sengupta, 2024, Chapter 29-1, 978-981-19-8851-6, 1, 10.1007/978-981-19-8851-6_29-1 | |
6. | Yuling Huang, Chujin Zhou, Kai Cui, Xiaoping Lu, A multi-agent reinforcement learning framework for optimizing financial trading strategies based on TimesNet, 2024, 237, 09574174, 121502, 10.1016/j.eswa.2023.121502 | |
7. | D.M.D.K. Dasanayake, H.Y. Dilshan, H.D.K.Y. Rathnaweera, Sapumal Ahangama, Indika Perera, 2023, A Novel Approach for Deep Learning-Powered Forecasting of Market Bottoms in Cryptocurrency and Stock Trading, 979-8-3503-2445-7, 1481, 10.1109/BigData59044.2023.10386273 | |
8. | Chujin Zhou, Yuling Huang, Kai Cui, Xiaoping Lu, R-DDQN: Optimizing Algorithmic Trading Strategies Using a Reward Network in a Double DQN, 2024, 12, 2227-7390, 1621, 10.3390/math12111621 | |
9. | Mahdieh Ghotbi, Morteza Zahedi, Predicting price trends combining kinetic energy and deep reinforcement learning, 2024, 244, 09574174, 122994, 10.1016/j.eswa.2023.122994 | |
10. | Fayad Ali, Ravate Suryakant, Sunil Nimbore, 2023, Ensemble Model Based on Deep Learning for Forecasting Crypto Asset Futures in Markets, 979-8-3503-1912-5, 1, 10.1109/SMARTGENCON60755.2023.10442376 | |
11. | Nils Ole Breuer, Andreas Sauter, Majid Mohammadi, Erman Acar, 2024, Chapter 8, 978-3-031-63799-5, 143, 10.1007/978-3-031-63800-8_8 | |
12. | Oleksandr Naumov, Olha Naumova, Prospects for applying deep learning models in economics and finance, 2024, 2024, 24158453, 321, 10.36887/2415-8453-2024-3-57 | |
13. | D. Ramya, , Reinforcement Learning Driven Trading Algorithm with Optimized Stock Portfolio Management Scheme to Control Financial Risk, 2024, 6, 2661-8907, 10.1007/s42979-024-03555-0 | |
14. | Yuling Huang, Chujin Zhou, Lin Zhang, Xiaoping Lu, A Self-Rewarding Mechanism in Deep Reinforcement Learning for Trading Strategy Optimization, 2024, 12, 2227-7390, 4020, 10.3390/math12244020 | |
15. | D. O. Cardozo, B. Medina, C. Quintero, M. Pardo, Enhancing Solar Radiation Prediction for Computational-Constrained Environments Using Hybrid Artificial Neural Networks, 2024, 12, 2169-3536, 196382, 10.1109/ACCESS.2024.3521318 | |
16. | Hüseyin İşcan, Ayşe Durgun, Yapay Zekâ: Alt Dalları ve Uygulama Alanları, 2024, 16, 2687-3427, 201, 10.52791/aksarayiibd.1574207 | |
17. | MD Shahriar Mahmud Bhuiyan, MD AL Rafi, Gourab Nicholas Rodrigues, MD Nazmul Hossain Mir, Adit Ishraq, M.F. Mridha, Jungpil Shin, Deep learning for algorithmic trading: A systematic review of predictive models and optimization strategies, 2025, 26, 25900056, 100390, 10.1016/j.array.2025.100390 | |
18. | Mohammad Al Khaldy, Ahmad al-Qerem, Amjad Aldweesh, Mouhammd Alkasassbeh, Ammar Almomani, Mohammad Alauthman, 2025, chapter 24, 9798337312002, 499, 10.4018/979-8-3373-1200-2.ch024 | |
19. | Amina Bibi, Danish Shehzad, Ahsan Imtiaz, 2025, Chapter 2, 978-3-031-77616-8, 11, 10.1007/978-3-031-77617-5_2 |
Publication | Data set | Period | Method | Main training target |
Doering et al. (2017) | London stock exchange | 2007–2008 | CNN | Prediction |
Sezer et al. (2018) | ETFs and stocks in Dow 30 | 2007–2017 | CNN | Arbitrage |
Gudelek et al. (2017) | 17 ETFs | 2000–2017 | CNN | Prediction |
Selvin et al. (2017) | Stocks in Infosys, TCS, Cipla | 2014–2014 | CNN sliding-window | Prediction |
Chen et al. (2018) | Taiwan Stock Index Futures and Mini Index Futures | 2012–2014 | CNN + visualization method | Arbitrage |
Sezer et al. (2019) | Stocks in Dow 30 | 1997–2017 | CNN with feature imaging | Arbitrage |
