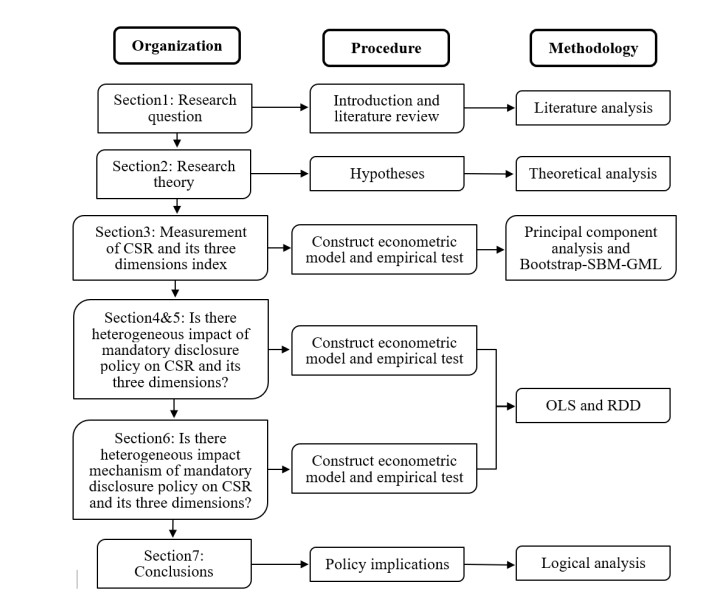
The corporate social responsibility (CSR) report is an important carrier of non-financial information disclosure of enterprises and an important bridge of communication between enterprises and interested parties. Compulsory disclosure has promoted the improvement of CSR levels to some extent. While, for interested parties, their attention to various dimensions of CSR has significant differences, which leads to the heterogeneous impact of mandatory disclosure policy on its different dimensions. Through regression discontinuity design model (RDD), as well as using quasi-natural experiments of Chinese mandatory disclosure policies issued in 2008, we are going to get the following conclusions by analyzing the heterogeneous impact of mandatory disclosure on CSR with the environment (CER), social (SOC) and economic (ECO) three-dimension on the basis of verifying that mandatory disclosure policy has a positive impact on CSR. (1) The effects of mandatory disclosure on the three dimensions of CSR are heterogeneous, that is, the significant effects and directions are significantly different in the three dimensions. (2) The heterogeneity of mandatory disclosure on CSR is reflected in the changing trend, and there is no significant difference at the turning point of the trend. (3) The heterogeneity of the impact mechanism of mandatory disclosure on CSR is reflected in the different mediating variables of policy on different dimensions impact, that is, the mediating variables of CER and ECO are the environmental disclosure information and return on assets. (4) The impact of mandatory disclosure on different dimensions of CSR is heterogeneous when the nature of industries and property rights are different.
Citation: Shuanglian Chen, Cunyi Yang, Khaldoon Albitar. Is there any heterogeneous impact of mandatory disclosure on corporate social responsibility dimensions? Evidence from a quasi-natural experiment in China[J]. Data Science in Finance and Economics, 2021, 1(3): 272-297. doi: 10.3934/DSFE.2021015
[1] | Matthew Ki, Junfeng Shang . Prediction of minimum wages for countries with random forests and neural networks. Data Science in Finance and Economics, 2024, 4(2): 309-332. doi: 10.3934/DSFE.2024013 |
[2] | Lu Liu, Ming Liu . How does the digital economy affect industrial eco-efficiency? Empirical evidence from China. Data Science in Finance and Economics, 2022, 2(4): 371-390. doi: 10.3934/DSFE.2022019 |
[3] | Jiamin Cheng, Yuanying Jiang . How can carbon markets drive the development of renewable energy sector? Empirical evidence from China. Data Science in Finance and Economics, 2024, 4(2): 249-269. doi: 10.3934/DSFE.2024010 |
[4] | Zimei Huang, Zhenghui Li . What reflects investor sentiment? Empirical evidence from China. Data Science in Finance and Economics, 2021, 1(3): 235-252. doi: 10.3934/DSFE.2021013 |
[5] | Ji Young Choi, Chae Young Lee, Man-Suk Oh . Discovering causal relationships among financial variables associated with firm value using a dynamic Bayesian network. Data Science in Finance and Economics, 2025, 5(1): 1-18. doi: 10.3934/DSFE.2025001 |
[6] | Dachen Sheng, Opale Guyot . Market power, internal and external monitoring, and firm distress in the Chinese market. Data Science in Finance and Economics, 2024, 4(2): 285-308. doi: 10.3934/DSFE.2024012 |
[7] | Yi Chen, Zhehao Huang . Measuring the effects of investor attention on China's stock returns. Data Science in Finance and Economics, 2021, 1(4): 327-344. doi: 10.3934/DSFE.2021018 |
[8] | Fangzhou Huang, Jiao Song, Nick J. Taylor . The impact of time-varying risk on stock returns: an experiment of cubic piecewise polynomial function model and the Fourier Flexible Form model. Data Science in Finance and Economics, 2021, 1(2): 141-164. doi: 10.3934/DSFE.2021008 |
[9] | Angelica Mcwera, Jules Clement Mba . Predicting stock market direction in South African banking sector using ensemble machine learning techniques. Data Science in Finance and Economics, 2023, 3(4): 401-426. doi: 10.3934/DSFE.2023023 |
[10] | Aihua Li, Qinyan Wei, Yong Shi, Zhidong Liu . Research on stock price prediction from a data fusion perspective. Data Science in Finance and Economics, 2023, 3(3): 230-250. doi: 10.3934/DSFE.2023014 |
The corporate social responsibility (CSR) report is an important carrier of non-financial information disclosure of enterprises and an important bridge of communication between enterprises and interested parties. Compulsory disclosure has promoted the improvement of CSR levels to some extent. While, for interested parties, their attention to various dimensions of CSR has significant differences, which leads to the heterogeneous impact of mandatory disclosure policy on its different dimensions. Through regression discontinuity design model (RDD), as well as using quasi-natural experiments of Chinese mandatory disclosure policies issued in 2008, we are going to get the following conclusions by analyzing the heterogeneous impact of mandatory disclosure on CSR with the environment (CER), social (SOC) and economic (ECO) three-dimension on the basis of verifying that mandatory disclosure policy has a positive impact on CSR. (1) The effects of mandatory disclosure on the three dimensions of CSR are heterogeneous, that is, the significant effects and directions are significantly different in the three dimensions. (2) The heterogeneity of mandatory disclosure on CSR is reflected in the changing trend, and there is no significant difference at the turning point of the trend. (3) The heterogeneity of the impact mechanism of mandatory disclosure on CSR is reflected in the different mediating variables of policy on different dimensions impact, that is, the mediating variables of CER and ECO are the environmental disclosure information and return on assets. (4) The impact of mandatory disclosure on different dimensions of CSR is heterogeneous when the nature of industries and property rights are different.
Corporate social responsibility (CSR) report is an important source of non-financial information disclosure for enterprises. Voluntary and mandatory disclosure policies have different effects on CSR practices. Governments in different countries (regions) have different opinions on whether CSR reports should be voluntary or compulsory, so they have different requirements related to CSR disclosures. According to Dhaliwal et al. (2014), there are 22 countries that implement voluntary disclosure policies of CSR, and only nine countries implement mandatory disclosure policies. Since 2007, Asian countries have implemented voluntary disclosure policies of CSR without formal guidance in parallel with incentives and requirements (Arena et al., 2018). In China, before 2007, less than 3% of the listed companies had social responsibility reports. In May 2008 Shanghai stock exchange has published a notice on strengthening the social responsibility and guidelines on environmental information disclosure for listed enterprises. Then, in Dec 2008, CSR reports were forced to be released by enterprises listed on Shanghai Stock Exchange, financial enterprises, enterprises listed in foreign capital stocks and enterprises listed on Shenzhen Stock Exchange. Previous studies have shown that enterprises who voluntarily provide CSR information have difficulties informing investors and other stakeholders with reliable activities and CSR performance because these information might be used as "green washing" (Mobus, 2005). The aim of compulsory disclosure policy is to actively enhance enterprises to undertake their social responsibility, improve the level and quality of social responsibility information disclosure, and facilitate the sustainable development of the environment, society and economy. However, enterprises have flexible choices in taking social responsibility. According to previous research, CSR activities have different influences due to the different factors, such as enterprise size (Scott et al., 1987), asset-liability ratio, the profitability of the previous year, heavily polluting industry or not, corporate governance and so on. Hemingway and Maclagan found that CSR reports that are voluntarily published to stakeholders have some problems such as lacking standardization, caring too much about formalization, not paying enough attention to the essence, reporting good news rather than bad news, conflict says and acts (Hemingway and Maclagan, 2004). Kim et al. (2012) indicated that enterprises with better CSR performance pay more attention to ethical issues and offer higher quality financial reports. Dhaliwal et al.(2011) and Ghoul et al.(2011) respectively concluded that enterprises with low cost of equity have better CSR disclosure and CSR performance.
Generally speaking, CSR involves three dimensions: environmental, social and economic and those are related to the sustainable development of society. Different studies have looked at different CSR dimensions. Carroll (1979) divided CSR into economic responsibility, legal responsibility, ethical responsibility and charitable responsibility according to attributes of responsibility. Clarkson (1995) has declared that the evaluation model of corporate social performance should be established based on stakeholder management framework; besides, enterprises only need to deal with stakeholders problems but not social problems because they are not the government or charities. In 2006, the Research Institute of Private Economics of Peking University published a Survey and Evaluation System and Standards of Corporate Social Responsibility in China, which divided the main indicators of CSR into rights and interests for shareholders and employees, social economy, legal responsibility, honest management, public welfare responsibility and environmental protection. No matter how to divide CSR dimensions, they are all based on the content of SA8000: Social Accountability 8000 (SA8000), SA8000 is the standard system of social responsibility established by regulations of the International Labor Organization. The core contents of this standard system include three aspects: the first one is protecting resources and the environment through technology and other means to achieve sustainable development. The second one is achieving social stability with the development of social undertakings, and the third is solving employment problems by incentivizing and creating more value. Based on the above, we focus on the CSR disclosure of listed enterprises by using the quasi-natural experiment as well as considering the three aspects of the content of 2008 notice on improving annual reports of listed enterprises in China Shanghai stock exchange, which are promoting the sustainable development of environment and ecology, promoting sustainable social development, promoting sustainable economic development. Therefore, we divide CSR into environmental, social and economic three dimensions in our empirical research.
When implementing mandatory disclosure of CSR, enterprises make decisions among different CSR dimensions according to the relevance degree between each dimension and their own interests. In the study of environmental dimension, Albertini (2014) indicated that technology and precision of environmental information for French enterprises has been enhanced under disclosure pressure. Federica et al. (2020) declared that the endorsement and implementation of the mandatory disclosure policy in Italy have improved the effectiveness and the quality of environmental information. In the study of the social dimension, Sánchez (2000) found that enterprises have obtained goodwill and trust from the government through reporting charitable donations. Some executives intend to improve their social image and status by demonstrating social responsibility (Atkinson and Galaskiewicz, 1988; Galaskiewicz, 1997; Waller and Lanis, 2009). In the study of the economic dimension, some proposed the communication effect and proved that the implementation of CSR mandatory information disclosure could improve the efficiency of capital allocation of listed companies (Fama and Laffer, 1972; Verrecchia, 2001; Kim et al., 2014). Besides, the social responsibility disclosure of listed companies is negatively correlated with the risk of stock price crash. For the greenwashing effect, social responsibility disclosure can be used by enterprises to cover up problems, and it is also agreed that social responsibility disclosure is positively related to the risk of stock price crash (Hemingway and Maclagan, 2004; Petrovits, 2005; Prior et al., 2008; Lin et al., 2015; Chen et al., 2018). From above, the effects of mandatory disclosure policies are usually analysed in a single industry, from a single dimension or even one perspective, such as the analysis between stock price and environmental information disclosure, which lack generalisation. Therefore, a comprehensive study on the impact of mandatory disclosure policy on different dimensions of CSR is crucial.
Our study makes three contributions to the existing literature on mandatory CSR disclosure as follows: First, based on the existing literature on linking the goals of enterprise' own development and sustainable social development, we define CSR and divide it into environmental, social and economic dimensions as well as to measure each dimension and CSR index by applying a comprehensive evaluation method. Second, we explore the differences in the impact and time effect of mandatory disclosure policy on various dimensions of CSR. Based on the regression discontinuity (RDD) model and robustness test such as bandwidth setting, we test the positive skipping impact of mandatory disclosure on the dimension of corporate environmental responsibility, which continuously increases over time in terms of a time trend. We find that compulsory CSR disclosure has no significant impact on the social dimension. Compulsory CSR disclosure has a certain inhibitory effect on the economic dimension, and the inhibitory degree strengthens at the beginning and then weakens with the time trend. Third, we find that there is heterogeneity in the impact mechanism of mandatory disclosure on the three CSR dimensions. On the one hand, the heterogeneity in the impact of mandatory disclosure policy on CSR different dimensions is reflected in the different mediating variables. The mediating variables of environmental and economic dimensions are the disclosure of environmental information and return on assets. On the other hand, the effect of mandatory disclosure on different dimensions of CSR is heterogeneous in different industries and property rights, which means that mandatory disclosure policy has a better effect on non-heavily polluting industries and non-state-owned enterprises in the three dimensions.
Figure 1 shows the research framework of this study.
The rest part of this paper is arranged as follows: Section 2 presents hypotheses development. Section 3 contains research design, including model setting, the measurement for variables and data source; Section 4 analyses the empirical results of the impact of mandatory disclosure policy on various dimensions of CSR with parameters estimates. Section 5 shows the robustness test of the empirical results with bandwidth, breakpoint and algorithm selection. Section 6 explores the heterogeneity of the impact of mandatory disclosure policy on various dimensions of CSR. Section 7 presents the conclusion.
Compulsory disclosure of CSR reduces information asymmetry levels. The improvement of the information disclosure environment affects the behavior of enterprises to fulfill their social and environmental responsibilities. By promoting responsibilities fulfillment, enterprises can realise the coordinated development of their own and social value. Simultaneously, each dimension of CSR has a different impact on enterprise value. To undertake environmental responsibility, enterprises need to invest more in R & D and innovation as well as emission reduction, etc. (Hong et al., 2020; Utomo et al., 2020). In the absence of external impetus, the uncertainty of the effect of R & D and innovation, and the external effect of environmental impact make enterprises pay more attention to their own value at the expense of environmental responsibility. The mandatory disclosure of CSR, especially information related to the environmental dimension, has an even stronger positive effect on enterprises. The social dimension of CSR involves employee welfare, product reputation, etc., which gives enterprises motivation to improve various elements to a certain extent (Sánchez, 2000; Waller and Lanis, 2009; Hung, 2013). Under these circumstances, mandatory disclosure of CSR will not have a significant impact on the social dimension. The economic dimension of CSR is mainly in favor of creating more value and solving employment problems. To some extent, the economic dimension is consistent with the enterprise value goal, but the negative impact of enterprises' value in the short-term from mandatory CSR disclosure has two reasons: on the one hand, the environmental dimension squeegees out the economic dimension and thus mandatory CSR disclosure will make enterprises invest more in technological innovation for mid-long term strategy. Therefore, it will squeeze out the pursuit of economic value in the short term (Fogler and Nutt, 1975; Christainsen and Haveman, 1981). On the other hand, in the process of performing CSR, the effect of communication and information asymmetry can cause the uncertainty of mandatory disclosure. Therefore, it is reasonable to hypothesize that:
Hypothesis 1 (H1): Compulsory disclosure of CSR has a heterogeneous impact on the different CSR dimensions.
Enterprises make decisions among different dimensions according to the relevance degree between each dimension and their own interests during mandatory disclosure of CSR. As independent decision-making, the enterprise's cognitive decision-making process in all dimensions is consistent when making decisions on mandatory CSR disclosure. Therefore, the impact of mandatory disclosure on each dimension, as well as their trends is heterogeneous, but the time of turning point of the trend in each dimension is consistent. Based on this, we can develop the following hypothesis:
Hypothesis 2 (H2): Compulsory disclosure of CSR has a consistent impact on the turning point of CSR dimensions, but the impact trend is heterogeneous.
The impact mechanism of mandatory disclosure on each dimension of CSR is heterogeneous, which comes from the heterogeneous mediating effect. From the environmental dimension, in recent years, the number of corporate social responsibility reports disclosed in China has risen. Listed companies constitute the main force in the release of corporate social responsibility reports in China. Some enterprises have gradually deviated from the negative attitude towards the disclosure of social responsibility reports and disclosed the efforts made by companies in environmental protection to varying degrees, including environmental investment and environmental technology development, company's pollutant emission, waste gas, solid waste and waste residue recycling, and the specific results of energy conservation and emission reduction. Environmental restriction intensity and other related information disclosure play a significant mediating role in the impact because mandatory disclosure has mandatory requirements on environmental information, and it is promoted by enterprises' environmental responsibility. According to the principal-agent theory, there are mainly agency relationships between shareholders and managers in China's listed companies and between controlling shareholders and small and medium-sized shareholders. Managers and controlling shareholders are collectively referred to as company insiders. Insiders grasp company information that outsiders do not understand through operation and management rights and discourse rights, resulting in information asymmetry. According to the theory of information asymmetry, the party with less information is easily in a disadvantageous position and produces adverse selection. From the CSR social dimension, after introducing the mandatory social responsibility disclosure policy, the companies subject to mandatory disclosure disclosed their social responsibility reports, which forced the companies to provide more social welfare. Therefore, the social welfare expenditure of enterprises can be used as an intermediary variable of the social dimension. From the perspective of the CSR economy, if the company bears too much social responsibility in the report, investors will think that the company's economic value has decreased. Rational investors do not care about the commitment of social responsibility but think that the company's value has been lost. And enterprise's return on assets may act as mediating variable because there is a strong correlation between the economy and its value.
Simultaneously, due to the difference in the degree of environmental pollution in different industries, the cost of performing CSR is different, and the impact on each dimension is significantly different. Furthermore, because of the difference in property rights characteristics, the balance point between enterprise value and CSR in enterprise goals is different, so the impact of mandatory CSR disclosure on various dimensions is also significantly different. To sum up, we formulate the following hypothesis:
Hypothesis 3 (H3): Compulsory disclosure of CSR has heterogeneity impact mechanism on CSR dimensions of enterprise, and that is manifested in the heterogeneity of mediating effect and enterprise attributes.
Based on the hypotheses above, a baseline regression model with dummy variables is provided to verify the effects of dummy variables and covariates on CSR and its dimensions (Tuzcuoglu, 2020). The basic form of the benchmark regression model is as follows:
CSRit=λ+λ1∗PLt+λ2∗Xit+πi+εit | (1) |
CERit=δ+δ1∗PLt+δ2∗Xit+πi+ϵit | (2) |
SOCit=α+α1∗PLt+α2∗Xit+πi+θit | (3) |
ECOit=β+β1∗PLt+β2∗Xit+πi+ϑit | (4) |
PLt={1,Yt≥00,Yt<0 |
In Formula (1) to (4), Yt represents the year difference; i denotes the enterprise individual; t is time; PLt is the processing variable, that is, when the time is after 2008, the value is 1; otherwise, it is 0; CER is the environmental dimension index; SOC is the social dimension index, ECO is the economic dimension index. X represents a series of covariables that affecting CSR or other corresponding dimension levels, such as enterprise size, capital-labor density, fixed asset ratio, property character, corporate date, asset-liability ratio and Tobin's Q index according to Feng et al. (2020); πi is the individual fixed effect; εit et al. is the standard error term of clustering with enterprises.
Based on the benchmark model, the regression discontinuity model is used to test the policy effect of mandatory disclosure of CSR to investigate the impact of quasi-natural experiments further. Regression discontinuity, next to random experiment, can be effectively used to analyze the causal relationship between mandatory disclosure policy and various dimensions of CSR with realistic constraints. Besides, it also can avoid the endogenous problem of parameter estimation during policy analysis when the randomized experiments are not available, so as to really reflect the cause-and-effect between policy and various dimensions of CSR, and the jump effect brought by policy can be used to estimate the cause-and-effect between them as well. The regression discontinuity analysis principle assumes breakpoint factors from policy implementation and the corresponding breakpoint relationship. When the breakpoint variable does not reach its value, the research object will not be affected by the breakpoint function, while when it exceeds or equals the critical value, it does have an effect. Based on the nature of mandatory disclosure policy, we explore the impact of mandatory CSR disclosure policy on the whole and all dimensions of CSR with regression analysis method of precise breakpoint; that is, we analyze the probability change of individuals affected by the breakpoint function directly from 0 to 1 at the breakpoint. In this paper, the CSR index is used to measure the overall level. If a "jump" of CSR at the "breakpoint" can be observed, this "jump" can be attributed to policy implementation; that is, mandatory disclosure has an impact on CSR. In the empirical process of RDD, the samples affected by the policy are the experimental group, while the samples not affected by the policy are the control group. Therefore, RDD is regarded as a quasi-natural experiment similar to DID. We build the following model according to the research of Lee and Lemieux (Lee and Lemieux, 2010; Li et al., 2021):
CSRit=λ0+λ1∗PLt+λ2∗f(Yt)+λ∗Xit+πi+εit | (5) |
CERit=δ0+δ1∗PLt+δ2∗f(Yt)+δ∗Xit+πi+ϵit | (6) |
SOCit=α0+α1∗PLt+α2∗f(Yt)+α∗Xit+πi+θit | (7) |
ECOit=β0+β1∗PLt+β2∗f(Yt)+β∗Xit+πi+ϑit | (8) |
PLt={1,Yt≥00,Yt<0 |
In Formula (5) to (8), f(Yt) is the implementation of variable polynomial adjustment function; about polynomial-time t, although the higher polynomial regression is usually used for breakpoint in previous literature, but Gelman and Imbens tested that low-order polynomial is tended to have better regression results and reduce the order of the polynomial function used for control in the case of small time-bandwidth (Angrist and Pischke, 2008; Gelman and Imbens, 2019). In view of the samples, we find that the policy effects of third-order polynomials are not significant in each dimension in experiments, so we just take the first-order and second-order polynomials regression. πi is the individual fixed effect. λ1, δ1, α1 and β1 represent the impact of mandatory disclosure on CSR and the three dimensions of environment, society and economy, who are the important coefficients in this research.