Luo et al. (2019) | Stock-index future in China financial market | 2017–2018 | CNN with RL | Buy-sell-hold Signal |
Gunduz et al. (2017) | Borsa Istanbul 100 stocks | 2011–2015 | CNN, LR | Features correlation |
Hoseinzade et al. (2019) | Daily direction of the close of S & P 500 index, NASDAQ Composite, Dow Jones Industrial Average, NYSE Composite, and RUSSELL 2000 | 2010–2017 | CNN | Prediction |
Publication | Data set | Period | Method | Main training target |
Mudassir et al. (2020) | BTC features and price | 2013–2019 | LSTM, ANN, AVM, SANN | Prediction |
Fang et al. (2019) | Shanghai 50 ETF options | 2015–2018 | LSTM, RF, LSTM-SVRs | Arbitrage |
Troiano et al. (2018) | Dow30 stocks | 2012–2016 | LSTM | Long-short-hold position |
Lin et al. (2018) | Taiwan stock exchange corporation | – | LSTM, RNN | Prediction |
Baek et al. (2018) | Korea composite stock price index 200 and S & P500 | 2010–2016 | LSTM with prevention module, prediction module | Prediction |
Shah et al. (2018) | Indian BSE Sensex index and Tech Mahindra stock price | 1997–2017 | LSTM, DNN | Prediction |
Zhao et al. (2017) | Stock indexes in SSE, SSE50, CSI500, CSI300 and SZSE | 2002–2017 | LSTM | Prediction |
Nelson et al. (2017) | 5 stocks in Brazilian stock exchange | 2008–2015 | LSTM | Prediction |
Zou et al. (2020) | Stocks in SP500 and the accounting and corporate statistics for the stocks | 2004–2013 | LSTM, RNN, ARIMA | Arbitrage |
Publication | Data set | Period | Method | Main training target |
Dixon et al. (2017) | CME listed commodity and FX futures | 1991–2014 | DMLP | Prediction |
Sezer et al. (2017) | Stocks in Dow 30 | 1997–2017 | DMLP + genetic algorithms (GA) | Arbitrage |
Singh et al. (2017) | Stocks in NASDAQ | 2004–2015 | (2D)²PCA + DMLP | Prediction |
Ji et al. (2019) | Bitcoin price | 2011–2018 | DMLP, LSTM, CNN, ResNet | Prediction |
Krauss et al. (2017) | Stocks in the S & P500 | 1992–2015 | DMLP, RF, GBT | Arbitrage |
Wang et al. (2021) | 5 stocks in Chinese stock market | 2011–2020 | DMLP with GARCH, PPO | Arbitrage |
Lv et al. (2019) | 424 stocks in S & P500 and 185 stocks in CSI300 | 2010–2017 | DMLP, ML models | Trading signal |
Day et al. (2016) | News from NowNews, AppleDaily, LTN, MoneyDJ | 2013–2014 | DMLP | Filter data set |
Publication | Data set | Period | Method | Main training target |
Liu et al. (2017) | Chinese stocks from the SINA FINANCE web | 2007–2017 | CNN-LSTM | Arbitrage |
Chen et al. (2020) | Shanghai and Shenzhen 300 stock index | 2017–2018 | CNN + DBN | Prediction |
Li et al. (2019) | APPL, IBM, PG, ES, IF | 2008–2018 | LSTM, DQN SDAE, A3C |
Arbitrage |
Serrano et al. (2018) | 8 assets from Bond Market | – | RL, DMLP, GA | Arbitrage |
Théate et al. (2021) | 30 stocks with diverse characteristics | 2012–2019 | RL + DNN+ DQN | Long-short position |
Jeong et al. (2019) | S & P500, KOSPI, HIS and EuroStoxx50 | 2007–2017 | DNN + DQN | Arbitrage |
Deng et al. (2017) | Stock-IF contract | 2014–2015 | DMLP + RL | Arbitrage |
Xie et al. (2020) | Shanghai and Shenzhen 300 stock index futures | – | RBM, PCA, AE | Prediction |
Hossain et al. (2018) | S & P500 index | 1950–2016 | LSTM + GRU | Prediction |
Publication | Data set | Period | Method | Main training target |
Doering et al. (2017) | London stock exchange | 2007–2008 | CNN | Prediction |
Sezer et al. (2018) | ETFs and stocks in Dow 30 | 2007–2017 | CNN | Arbitrage |
Gudelek et al. (2017) | 17 ETFs | 2000–2017 | CNN | Prediction |