Chinese A-share listed companies who were forced to disclose their CSR in 2008 are taken as initial study samples. In order to realize regression discontinuity, we only consider the sample data of 4 years before and after the policy impact (the annual data of listed companies from 2004 to 2012) with data availability. In order to meet the basic requirements of RDD, we exclude the data in 2008 to ensure that the period length around the breakpoint is the same. It should be noted that, in fact, data up to 2020 can be publicly obtained in this paper. Still, due to the limitations of the RDD method, the actual amount of data involved in regression needs to be consistent around the breakpoint, so it is meaningless to include data after 2012 in this paper. On this basis, the enterprises with ST/ST* during the investigation period from Jan. 2004 to Dec. 2012 are eliminated according to standard practice. In addition, we take financial enterprises into consideration of mandatory disclosure policy targets. Moreover, the listed companies after January 1, 2004 are also deleted because they did not have the data of 2004. After the above processing, we finally got 257 samples for this empirical study. The financial, legal and disciplinary data of sample enterprises are from the Wind and CSMAR database; Data of environmental indicators is collected manually from annual reports and CSR reports with content analysis method. Data processing is performed by the software of SPSS24.0, Stata16 and Maxdea Ultra 8.20.
For covariates, this paper selects six covariates that may influence the core variables and have a weak overlap with the core variables: Logarithm of firm size (linta); Capital labor density (cd); Fixed assets ratio (fr); Property right character (owner, 1 for state-owned enterprises and 0 for non-state-owned enterprises); Date of incorporation (age); Asset-liability ratio (dar); Tobin's Q index (tq).
The descriptive statistics of the variables are shown in Table1. The values in brackets are standard deviations. Since the data of 2008 are excluded, the mean value of three-dimensional index in descriptive statistics is not 0.
Variables | Unit | Summary | Before Mandatory Disclosure | After Mandatory Disclosure |
CSR | - | −0.096 (1.954) | −0.941 (1.075) | 0.747 (2.248) |
CER | - | −0.088 (0.982) | −0.756 (0.531) | 0.579 (0.868) |
SOC | - | −0.010 (1.035) | −0.165 (0.404) | 0.143 (1.390) |
ECO | - | 0.002 (1.004) | −0.019 (0.710) | 0.024 (1.231) |
lnta | - | 8.874 (1.393) | 8.400 (1.241) | 9.349 (1.377) |
cd | 10 million ¥/employee | 0.159 (1.002) | 0.198 (1.317) | 0.121 (0.520) |
fr | percentage | 0.289 (0.201) | 0.316 (0.205) | 0.262 (0.195) |
owner | - | 0.789 (0.407) | 0.796 (0.403) | 0.784 (0.411) |
age | year | 13.482 (4.650) | 10.983 (3.922) | 15.982 (3.922) |
dar | percentage | 0.512 (0.179) | 0.487 (0.177) | 0.537 (0.178) |
tq | - | 1.669 (0.988) | 1.539 (0.849) | 1.799 (1.096) |
The core variables in Table 1 include CSR and its indices of each dimension. These indices are the index values obtained from the comprehensive evaluation method. Although the methods are consistent, the index measurement of each part needs special elaboration for the difference of indicators connotation of each dimension and the distribution of original index data.
According to Li et al. (2020), five dimensions, such as environmental legal awareness, environmental social assessment, environment-friendly output, low-carbon technology and green management, can be evaluated environmental index of CSR. Among these dimensions, the legal consciousness is mainly decided by the awareness to comply with the environmental protection related laws and regulations, namely, its index set by environmental punishment. The environmental social assessment comes from the environmental protecting reputation, which is reflected by two indicators of environmental recognition and advantages. The environment-friendly output depends on environmental protection to enterprise's activities production and operation, which consists of three indicators of circular economy, environment-friendly products and pollution emissions. The low carbon technology mainly depends on energy saving or wastes reducing measures. The green management reflects the impact of enterprise management on the environment; its detection index is third-party inspection and green office is being adopted. In this research, we use the method of content analysis to quantitatively measure the environmental dimension level of CSR (Hardy and Schwartz, 1996), which is used to determine the score of each specific project through analysis of various published reports for enterprises and then obtain its overall evaluation. This method is often used in social responsibility and environmental information research because its qualitative description can be quantified for further research. By collecting relevant data, we analyze 2313 annual reports and disclosed social responsibility reports of sample enterprises in the corresponding years, as well as search and classify special fields for each index. Table 2 shows the weight of each part of the CER index measured by principal component analysis (Gallego-Álvarez et al., 2018).
Primary Indicator | Secondary Indicator | Tertiary Indicator | Weight |
CER index | Law sense (0.1762) | Environmental penalties | 0.1762 |
Social evaluation (0.2228) | Environmental commendation | 0.0448 | |
Environmental advantages | 0.1779 | ||
Environment-friendly outputs (0.1957) | Circular economy | 0.0458 | |
Environment-friendly products | 0.0652 | ||
Pollutant discharge | 0.0847 | ||
Low-carbon technology (0.1357) | Energy saving | 0.0574 | |
Reduce three wastes measures | 0.0782 | ||
Green management (0.2696) | Environmental qualification | 0.0505 | |
Green office | 0.2192 |
The score of CER can be calculated according to the original data of weights and indicators in Table 2.
The measurement of social (SOC) and economic (ECO) dimension index of CSR consists of two aspects: on the one hand, we calculate the involving related numerical variables; On the other hand, we analyze two dimensions of input and output from involving variables of social and economic. Based on these, the social and economic dimensions of CSR are analyzed by adopting data envelopment analysis (DEA) (Demirtas and Kececi, 2020). Data envelop analysis (DEA) was proposed in 1978 by Charnes et al. (1978), which is used to evaluate the relative efficiency of a group of decision-making units with multiple inputs and outputs. The basic models are CCR and BCC models, but they do not take factor "slack" into account. In order to deal with this phenomenon, Tone (2001) proposed the SBM model in 2001. The productivity index of Malmquist is used to study productivity changes and is popular in the efficiency analysis of panel data. The Malmquist-Luenberger (ML) index supports the analysis of the undesired output by introducing the directional distance function that contains the undesired output into the Malmquist index. To facilitate intertemporal comparison, Oh included the production unit in the whole reference set and constructed the global-Malmquist-Luenberger (GML) index (Oh, 2010; Yang et al., 2021).
Appealing to previous research and China Corporate Social Responsibility Investigation System and Standards, the social dimension of CSR covers five contents of social economy, tax, employees' rights and interests, legal responsibility and good faith management. Besides, according to data availability and unexpected output, the details of SOC index take total assets and labor as input indicators, taxes and salaries as expected outputs, and the frequency of litigation and arbitration, as well as violations of discipline and regulations as unexpected outputs, which are shown in Table 3.
Primary Indicator | Secondary Indicator | Tertiary Indicator |
Input index | Capital | Total assets |
Labor | Number of employees | |
Output index | Desirable output | Tax fee |
Staff salary | ||
Undesirable output | Frequency of litigation and arbitration | |
Frequency of discipline & regulations violations |
According to existing studies and China Corporate Social Responsibility Survey and Evaluation System and Standards, the economic dimension of CSR includes shareholders' equity, transaction risk and operational risk. In Table 4, total assets and labor are taken as input indicators, earnings per share and revenue from main businesses are expected outputs, and frequency of transaction abnormalities and frequency of equity pledge are unexpected outputs by analyzing economic dimension.
Primary Indicator | Secondary Indicator | Tertiary Indicator |
Input index | Capital | Total assets |
Labor | Number of employees | |
Output index | Desirable output | Earnings per share |
Main business revenue | ||
Undesirable output | Trading frequency | |
Frequency of shareholding pledge |
Based on determining the input-output index, we adopted the Bootstrap-SBM-GML model with the constant return to scale (CRS) and Z-score normalization processing (normal normalization). Then, the Bootstrap method is used to simulate the efficiency value for 2000 repeated samples with original samples (Simar and Wilson, 1998). Finally, the total factor productivity of social and economic dimensions of CSR is calculated.
In view of inconsistent measurement standards of three-dimension indicators of CSR, the method of direct adding or dispersion normalization causes that the CSR value obtained by summation cannot well reflect the idea of weight balance of each dimension. Therefore, the data of three dimensions are converted into the unified Z-score value for comparison by adopting Z-score standardization. Z-score is got from standardization, then different dimensions are comparable, and these three dimensions can be directly summed up to obtain the CSR Index.
In this part, we use the benchmark regression model (1)-(4) to analyze the impact of mandatory disclosure policy. According to the setting model, parameter estimation results are shown in Table 5.
Items | CSR | CER | SOC | ECO |
PL | 0.881*** (5.72) | 1.230*** (19.43) | 0.128** (2.50) | −0.193** (−2.20) |
lnta | 0.416*** (4.00) | 0.181*** (4.23) | 0.089 (1.41) | 0.146** (2.46) |
cd | 0.143*** (3.60) | −0.006 (−0.39) | 0.084*** (3.48) | 0.065*** (2.88) |
fr | −0.939** (−2.42) | −0.114 (−0.71) | −0.086 (−0.37) | −0.738*** (−3.34) |
owner | 0.457* (1.66) | 0.114 (1.01) | 0.177 (1.06) | 0.166 (1.06) |
age | 0.081** (2.43) | −0.019 (−1.36) | 0.085*** (4.20) | 0.015 (0.77) |
dar | −1.641*** (−4.21) | −0.051 (−0.32) | −0.903*** (−3.82) | −0.687*** (−3.09) |
tq | 0.229*** (5.19) | 0.095*** (5.27) | 0.030 (1.11) | 0.104*** (4.13) |
Cons | −4.970 | −2.248 | −1.576 | −0.805*** (−5.53) |
N | 2056 | 2056 | 2056 | 2056 |
F | 104.66 | 342.78 | 15.87 | 7.89 |
R−squared | 0.3186 | 0.6049 | 0.0662 | 0.0340 |
Notes: *, **, *** denote significant levels of 10%, 5% and 1%, respectively. The values in brackets are T-statistics. |
According to Table 5, we get the results that mandatory disclosure policy had a significant impact on CSR and its three dimensions. Still, their effect on degree and direction are significantly different. To be specific, the impact on the environmental dimension is positive, while the social dimension is positive, and its influence degree is low. The impact on the economic dimension is significantly negative, and the degree is also small. The above conclusions are consistent with Hypothesis H1. However, due to the endogeneity problem of neglected variables in the benchmark regression, the estimation effect is imperfect, and their results may lack robustness. At the same time, due to the influence of breakpoints, we find it difficult to capture the relevant mutation information, as well as the effect of policy implementation. Based on this, the impact of mandatory disclosure on various dimensions of CSR by adopting the RDD method is further analyzed in order to effectively avoid endogenous problems caused by neglected variables that are related to time trends in benchmark regression.
RDD is different from the least square estimation in the baseline regression. On the one hand, the regression discontinuity estimation does not have harsh requirements on the endogeneity of the variable itself but supposes that the place of variables cannot be controlled accurately by individuals. On the other hand, its research design is more similar to a randomized controlled experiment with more reliable estimated results (Lee and Lemieux, 2010). We should first observe whether mandatory disclosure policy causes breakpoint changes in core variables or not when doing RDD estimation. There are two problems in the scatter diagram method, although it is usually used for observation in previous years, one is too many samples but not intuitive; the other may be unclear enough jump phenomenon in its actual analysis. Therefore, we use the fitting method to fit the left and right of the breakpoint respectively and speculate whether the jump phenomenon occurs by observing the difference of fitting lines on both sides (Calonico et al., 2015). According to the set model, here are fitting results from Figure 2.
According to Figure 2, it can be seen that the linear fitting of each dependent variable and second-order polynomial fitting have the same jumping. First of all, mandatory disclosure policy has a significant impact on CSR. CSR index jumps significantly at the breakpoint. In linear fitting, its intercept rises from about −0.25 to 0.75; that is, the local treatment effect of mandatory disclosure policy is about 1, which indicates that the policy can improve the overall CSR for enterprises to some extent. Second, in the linear fitting of the CER index, we can see that the intercept increases from about −0.4 to 0.75. The local treatment effect of mandatory disclosure policy is about 1.15, which denotes that the policy has a significant positive effect on the environmental dimension of CSR. Third, in the SOC index, the jump in linear fitting and second-order polynomial fitting is not obvious, which is different from the baseline regression results, which means that the policy has no significant impact on the social dimension of CSR. Fourth, in the linear fitting of the ECO index, its intercept decreases from about 0.15 to −0.03, and the local treatment effect of mandatory disclosure policy is about -0.18, which shows that the policy has a low negative impact on the economic dimension of CSR.
In view of breakpoints of core variables, the impact of mandatory disclosure policy is analyzed by using the regression discontinuity model. The selection of bandwidth has a significant impact on the regression estimation results of breakpoints. Based on the characteristics of annual breakpoints and annual data, the method of setting the regression bandwidth by many experiments is adopted. In order to ensure the robustness of the estimation results, three kinds of broadband, such as ±4, ±3 and ±2, are selected in the model, and ±4 is taken as the benchmark bandwidth among them. Besides, the selection of kernel function also affects the result of regression discontinuity estimation. To ensure the robustness, three estimation methods of Triangle, Epanechnikov and Uniform are used respectively in model setting, in which Triangle is taken as the reference kernel function. Here are the estimated results in Table 6.
Order | Covariate | CSR | CER | SOC | ECO |
First−order polynomial | Without covariates | 0.482** (2.37) |
1.148*** (10.85) |
−0.043 (−0.39) |
−0.434*** (−3.41) |
With covariates | 0.584*** (3.141) |
1.134*** (12.38) |
−0.017 (−0.26) |
−0.384*** (−3.32) |
|
Second−order polynomial | Without covariates | 0.254 (1.04) |
0.405** (4.67) |
−0.115 (−0.47) |
−0.729** (−2.16) |
With covariates | 0.312 (1.23) |
0.330*** (6.82) |
0.103 (0.46) |
−0.538* (−1.76) |
|
Notes: *, **, *** represent significant levels of 10%, 5% and 1%, respectively. The values in brackets are Z-statistics. |
According to Table 6, the impact of mandatory disclosure policy on CSR and its three dimensions is basically the same as the results of the fitting method in the first-order polynomial regression. To be specific, it has a significant positive effect on the environmental dimension and has no significant effect on the social dimension, as well as a significant negative impact on the economic dimension. The differences between the three dimensions in the second-order regression are consistent with the first-order, but there is no significant jump in CSR. The addition of covariates did not change the regression results significantly.
According to empirical research, we tested that mandatory disclosure policy has a significant impact on CSR, especially has a heterogeneous impact on its three dimensions. First of all, the policy has a significant promoting effect on the environmental dimension and a negative impact on the economic dimension of CSR. In order to improve the part of environmental quality, enterprises have to disclose social responsibility reports and shoulder more environmental responsibility (Albertini, 2014; Federica et al., 2020), invest in research, development and innovation, and reduce corporate emissions, etc. There is a negative impact on the economic benefits from compulsory disclosure of social responsibility reports. For one part, it relates to the crowding-out effect of technological innovation on the economic dimension of CSR in the short term; for the other part, it connects with the reduction of investment or cover-up the effect by investors due to the content of CSR reports. The estimation results of the environment and economic dimensions of CSR are basically identical with the difference of OLS, but their impact degree is different. Through the analysis of coefficient before the policy change, we find that estimation results of the OLS are too optimistic, such as the promotion of CSR environmental dimension effect is expanded by mandatory disclosure policies; the negative impact of mandatory disclosure policy on economic dimension to a certain extent is underestimated. In addition, compared with the OLS estimate result, regression discontinuity result shows that level of the social dimension of CSR has no obvious jump near the policy implement breakpoints, which indicates that mandatory disclosure of social responsibility reports cannot prompt enterprises to assume more social dimension responsibility although more social responsibilities, like donation, organize activities are filled in enterprises CSR report. This also proves the limitations of OLS methods used in policy effects. From the above analysis, we verified hypotheses 1 and 2.
The model setting affects the accuracy of regression discontinuity results, while bandwidth is the key. In the previous RDD estimation, the IK method was usually used to calculate the optimal bandwidth (Imbens and Kalyanaraman, 2012), and then 50% and 200% of the optimal bandwidth was used for the robustness test. Based on year breakpoints and the characteristics of annual data, we adopt setting experiments many times by taking ±4 (plus or minus four years) as benchmark return bandwidth, as well as selecting ±2 and ±3 as inspection scope. RDD estimation results of these two groups of bandwidth tests are shown in Table 7.
Bandwidth | Covariate | CSR | CER | SOC | ECO |
±3 | Without covariates | 0.421** (2.01) |
0.900*** (6.04) |
−0.021 (−0.23) |
−0.532*** (−3.03) |
With covariates | 0.434** (2.23) |
0.855*** (6.63) |
−0.075 (−0.79) |
−0.444*** (−2.81) |
|
±2 | Without covariates | 0.497** (2.32) |
0.900*** (6.04) |
0.003 (0.04) |
−0.532*** (−3.03) |
With covariates | 0.477** (2.34) |
0.832*** (6.44) |
−0.045 (−0.48) |
−0.422*** (−2.67) |
|
Notes: *, **, *** stand for significant levels of 10%, 5% and 1%, respectively. The values in brackets are Z-statistics. |
Table 7 shows that the size and significance of coefficients in each group of CSR and three dimensions are basically consistent with the above conclusions. The heterogeneity of policy impact on CSR and its three dimensions are the same as the basic RDD estimation results, and the conclusion is robust. Besides, the conclusion of this test are also stated that the impact of mandatory CSR disclosure policy is different in the short, medium and long term.
According to the above conclusion, we take 2007 and 2009 as breakpoints for regression respectively in order to eliminate the difference of CSR performance over time. Here are the RDD estimation results at different breakpoints in Table 8.
Breakpoint | Covariate | CSR | CER | SOC | ECO |
2007 | Without covariates | 0.539*** (4.27) |
0.344*** (5.65) |
−0.054 (1.07) |
0.078 (1.15) |
With covariates | 0.421*** (3.76) |
0.370*** (7.18) |
−0.015 (−0.34) |
0.066 (0.83) |
|
2009 | Without covariates | −0.252 (−0.83) |
0.491*** (3.30) |
−0.023 (−0.51) |
−0.028 (−0.30) |
With covariates | −0.043 (0.14) |
0.421*** (3.09) |
−0.044 (−0.39) |
−0.021 (−0.23) |
|
Notes: *, **, *** denote significant levels of 10%, 5% and 1%, respectively. The values in brackets are Z-statistics. |
Table 8 shows that the social and economic dimension index of CSR have no jumps in 2007 and 2009. However, the environmental dimension index shows a significant upward jump in 2007 and 2009, indicating that mandatory disclosure had a "warming up effect" on the environmental dimension of CSR in the year before and after the promulgation. Although it cannot pass the placebo test completely, based on the relation between environmental effect and R & D investment and pollutant emission to some extent, the breakpoint of environmental dimension can be explained by economics based on the placebo test of economic and social dimensions, which is deemed to pass.
The estimation method of the breakpoint affects its regression result. Unlike the triangle kernel method in previous research, we adopt Epanechnikov and Uniform to get the regression result. Here are the RDD estimation results with these two algorithms in Table 9.
Algorithm | Covariate | CSR | CER | SOC | ECO |
Epanechnikov | Without covariates | 0.515** (2.55) |
1.174*** (11.34) |
−0.034 (−0.56) |
−0.424*** (−3.38) |
With covariates | 0.608*** (3.27) |
1.163*** (12.93) |
−0.026 (−0.49) |
−0.378*** (−3.31) |
|
Uniform | Without covariates | 0.893*** (5.42) |
1.232*** (15.35) |
−0.022 (−0.44) |
−0.184* (−1.78) |
With covariates | 0.896*** (5.81) |
1.226*** (17.23) |
−0.021 (−0.43) |
−0.188** (−1.95) |
|
Notes: *, **, *** mean significant levels of 10%, 5% and 1%, respectively. The values in brackets are Z-statistics. |
According to Table 9, the results of CSR and various dimensions are consistent with the above. Therefore, the heterogeneity of impact on CSR and its three dimensions is still the same as the results of RDD basic estimation, and the conclusion is still robust.
In this section, the robustness analysis is performed by adjusting the explained variables. In 2001 and 2002, Tone proposed model of SBM and the super-efficiency SBM. In the latter model, he considered the slack variables and sorted the decision-making units whose efficiency value was greater than 1 (Tone, 2001, 2002). In this paper, CCR-GML, super-efficiency SBM-GML with constant returns to scale and variable return to scale, ordinary SBM-GML with the constant return to scale and variable return to scale are respectively used to calculate the social and economic dimensions and the corresponding CSR. Here are the RDD estimates results for different explanatory variable models in Table 10.
DEA model | Covariate | CSR | CER | SOC | ECO |
CCR−GML | Without covariates | 0.658*** (3.01) |
1.148*** (10.85) |
−0.037 (−0.37) |
−0.325** (−2.09) |
With covariates | 0.684*** (3.69) |
1.134*** (12.38) |
−0.024 (−0.28) |
−0.313** (−2.38) |
|
(CRS) Super−SBM−GML |
Without covariates | 0.619*** (3.16) |
1.148*** (10.85) |
−0.014 (−0.18) |
−0.386*** (−2.83) |
With covariates | 0.634*** (3.56) |
1.134*** (12.38) |
−0.005 (−0.07) |
−0.015*** (−3.06) |
|
(VRS) Super−SBM−GML |
Without covariates | 0.665*** (3.29) |
1.148*** (10.85) |
−0.015 (−0.16) |
−0.339*** (−2.82) |
With covariates | 0.718*** (4.15) |
1.134*** (12.38) |
0.015 (0.20) |
−0.318*** (−2.89) |
|
(CRS) SBM−GML |
Without covariates | 0.613*** (3.10) |
1.148*** (10.85) |
−0.014 (−0.17) |
−0.392*** (−2.84) |
With covariates | 0.627*** (3.49) |
1.134*** (12.38) |
−0.005 (−0.08) |
−0.389*** (−3.08) |
|
(VRS) SBM−GML |
Without covariates | 0.669*** (3.36) |
1.148*** (10.85) |
−0.007 (−0.08) |
−0.342*** (−2.83) |
With covariates | 0.715*** (4.14) |
1.134*** (12.38) |
0.018 (0.24) |
−0.324*** (−2.91) |
|
Notes: *, **, *** stand for significant levels of 10%, 5% and 1%, respectively. The values in brackets are Z-statistics. |
From Table 10, we can see that the impact of mandatory disclosure policy on CSR and its three dimensions is still the same as the basic RDD estimation results. Specifically, the environmental dimension has a significant positive effect; the social dimension has no obvious effect; the economic dimension has a significant negative effect; the conclusions are still robust.