Selvin et al. (2017) | Stocks in Infosys, TCS, Cipla | 2014–2014 | CNN sliding-window | Prediction |
Chen et al. (2018) | Taiwan Stock Index Futures and Mini Index Futures | 2012–2014 | CNN + visualization method | Arbitrage |
Sezer et al. (2019) | Stocks in Dow 30 | 1997–2017 | CNN with feature imaging | Arbitrage |
Luo et al. (2019) | Stock-index future in China financial market | 2017–2018 | CNN with RL | Buy-sell-hold Signal |
Gunduz et al. (2017) | Borsa Istanbul 100 stocks | 2011–2015 | CNN, LR | Features correlation |
Hoseinzade et al. (2019) | Daily direction of the close of S & P 500 index, NASDAQ Composite, Dow Jones Industrial Average, NYSE Composite, and RUSSELL 2000 | 2010–2017 | CNN | Prediction |
Publication | Data set | Period | Method | Main training target |
Mudassir et al. (2020) | BTC features and price | 2013–2019 | LSTM, ANN, AVM, SANN | Prediction |
Fang et al. (2019) | Shanghai 50 ETF options | 2015–2018 | LSTM, RF, LSTM-SVRs | Arbitrage |
Troiano et al. (2018) | Dow30 stocks | 2012–2016 | LSTM | Long-short-hold position |
Lin et al. (2018) | Taiwan stock exchange corporation | – | LSTM, RNN | Prediction |
Baek et al. (2018) | Korea composite stock price index 200 and S & P500 | 2010–2016 | LSTM with prevention module, prediction module | Prediction |
Shah et al. (2018) | Indian BSE Sensex index and Tech Mahindra stock price | 1997–2017 | LSTM, DNN | Prediction |
Zhao et al. (2017) | Stock indexes in SSE, SSE50, CSI500, CSI300 and SZSE | 2002–2017 | LSTM | Prediction |
Nelson et al. (2017) | 5 stocks in Brazilian stock exchange | 2008–2015 | LSTM | Prediction |
Zou et al. (2020) | Stocks in SP500 and the accounting and corporate statistics for the stocks | 2004–2013 | LSTM, RNN, ARIMA | Arbitrage |
Publication | Data set | Period | Method | Main training target |
Dixon et al. (2017) | CME listed commodity and FX futures | 1991–2014 | DMLP | Prediction |
Sezer et al. (2017) | Stocks in Dow 30 | 1997–2017 | DMLP + genetic algorithms (GA) | Arbitrage |
Singh et al. (2017) | Stocks in NASDAQ | 2004–2015 | (2D)²PCA + DMLP | Prediction |
Ji et al. (2019) | Bitcoin price | 2011–2018 | DMLP, LSTM, CNN, ResNet | Prediction |
Krauss et al. (2017) | Stocks in the S & P500 | 1992–2015 | DMLP, RF, GBT | Arbitrage |
Wang et al. (2021) | 5 stocks in Chinese stock market | 2011–2020 | DMLP with GARCH, PPO | Arbitrage |
Lv et al. (2019) | 424 stocks in S & P500 and 185 stocks in CSI300 | 2010–2017 | DMLP, ML models | Trading signal |
Day et al. (2016) | News from NowNews, AppleDaily, LTN, MoneyDJ | 2013–2014 | DMLP | Filter data set |
Publication | Data set | Period | Method | Main training target |
Liu et al. (2017) | Chinese stocks from the SINA FINANCE web | 2007–2017 | CNN-LSTM | Arbitrage |
Chen et al. (2020) | Shanghai and Shenzhen 300 stock index | 2017–2018 | CNN + DBN | Prediction |
Li et al. (2019) | APPL, IBM, PG, ES, IF | 2008–2018 | LSTM, DQN SDAE, A3C |
Arbitrage |
Serrano et al. (2018) | 8 assets from Bond Market | – | RL, DMLP, GA | Arbitrage |
Théate et al. (2021) | 30 stocks with diverse characteristics | 2012–2019 | RL + DNN+ DQN | Long-short position |
Jeong et al. (2019) | S & P500, KOSPI, HIS and EuroStoxx50 | 2007–2017 | DNN + DQN | Arbitrage |
Deng et al. (2017) | Stock-IF contract | 2014–2015 | DMLP + RL | Arbitrage |
Xie et al. (2020) | Shanghai and Shenzhen 300 stock index futures | – | RBM, PCA, AE | Prediction |
Hossain et al. (2018) | S & P500 index | 1950–2016 | LSTM + GRU | Prediction |