The validity of RDD estimation depends on two assumptions. First, driving variables are not manipulated. In this paper, the processing variable is the year variable, and the driving variable is the mandatory disclosure policy, as well as the year exists objectively and policy is not subject to subjective influence. Second, the effectiveness of RDD estimation is also decided by the smoothness hypothesis, that is, there should be no obvious jump between the two sides of the cut-off point for the covariates that influence the policy effect. This part also tests the continuity of the covariables of the breakpoint regression model at the breakpoint. Here are the fitting results in Figure 3.
It may be unscientific to observe the jumps to some extent from b, c and d of Figures 3. The actual jumps situation still needs to take the specific regression results for reference. Here are in Table 11.
lnta | cd | fr | owner | age | dar | tq | |
Coef. | −0.098 (−0.68) |
−1.8 e+05 (−1.02) |
0.006 (0.27) |
0.016 (0.35) |
0.000 (0.00) |
−0.003 (−0.15) |
−0.277 (−1.23) |
Notes: the values in brackets are Z-statistics. |
According to Table 11, we get to know that each covariate has no significant jump at the breakpoint in regression model, that is, the significance level of the covariate jumping at the breakpoint has not reached 10%. It meets the premise of the smoothness hypothesis for the regression discontinuity.
According to hypothesis 3 above, we set PLENVit as a mediating variable to analyze the mechanism of mandatory disclosure policy affecting the dimension of corporate environmental responsibility. PLENVit is a dummy variable, that is, when the sample enterprise i disclosed the contents of environmental protection and sustainable development in year t, PLENVit = 1; If the sample enterprise i did not disclose the contents of environmental protection and sustainable development in year t, then PLENVit = 0. The mediating effect test model is as follows:
CERit=δ0+δ1∗PLt+δ∗Xit+πi+εit | (9) |
PLENVit=α0+α1∗PLt+α∗Xit+πi+ϵit | (10) |
CERit=β0+β1∗PLt+β2∗PLENVit+β∗Xit+πi+θit | (11) |
PLt={1,Yt≥00,Yt<0 |
In formula (9)-(11), δ1 measures the influence of mandatory disclosure policy on the environmental dimension index CERit,α1 tests the impact of mandatory disclosure policy on business PLENVit,β1 measures the direct influence of mandatory disclosure policy on the environmental dimension index CERit,β2 measures the influence of PLENVit environmental dimension index CERit. With the method of the stepwise testing regression coefficient, the regression results in Table 12 show that the meaning and calculation method of variable in the regression equation are consistent with regression discontinuity.
Items | CER (1) | PLENV (2) | CER (3) |
PL | 1.198*** | 0.627*** | 0879*** |
PLENV | — | — | 0.716*** |
covariates | Yes | Yes | Yes |
Cons | −1.606 | −0.411 | −1.321 |
N | 2056 | 2056 | 2056 |
R-squared | 0.5774 | 0.6266 | 0.5780 |
Notes: *** stands for significant levels of 1%. |
Model (1) verifies the direct impact of mandatory disclosure policy on environmental dimension index CERit from Table 12. The result shows that the impact of mandatory disclosure policy on CERit is significantly positive, which is consistent with the regression discontinuity results. Model (2) tested the effect of mandatory disclosure policy on PLENVit, and their regression coefficient is 0.627 and significantly positive, which indicates that mandatory disclosure policy leads to the increase of PLENVit. Model (3) shows effect coefficient of mandatory disclosure policy on CERit is 0.879 and significant. The environmental dimension index from PLENVit is also significantly positive at the 1% confidence level, which means that disclosing environment and sustainable development for listed companies plays a partial mediating role in the impact of mandatory disclosure policy on the environmental dimension index. In conclusion, the impact of mandatory disclosure policy on the environmental dimension index is partly realized by promoting enterprises to disclose the environment and sustainable development.
Next, the Sobel test is used to verify the robustness of mediating effect, and the analysis results are consistent with the stepwise test. The total effect of mandatory disclosure policy on the environmental dimension is equal to the direct effect of 0.879 plus the indirect effect of 0.319, and the total effect is 1.198. The calculated mediating effect accounts for 26.63% of the total effect.
Consistent with the mediating effect test of environmental dimension, the following mediating effect model is also built to verify the impact of return on assets (ROA) on the economic dimension:
ECOit=δ0+δ1∗PLt+δ∗Xit+πi+εit | (12) |
ROAit=α0+α1∗PLt+α∗Xit+πi+ϵit | (13) |
ECOit=β0+β1∗PLt+β2∗ROAit+β∗Xit+πi+θit | (14) |
PLt={1,Yt≥00,Yt<0 |
In formula (12)–(14), δ1 measures the influence of mandatory disclosure policy on the economic dimension index ECOit. α1 tests the impact of mandatory disclosure policy on business ROAit. β1 represents the direct influence of mandatory disclosure policy on the economic dimension index ECOit. β2 measures the influence of ROAit on CSR economic dimension index ECOit. By adopting the method of stepwise testing regression coefficient, the meaning and calculation method of variables in the regression equation are consistent with that in regression discontinuity. Here are the regression results in Table 13.
Items | ECO (1) | ROA (2) | ECO (3) |
PL | −0.195*** | −0.027*** | −0.034 |
ROA | — | — | 5.980*** |
covariates | Yes | Yes | Yes |
Cons | −1.323 | 0.028 | −1.488 |
N | 2056 | 2056 | 2056 |
R−squared | 0.1285 | 0.4460 | 0.1877 |
Notes: *** stands for significant levels of 1%. |
Model (1) verifies the direct impact of mandatory disclosure policy on the economic dimension indicator ECOit in Table 13. The results show that the impact of mandatory disclosure policy on ECOit is significantly negative, which is consistent with the regression discontinuity results. Model (2) verifies the effect of mandatory disclosure policy on return on assets ROAit of enterprises. The regression coefficient is −0.027 and significantly negative, meaning that mandatory disclosure policy decreases return on assets ROAit. The effect coefficient of mandatory disclosure policy on Ecoit is −0.034 in Model (3), but not significant. The effect of ROAit on Ecoit is significantly positive at the 1% confidence level, which indicates that ROA plays a fully mediating role in the effect of mandatory disclosure policy on the economic dimension index. In conclusion, the effect of mandatory disclosure policy on the economic dimension index is almost completely realized by reducing the return on enterprise assets ROAit.
Next, the Sobel test is used to verify the robustness of the mediating effect; it shows that the analysis results are consistent with the stepwise test. The total effect of mandatory disclosure policy on the economic dimension is equal to the direct effect of −0.034 plus the indirect effect of −0.161, and the total effect is −0.195. The calculated mediating effect accounts for 82.56% of the total effect.
According to Guidelines for Environmental Information Disclosure of Listed Enterprises (Revised Opinion Manuscript) from the Ministry of Environmental Protection of China in sep14th, it announces that thermal power, steel, cement, electrolytic aluminium, coal, metallurgical, chemical, petrochemical, building materials, paper making, brewing, pharmaceutical, fermentation, textile, leather and mining and other 16 sectors belong to the heavy pollution industry. Based on China National Economy Industry Classification (GB T4754−2011), we divide samples into 110 heavy pollution industry and 147 non-heavy pollution industry enterprises and then analyze the impact of mandatory disclosure policy on CSR and their different dimensions for these two types of enterprises (Chang et al., 2019; Sedláček and Popelková, 2020;Xu et al., 2020). The RDD analysis results of industry heterogeneity are shown in Table 14.
Industry | Covariate | CSR | CER | SOC | ECO |
Heavy polluting industry | Without covariates | 0.287 (1.05) |
1.079*** (7.15) |
−0.056 (−0.60) |
−0.600*** (−1.80) |
With covariates | 0.334 (1.36) |
0.985*** (7.26) |
−0.054 (−0.66) |
−0.544*** (−1.74) |
|
Non-heavy polluting industries | Without covariates | 0.629** (2.18) |
1.200*** (8.82) |
−0.061 (−0.70) |
−0.310* (−1.80) |
With covariates | 0.772*** (2.94) |
1.247*** (10.45) |
−0.064 (−0.86) |
−0.264* (−1.74) |
|
Notes: **, *** denote significant levels of 10%, 5% and 1%, respectively. The values in brackets are Z-statistics. |
From Table 14, the effect of mandatory disclosure policy on enterprises' three dimensions varies with different industries. In the environmental dimension, the non-heavy polluting industries are more positively affected by the policy. In the social dimension, the policy effect of heavily polluting industries is still negative, while it is not significantly positive for the non-heavy polluting industries. There is no obvious difference between these two in the economic dimension. On the whole, mandatory disclosure policy has a better effect on CSR for the non-heavy polluting industries.
In the 1990s, a Japanese economist named Masu Uekusa put forward the "Theory of Duality" that state-owned enterprises have the nature of public and enterprise in his book "The Economics of Public Regulation". In China's stock market, state-owned enterprises occupy a considerable proportion, and they should have achieved more social and national policy goals. Based on the property right character of enterprises samples in the year of policy promulgation (2008) in the CSMAR database, we classify 257 samples into 202 state-owned enterprises and 55 non-state-owned enterprises and analyze the impact of mandatory disclosure policy on different dimensions of these two kinds of enterprises. The RDD analysis results of industry heterogeneity are reported in Table 15.
Owner | Covariate | CSR | CER | SOC | ECO |
State-owned enterprises | Without covariates | 0.300 (1.23) |
1.054*** (8.72) |
−0.034 (−0.46) |
−0.341** (−2.52) |
With covariates | 0.372* (1.68) |
1.031*** (10.06) |
−0.020 (−0.31) |
−0.336*** (−2.69) |
|
Non-state-owned enterprises | Without covariates | 1.151*** (3.71) |
1.493*** (6.98) |
0.019 (0.19) |
−0.172 (−1.29) |
With covariates | 1.297*** (5.61) |
1.516*** (9.28) |
0.021 (0.17) |
−0.175 (−1.59) |
|
Notes: **, *** mean significant levels of 5% and 1%, respectively. The values in brackets are Z-statistics. |
The effect of mandatory disclosure policy on CSR and its three dimensions varies with the nature of property rights in Table 15. In the environmental dimension, non-state-owned enterprises are more positively affected by the policy; in the social dimension, the policy effect of state-owned enterprises is still negative, while the policy effect of non-state-owned enterprises is not significantly positive. In the economic dimension, the negative impact of non-state-owned enterprises is less and insignificant. On the whole, mandatory disclosure policy has a better effect on the CSR for non-state-owned enterprises.
Mandatory disclosure policies have a complex impact on corporate social responsibility. This paper uses principal component analysis and the Bootstrap-SBM-GML model to obtain the index of three dimensions of CSR. Based on the RDD method, the research draws the following conclusions.
First, enterprises engage more in social responsibility after the implementation of CSR mandatory disclosure. However, there is heterogeneity in enterprises decisions on engaging in the three dimensions of CSR and the effects of the mandatory CSR disclosure policy are significantly different on the CSR dimensions. In the environmental dimension, mandatory disclosure policy has a statistically significant positive effect, and a large jump in the environmental dimension index is observed near the breakpoint, which indicates that the policy has played a significant effect in promoting corporate environmental responsibility. In the social dimension, the impact of mandatory disclosure policy is not significant, which indicates that the policy does not make a significant change on the level of social responsibility of listed companies in China. In the economic dimension, mandatory disclosure policy has a statistically significant negative impact, and an obvious downward jump in the index of the economic dimension is observed near the breakpoint, which indicates that the mandatory CSR policy negatively affects the economic benefits of enterprises to some extent.
Second, the implementation of mandatory disclosure policy is consistent with the turning point of CSR and all dimensions, but the impact trend is heterogeneous. In the test of different bandwidth, CSR and both environmental and economic dimensions show jumps in 2008 with policies promulgation while the impact of mandatory CSR disclosure policy is different in the short, medium and long term. In the environment dimension, mandatory disclosure policy significantly promoted the environmental level in the short term while the effect is increased in the medium and long term with a stronger promoting effect. In the social dimension, it slightly promoted the level of the social dimension in the short term but slightly inhibited in the medium and long term. In the economic dimension, the negative impact is enhanced from the short to medium term while the negative impact is reduced in the long term.
Finally, there is a heterogeneous impact mechanism of CSR on the various dimensions after implementing mandatory disclosure policy. We find that environment and sustainable development information plays a partial mediating role in the environmental dimension while return on assets plays a fully mediating role in the economic dimension. We also find that compared with heavy polluters, the mandatory disclosure policy has a better effect on non-heavy pollution enterprises. The mandatory disclosure policy has better effects on non-state-owned enterprises compared with state-owned enterprises.
The above conclusions offer references for the government to make relevant decisions. First of all, information disclosure policies should be set according to the characteristics of different dimensions of enterprises. In this paper, CSR is divided into environmental, social and economic dimensions. According to the regression discontinuity study, the implementation effect of mandatory CSR disclosure policy is obviously different in the three dimensions. Therefore, it is necessary to implement CSR disclosure policies more specifically to different dimensions of social responsibility. In the heterogeneity analysis, although the positive and negative effects of mandatory disclosure policy on the three dimensions do not change due to the nature of industries and property rights, the policy obviously has a better effect on non-heavy polluting and non-state-owned enterprises. This also provides ideas for future empirical research, that is, to study listed companies in different industries or at different stages when data are available and explore effective targeted regulation or information disclosure policies. Then, mandatory disclosure policy has brought significant improvement to the overall level of CSR, which is proved to be effective. Still, from the medium and long-term perspective, the policy effect of mandatory disclosure is gradually weakened, such as the cliff descending of CER in 2009. Finally, there are no requirements in China for assuring or auditing CSR reports, therefore, this may cause some problems. For instance: enterprises have the opportunity to exaggerate the fact of their reports and thus enterprises with more flexible choices may weaken the effect of CSR policy. Policymakers in China need to take the above aspects into consideration as well as other countermeasures such as implementing long-term regulation policy on information disclosure of listed enterprises from aspects of finance and legislation; improving the pertinence of policies, maintaining the continuity of policies, ensuring the continuity of policy effects, guiding enterprises to voluntarily disclosure gradually, improving enterprises' ability to assume social responsibilities.
All authors declare no conflicts of interest in this paper.
This research was funded by the National Social Science Fund of China, grant number 20BTJ022.
[1] |
Charnes A, Cooper WW, Rhodes E (1978) Measuring the efficiency of decision making units. Eur J Oper Res 2: 429-444. doi: 10.1016/0377-2217(78)90138-8
![]() |
[2] | Albertini E (2014) A Descriptive Analysis of Environmental Disclosure: A Longitudinal Study of French Companies. J Bus Ethics 121. |
[3] | Angrist JD, Pischke JS (2008) Mostly Harmless Econometrics: An Empiricist's Companion, Princeton University Press. |
[4] | Arena C, Liong R, Vourvachis P (2018) Carrot or stick: CSR disclosures by Southeast Asian companies. Sustainability Account Manage Policy J 9. |
[5] |
Atkinson L, Galaskiewicz J (1988) Stock ownership and company contributions to charity. Admin Sci Q, 82-100. doi: 10.2307/2392856
![]() |
[6] |
Christainsen GB, Haveman RH (1981) The contribution of environmental regulations to the slowdown in productivity growth. J Environ Econ Manage 8: 381-390. doi: 10.1016/0095-0696(81)90048-6
![]() |
[7] | Calonico S, Cattaneo MD, Titiunik R (2015) Optimal Data-Driven Regression Discontinuity Plots. J Am Stat Assoc 110. |
[8] | Carroll AB (1979) A Three-Dimensional Conceptual Model of Corporate Performance. Acad Manage Rev 4. |
[9] |
Chang CY, Shie FS, Yang SL (2019) The relationship between herding behavior and firm size before and after the elimination of short-sale price restrictions. Quant Financ Econ 3: 526-549. doi: 10.3934/QFE.2019.3.526
![]() |
[10] | Chen YC, Hung M, Wang Y (2018) The effect of mandatory CSR disclosure on firm profitability and social externalities: Evidence from China. J Account Econ 65. |
[11] |
Clarkson MBE (1995) A Stakeholder Framework for Analyzing and Evaluating Corporate Social Performance. Acad Manage Rev 20: 92-117. doi: 10.2307/258888
![]() |
[12] |
Demirtas YE, Kececi NF (2020) The efficiency of private pension companies using dynamic data envelopment analysis. Quant Financ Econ 4: 204-219. doi: 10.3934/QFE.2020009
![]() |
[13] |
Dhaliwal D, Li OZ, Tsang A, et al. (2014) Corporate social responsibility disclosure and the cost of equity capital: The roles of stakeholder orientation and financial. transparency. J Account Public Policy 33: 328-355. doi: 10.1016/j.jaccpubpol.2014.04.006
![]() |
[14] |
Dhaliwal DS, Li OZ, Tsang A, et al. (2011) Voluntary Nonfinancial Disclosure and the Cost of Equity Capital: The Initiation of Corporate Social Responsibility Reporting. Account Rev 86: 59-100. doi: 10.2308/accr.00000005
![]() |
[15] | Fama EF, Laffer AB (1972) The Number of Firms and Competition. Am Econ Rev 62. |
[16] | Federica B, Arianna L, Riccardo T (2020) Credibility of Environmental Issues in Non-Financial Mandatory Disclosure: Measurement and Determinants. J Clean Prod 288. |
[17] | Feng Y, Chen S, Failler P (2020) Productivity Effect Evaluation on Market-Type Environmental Regulation: A Case Study of SO2 Emission Trading Pilot in China. Int J Environ Res Public Health 17. |
[18] | Fogler HR, Nutt F (1975) A Note on Social Responsibility and Stock Valuation. Acad Manage J 18. |
[19] | Galaskiewicz J (1997) An urban grants economy revisited: Corporate charitable contributions in the Twin Cities, 1979-81, 1987-89. Admin Sci Q, 445-471. |
[20] |
Gallego-Álvarez I, Lozano MB, Rodríguez-Rosa M (2018) An analysis of the environmental information in international companies according to the new GRI standards. J Clean Prod 182: 57-66. doi: 10.1016/j.jclepro.2018.01.240
![]() |
[21] | Gelman A, Imbens G (2019) Why High-Order Polynomials Should Not Be Used in Regression Discontinuity Designs. J Bus Econ Stat 37. |
[22] |
El Ghoul S, Guedhami O, Kwok CC, et al. (2011) Does corporate social responsibility affect the cost of capital? J Bank Financ 35: 2388-2406. doi: 10.1016/j.jbankfin.2011.02.007
![]() |
[23] | Hardy DR, Schwartz MF (1996) Customized information extraction as a basis for resource discovery. ACM Trans Comput Syst (TOCS) 14. |
[24] | Hemingway CA, Maclagan PW (2004) Managers' Personal Values as Drivers of Corporate Social Responsibility. J Bus Ethics 50. |
[25] |
Hong M, Drakeford B, Zhang K (2020) The impact of mandatory CSR disclosure on green innovation: evidence from China. Green Financ 2: 302-322. doi: 10.3934/GF.2020017
![]() |
[26] | Hung M, Shi J, Wang Y (2013) Mandatory CSR disclosure and information asymmetry: Evidence from a quasi-natural experiment in china. Social Sci Electron Publishing 33: 1-17. |
[27] | Imbens G, Kalyanaraman K (2012) Optimal Bandwidth Choice for the Regression Discontinuity Estimator. Rev Econ Stud 79. |
[28] | Kim Y, Li H, Li S (2014) Corporate social responsibility and stock price crash risk. J Bank Financ 43. |
[29] |
Kim Y, Park MS, Wier B (2012) Is Earnings Quality Associated with Corporate Social Responsibility? Account Rev 87: 761-796. doi: 10.2308/accr-10209
![]() |
[30] | Lee DS, Lemieux T (2010) Regression Discontinuity Designs in Economics. J Econ Lit 48. |
[31] | Li F, Yang C, Li Z, et al. (2021) Does Geopolitics Have an Impact on Energy Trade? Empirical Research on Emerging Countries. Sustainability 13. |
[32] |
Li Z, Liao G, Albitar K (2020) Does corporate environmental responsibility engagement affect firm value? The mediating role of corporate innovation. Bus Strat Environ 29: 1045-1055. doi: 10.1002/bse.2416
![]() |
[33] | Lin KJ, Tan J, Zhao L, et al. (2015) In the name of charity: Political connections and strategic corporate social responsibility in a transition economy. J Corp Financ 32. |
[34] | Mobus JL (2005) Mandatory environmental disclosures in a legitimacy theory context. Account Audit Account J 18. |
[35] | Oh DH (2010) A global Malmquist-Luenberger productivity index. J Prod Anal 34. |
[36] | Petrovits CM (2005) Corporate-sponsored foundations and earnings management. J Account Econ 41. |
[37] |
Prior D, Surroca J, Tribo JA (2008) Are socially responsible managers really ethical? Exploring the relationship between earnings management and corporate social responsibility. Corp Govern Inter Rev 16: 160-177. doi: 10.1111/j.1467-8683.2008.00678.x
![]() |
[38] | Cowen SS, Ferreri LB, Parker LD (1987) The impact of corporate characteristics on social responsibility disclosure: A typology and frequency-based analysis. Pergamon 12. |
[39] | Sánchez CM (2000) Motives for Corporate Philanthropy in El Salvador: Altruism and Political Legitimacy. J Bus Ethics 27. |
[40] |
Sedláček J, Popelková V (2020) Non-financial information and their reporting—evidence of small and medium-sized enterprises and large corporations on the Czech capital market. Natl Account Rev 2: 204-216. doi: 10.3934/NAR.2020012
![]() |
[41] | Simar L, Wilson PW (1998) Sensitivity Analysis of Efficiency Scores: How to Bootstrap in Nonparametric Frontier Models. Manage Sci 44. |
[42] | Tone K (2001) A slacks-based measure of efficiency in data envelopment analysis. Eur J Oper Res 130. |
[43] | Tone K (2002) A slacks-based measure of super-efficiency in data envelopment analysis. Eur J Oper Res 143. |
[44] |
Tuzcuoglu T (2020) The impact of financial fragility on firm performance: an analysis of BIST companies. Quant Financ Econ 4: 310-342. doi: 10.3934/QFE.2020015
![]() |
[45] |
Utomo MN, Rahayu S, Kaujan K, et al. (2020) Environmental performance, environmental disclosure, and firm value: empirical study of non-financial companies at Indonesia Stock Exchange. Green Financ 2: 100-113. doi: 10.3934/GF.2020006
![]() |
[46] | Verrecchia RE (2001) Essays on disclosure. J Account Econ 32. |
[47] | Waller DS, Lanis R (2009) Corporate Social Responsibility (CSR) Disclosure of Advertising Agencies: An Exploratory Analysis of Six Holding Companies' Annual Reports. J Adver 38. |
[48] |
Xu M, Albitar K, Li Z (2020) Does corporate financialization affect EVA? Early evidence from China. Green Financ 2: 392-408. doi: 10.3934/GF.2020021
![]() |
[49] | Yang C, Li T, Albitar K (2021) Does energy efficiency affect ambient PM2.5? The moderating role of energy investment. |
1. | Husam Ananzeh, Hamzeh Al Amosh, Khaldoon Albitar, The effect of corporate governance quality and its mechanisms on firm philanthropic donations: evidence from the UK, 2022, 30, 1834-7649, 477, 10.1108/IJAIM-12-2021-0248 | |
2. | Chunhui Huo, Inzamam Ul Haq, Ji Wang, Nudging Toward Internal and External Origin Drivers: A Review of Corporate Green Innovation Research, 2024, 14, 2158-2440, 10.1177/21582440241288750 | |
3. | Longsheng Wu, Johnny F.I. Lam, Yinghan Liu, Can semi-mandatory non-financial disclosure requirements drive firms to improve ESG performance - evidence from Chinese listed companies, 2024, 10, 24058440, e34235, 10.1016/j.heliyon.2024.e34235 | |
4. | Yi Xu, Jiajun Qi, Jilong Chen, Lingbing Feng, Kick down the ladder? The impact of cryptocurrency bans on company legal risks and employee structure, 2025, 15446123, 107186, 10.1016/j.frl.2025.107186 |
Variables | Unit | Summary | Before Mandatory Disclosure | After Mandatory Disclosure |
CSR | - | −0.096 (1.954) | −0.941 (1.075) | 0.747 (2.248) |
CER | - | −0.088 (0.982) | −0.756 (0.531) | 0.579 (0.868) |
SOC | - | −0.010 (1.035) | −0.165 (0.404) | 0.143 (1.390) |
ECO | - | 0.002 (1.004) | −0.019 (0.710) | 0.024 (1.231) |
lnta | - | 8.874 (1.393) | 8.400 (1.241) | 9.349 (1.377) |
cd | 10 million ¥/employee | 0.159 (1.002) | 0.198 (1.317) | 0.121 (0.520) |
fr | percentage | 0.289 (0.201) | 0.316 (0.205) | 0.262 (0.195) |
owner | - | 0.789 (0.407) | 0.796 (0.403) | 0.784 (0.411) |
age | year | 13.482 (4.650) | 10.983 (3.922) | 15.982 (3.922) |
dar | percentage | 0.512 (0.179) | 0.487 (0.177) | 0.537 (0.178) |
tq | - | 1.669 (0.988) | 1.539 (0.849) | 1.799 (1.096) |
Primary Indicator | Secondary Indicator | Tertiary Indicator | Weight |
CER index | Law sense (0.1762) | Environmental penalties | 0.1762 |
Social evaluation (0.2228) | Environmental commendation | 0.0448 | |
Environmental advantages | 0.1779 | ||
Environment-friendly outputs (0.1957) | Circular economy | 0.0458 | |
Environment-friendly products | 0.0652 | ||
Pollutant discharge | 0.0847 | ||
Low-carbon technology (0.1357) | Energy saving | 0.0574 | |
Reduce three wastes measures | 0.0782 | ||
Green management (0.2696) | Environmental qualification | 0.0505 | |
Green office | 0.2192 |
Primary Indicator | Secondary Indicator | Tertiary Indicator |
Input index | Capital | Total assets |
Labor | Number of employees | |
Output index | Desirable output | Tax fee |
Staff salary | ||
Undesirable output | Frequency of litigation and arbitration | |
Frequency of discipline & regulations violations |
Primary Indicator | Secondary Indicator | Tertiary Indicator |
Input index | Capital | Total assets |
Labor | Number of employees | |
Output index | Desirable output | Earnings per share |
Main business revenue | ||
Undesirable output | Trading frequency | |
Frequency of shareholding pledge |
Items | CSR | CER | SOC | ECO |
PL | 0.881*** (5.72) | 1.230*** (19.43) | 0.128** (2.50) | −0.193** (−2.20) |
lnta | 0.416*** (4.00) | 0.181*** (4.23) | 0.089 (1.41) | 0.146** (2.46) |
cd | 0.143*** (3.60) | −0.006 (−0.39) | 0.084*** (3.48) | 0.065*** (2.88) |
fr | −0.939** (−2.42) | −0.114 (−0.71) | −0.086 (−0.37) | −0.738*** (−3.34) |
owner | 0.457* (1.66) | 0.114 (1.01) | 0.177 (1.06) | 0.166 (1.06) |
age | 0.081** (2.43) | −0.019 (−1.36) | 0.085*** (4.20) | 0.015 (0.77) |
dar | −1.641*** (−4.21) | −0.051 (−0.32) | −0.903*** (−3.82) | −0.687*** (−3.09) |
tq | 0.229*** (5.19) | 0.095*** (5.27) | 0.030 (1.11) | 0.104*** (4.13) |
Cons | −4.970 | −2.248 | −1.576 | −0.805*** (−5.53) |
N | 2056 | 2056 | 2056 | 2056 |
F | 104.66 | 342.78 | 15.87 | 7.89 |
R−squared | 0.3186 | 0.6049 | 0.0662 | 0.0340 |
Notes: *, **, *** denote significant levels of 10%, 5% and 1%, respectively. The values in brackets are T-statistics. |
Order | Covariate | CSR | CER | SOC | ECO |
First−order polynomial | Without covariates | 0.482** (2.37) |
1.148*** (10.85) |
−0.043 (−0.39) |
−0.434*** (−3.41) |
With covariates | 0.584*** (3.141) |
1.134*** (12.38) |
−0.017 (−0.26) |
−0.384*** (−3.32) |
|
Second−order polynomial | Without covariates | 0.254 (1.04) |
0.405** (4.67) |
−0.115 (−0.47) |
−0.729** (−2.16) |
With covariates | 0.312 (1.23) |
0.330*** (6.82) |
0.103 (0.46) |
−0.538* (−1.76) |
|
Notes: *, **, *** represent significant levels of 10%, 5% and 1%, respectively. The values in brackets are Z-statistics. |
Bandwidth | Covariate | CSR | CER | SOC | ECO |
±3 | Without covariates | 0.421** (2.01) |
0.900*** (6.04) |
−0.021 (−0.23) |
−0.532*** (−3.03) |
With covariates | 0.434** (2.23) |
0.855*** (6.63) |
−0.075 (−0.79) |
−0.444*** (−2.81) |
|
±2 | Without covariates | 0.497** (2.32) |
0.900*** (6.04) |
0.003 (0.04) |
−0.532*** (−3.03) |
With covariates | 0.477** (2.34) |
0.832*** (6.44) |
−0.045 (−0.48) |
−0.422*** (−2.67) |
|
Notes: *, **, *** stand for significant levels of 10%, 5% and 1%, respectively. The values in brackets are Z-statistics. |
Breakpoint | Covariate | CSR | CER | SOC | ECO |
2007 | Without covariates | 0.539*** (4.27) |
0.344*** (5.65) |
−0.054 (1.07) |
0.078 (1.15) |
With covariates | 0.421*** (3.76) |
0.370*** (7.18) |
−0.015 (−0.34) |
0.066 (0.83) |
|
2009 | Without covariates | −0.252 (−0.83) |
0.491*** (3.30) |
−0.023 (−0.51) |
−0.028 (−0.30) |
With covariates | −0.043 (0.14) |
0.421*** (3.09) |
−0.044 (−0.39) |
−0.021 (−0.23) |
|
Notes: *, **, *** denote significant levels of 10%, 5% and 1%, respectively. The values in brackets are Z-statistics. |
Algorithm | Covariate | CSR | CER | SOC | ECO |
Epanechnikov | Without covariates | 0.515** (2.55) |
1.174*** (11.34) |
−0.034 (−0.56) |
−0.424*** (−3.38) |
With covariates | 0.608*** (3.27) |
1.163*** (12.93) |
−0.026 (−0.49) |
−0.378*** (−3.31) |
|
Uniform | Without covariates | 0.893*** (5.42) |
1.232*** (15.35) |
−0.022 (−0.44) |
−0.184* (−1.78) |
With covariates | 0.896*** (5.81) |
1.226*** (17.23) |
−0.021 (−0.43) |
−0.188** (−1.95) |
|
Notes: *, **, *** mean significant levels of 10%, 5% and 1%, respectively. The values in brackets are Z-statistics. |
DEA model | Covariate | CSR | CER | SOC | ECO |
CCR−GML | Without covariates | 0.658*** (3.01) |
1.148*** (10.85) |
−0.037 (−0.37) |
−0.325** (−2.09) |
With covariates | 0.684*** (3.69) |
1.134*** (12.38) |
−0.024 (−0.28) |
−0.313** (−2.38) |
|
(CRS) Super−SBM−GML |
Without covariates | 0.619*** (3.16) |
1.148*** (10.85) |
−0.014 (−0.18) |
−0.386*** (−2.83) |
With covariates | 0.634*** (3.56) |
1.134*** (12.38) |
−0.005 (−0.07) |
−0.015*** (−3.06) |
|
(VRS) Super−SBM−GML |
Without covariates | 0.665*** (3.29) |
1.148*** (10.85) |
−0.015 (−0.16) |
−0.339*** (−2.82) |
With covariates | 0.718*** (4.15) |
1.134*** (12.38) |
0.015 (0.20) |
−0.318*** (−2.89) |
|
(CRS) SBM−GML |
Without covariates | 0.613*** (3.10) |
1.148*** (10.85) |
−0.014 (−0.17) |
−0.392*** (−2.84) |
With covariates | 0.627*** (3.49) |
1.134*** (12.38) |
−0.005 (−0.08) |
−0.389*** (−3.08) |
|
(VRS) SBM−GML |
Without covariates | 0.669*** (3.36) |
1.148*** (10.85) |
−0.007 (−0.08) |
−0.342*** (−2.83) |
With covariates | 0.715*** (4.14) |
1.134*** (12.38) |
0.018 (0.24) |
−0.324*** (−2.91) |
|
Notes: *, **, *** stand for significant levels of 10%, 5% and 1%, respectively. The values in brackets are Z-statistics. |
lnta | cd | fr | owner | age | dar | tq | |
Coef. | −0.098 (−0.68) |
−1.8 e+05 (−1.02) |
0.006 (0.27) |
0.016 (0.35) |
0.000 (0.00) |
−0.003 (−0.15) |
−0.277 (−1.23) |
Notes: the values in brackets are Z-statistics. |
Items | CER (1) | PLENV (2) | CER (3) |
PL | 1.198*** | 0.627*** | 0879*** |
PLENV | — | — | 0.716*** |
covariates | Yes | Yes | Yes |
Cons | −1.606 | −0.411 | −1.321 |
N | 2056 | 2056 | 2056 |
R-squared | 0.5774 | 0.6266 | 0.5780 |
Notes: *** stands for significant levels of 1%. |
Items | ECO (1) | ROA (2) | ECO (3) |
PL | −0.195*** | −0.027*** | −0.034 |
ROA | — | — | 5.980*** |
covariates | Yes | Yes | Yes |
Cons | −1.323 | 0.028 | −1.488 |
N | 2056 | 2056 | 2056 |
R−squared | 0.1285 | 0.4460 | 0.1877 |
Notes: *** stands for significant levels of 1%. |
Industry | Covariate | CSR | CER | SOC | ECO |
Heavy polluting industry | Without covariates | 0.287 (1.05) |
1.079*** (7.15) |
−0.056 (−0.60) |
−0.600*** (−1.80) |
With covariates | 0.334 (1.36) |
0.985*** (7.26) |
−0.054 (−0.66) |
−0.544*** (−1.74) |
|
Non-heavy polluting industries | Without covariates | 0.629** (2.18) |
1.200*** (8.82) |
−0.061 (−0.70) |
−0.310* (−1.80) |
With covariates | 0.772*** (2.94) |
1.247*** (10.45) |
−0.064 (−0.86) |
−0.264* (−1.74) |
|
Notes: **, *** denote significant levels of 10%, 5% and 1%, respectively. The values in brackets are Z-statistics. |
Owner | Covariate | CSR | CER | SOC | ECO |
State-owned enterprises | Without covariates | 0.300 (1.23) |
1.054*** (8.72) |
−0.034 (−0.46) |
−0.341** (−2.52) |
With covariates | 0.372* (1.68) |
1.031*** (10.06) |
−0.020 (−0.31) |
−0.336*** (−2.69) |
|
Non-state-owned enterprises | Without covariates | 1.151*** (3.71) |
1.493*** (6.98) |
0.019 (0.19) |
−0.172 (−1.29) |
With covariates | 1.297*** (5.61) |
1.516*** (9.28) |
0.021 (0.17) |
−0.175 (−1.59) |
|
Notes: **, *** mean significant levels of 5% and 1%, respectively. The values in brackets are Z-statistics. |
Variables | Unit | Summary | Before Mandatory Disclosure | After Mandatory Disclosure |
CSR | - | −0.096 (1.954) | −0.941 (1.075) | 0.747 (2.248) |
CER | - | −0.088 (0.982) | −0.756 (0.531) | 0.579 (0.868) |
SOC | - | −0.010 (1.035) | −0.165 (0.404) | 0.143 (1.390) |
ECO | - | 0.002 (1.004) | −0.019 (0.710) | 0.024 (1.231) |
lnta | - | 8.874 (1.393) | 8.400 (1.241) | 9.349 (1.377) |
cd | 10 million ¥/employee | 0.159 (1.002) | 0.198 (1.317) | 0.121 (0.520) |
fr | percentage | 0.289 (0.201) | 0.316 (0.205) | 0.262 (0.195) |
owner | - | 0.789 (0.407) | 0.796 (0.403) | 0.784 (0.411) |
age | year | 13.482 (4.650) | 10.983 (3.922) | 15.982 (3.922) |
dar | percentage | 0.512 (0.179) | 0.487 (0.177) | 0.537 (0.178) |
tq | - | 1.669 (0.988) | 1.539 (0.849) | 1.799 (1.096) |
Primary Indicator | Secondary Indicator | Tertiary Indicator | Weight |
CER index | Law sense (0.1762) | Environmental penalties | 0.1762 |
Social evaluation (0.2228) | Environmental commendation | 0.0448 | |
Environmental advantages | 0.1779 | ||
Environment-friendly outputs (0.1957) | Circular economy | 0.0458 | |
Environment-friendly products | 0.0652 | ||
Pollutant discharge | 0.0847 | ||
Low-carbon technology (0.1357) | Energy saving | 0.0574 | |
Reduce three wastes measures | 0.0782 | ||
Green management (0.2696) | Environmental qualification | 0.0505 | |
Green office | 0.2192 |
Primary Indicator | Secondary Indicator | Tertiary Indicator |
Input index | Capital | Total assets |
Labor | Number of employees | |
Output index | Desirable output | Tax fee |
Staff salary | ||
Undesirable output | Frequency of litigation and arbitration | |
Frequency of discipline & regulations violations |
Primary Indicator | Secondary Indicator | Tertiary Indicator |
Input index | Capital | Total assets |
Labor | Number of employees | |
Output index | Desirable output | Earnings per share |
Main business revenue | ||
Undesirable output | Trading frequency | |
Frequency of shareholding pledge |
Items | CSR | CER | SOC | ECO |
PL | 0.881*** (5.72) | 1.230*** (19.43) | 0.128** (2.50) | −0.193** (−2.20) |
lnta | 0.416*** (4.00) | 0.181*** (4.23) | 0.089 (1.41) | 0.146** (2.46) |
cd | 0.143*** (3.60) | −0.006 (−0.39) | 0.084*** (3.48) | 0.065*** (2.88) |
fr | −0.939** (−2.42) | −0.114 (−0.71) | −0.086 (−0.37) | −0.738*** (−3.34) |
owner | 0.457* (1.66) | 0.114 (1.01) | 0.177 (1.06) | 0.166 (1.06) |
age | 0.081** (2.43) | −0.019 (−1.36) | 0.085*** (4.20) | 0.015 (0.77) |
dar | −1.641*** (−4.21) | −0.051 (−0.32) | −0.903*** (−3.82) | −0.687*** (−3.09) |
tq | 0.229*** (5.19) | 0.095*** (5.27) | 0.030 (1.11) | 0.104*** (4.13) |
Cons | −4.970 | −2.248 | −1.576 | −0.805*** (−5.53) |
N | 2056 | 2056 | 2056 | 2056 |
F | 104.66 | 342.78 | 15.87 | 7.89 |
R−squared | 0.3186 | 0.6049 | 0.0662 | 0.0340 |
Notes: *, **, *** denote significant levels of 10%, 5% and 1%, respectively. The values in brackets are T-statistics. |
Order | Covariate | CSR | CER | SOC | ECO |
First−order polynomial | Without covariates | 0.482** (2.37) |
1.148*** (10.85) |
−0.043 (−0.39) |
−0.434*** (−3.41) |
With covariates | 0.584*** (3.141) |
1.134*** (12.38) |
−0.017 (−0.26) |
−0.384*** (−3.32) |
|
Second−order polynomial | Without covariates | 0.254 (1.04) |
0.405** (4.67) |
−0.115 (−0.47) |
−0.729** (−2.16) |
With covariates | 0.312 (1.23) |
0.330*** (6.82) |
0.103 (0.46) |
−0.538* (−1.76) |
|
Notes: *, **, *** represent significant levels of 10%, 5% and 1%, respectively. The values in brackets are Z-statistics. |
Bandwidth | Covariate | CSR | CER | SOC | ECO |
±3 | Without covariates | 0.421** (2.01) |
0.900*** (6.04) |
−0.021 (−0.23) |
−0.532*** (−3.03) |
With covariates | 0.434** (2.23) |
0.855*** (6.63) |
−0.075 (−0.79) |
−0.444*** (−2.81) |
|
±2 | Without covariates | 0.497** (2.32) |
0.900*** (6.04) |
0.003 (0.04) |
−0.532*** (−3.03) |
With covariates | 0.477** (2.34) |
0.832*** (6.44) |
−0.045 (−0.48) |
−0.422*** (−2.67) |
|
Notes: *, **, *** stand for significant levels of 10%, 5% and 1%, respectively. The values in brackets are Z-statistics. |
Breakpoint | Covariate | CSR | CER | SOC | ECO |
2007 | Without covariates | 0.539*** (4.27) |
0.344*** (5.65) |
−0.054 (1.07) |
0.078 (1.15) |
With covariates | 0.421*** (3.76) |
0.370*** (7.18) |
−0.015 (−0.34) |
0.066 (0.83) |
|
2009 | Without covariates | −0.252 (−0.83) |
0.491*** (3.30) |
−0.023 (−0.51) |
−0.028 (−0.30) |
With covariates | −0.043 (0.14) |
0.421*** (3.09) |
−0.044 (−0.39) |
−0.021 (−0.23) |
|
Notes: *, **, *** denote significant levels of 10%, 5% and 1%, respectively. The values in brackets are Z-statistics. |
Algorithm | Covariate | CSR | CER | SOC | ECO |
Epanechnikov | Without covariates | 0.515** (2.55) |
1.174*** (11.34) |
−0.034 (−0.56) |
−0.424*** (−3.38) |
With covariates | 0.608*** (3.27) |
1.163*** (12.93) |
−0.026 (−0.49) |
−0.378*** (−3.31) |
|
Uniform | Without covariates | 0.893*** (5.42) |
1.232*** (15.35) |
−0.022 (−0.44) |
−0.184* (−1.78) |
With covariates | 0.896*** (5.81) |
1.226*** (17.23) |
−0.021 (−0.43) |
−0.188** (−1.95) |
|
Notes: *, **, *** mean significant levels of 10%, 5% and 1%, respectively. The values in brackets are Z-statistics. |
DEA model | Covariate | CSR | CER | SOC | ECO |
CCR−GML | Without covariates | 0.658*** (3.01) |
1.148*** (10.85) |
−0.037 (−0.37) |
−0.325** (−2.09) |
With covariates | 0.684*** (3.69) |
1.134*** (12.38) |
−0.024 (−0.28) |
−0.313** (−2.38) |
|
(CRS) Super−SBM−GML |
Without covariates | 0.619*** (3.16) |
1.148*** (10.85) |
−0.014 (−0.18) |
−0.386*** (−2.83) |
With covariates | 0.634*** (3.56) |
1.134*** (12.38) |
−0.005 (−0.07) |
−0.015*** (−3.06) |
|
(VRS) Super−SBM−GML |
Without covariates | 0.665*** (3.29) |
1.148*** (10.85) |
−0.015 (−0.16) |
−0.339*** (−2.82) |
With covariates | 0.718*** (4.15) |
1.134*** (12.38) |
0.015 (0.20) |
−0.318*** (−2.89) |
|
(CRS) SBM−GML |
Without covariates | 0.613*** (3.10) |
1.148*** (10.85) |
−0.014 (−0.17) |
−0.392*** (−2.84) |
With covariates | 0.627*** (3.49) |
1.134*** (12.38) |
−0.005 (−0.08) |
−0.389*** (−3.08) |
|
(VRS) SBM−GML |
Without covariates | 0.669*** (3.36) |
1.148*** (10.85) |
−0.007 (−0.08) |
−0.342*** (−2.83) |
With covariates | 0.715*** (4.14) |
1.134*** (12.38) |
0.018 (0.24) |
−0.324*** (−2.91) |
|
Notes: *, **, *** stand for significant levels of 10%, 5% and 1%, respectively. The values in brackets are Z-statistics. |
lnta | cd | fr | owner | age | dar | tq | |
Coef. | −0.098 (−0.68) |
−1.8 e+05 (−1.02) |
0.006 (0.27) |
0.016 (0.35) |
0.000 (0.00) |
−0.003 (−0.15) |
−0.277 (−1.23) |
Notes: the values in brackets are Z-statistics. |
Items | CER (1) | PLENV (2) | CER (3) |
PL | 1.198*** | 0.627*** | 0879*** |
PLENV | — | — | 0.716*** |
covariates | Yes | Yes | Yes |
Cons | −1.606 | −0.411 | −1.321 |
N | 2056 | 2056 | 2056 |
R-squared | 0.5774 | 0.6266 | 0.5780 |
Notes: *** stands for significant levels of 1%. |
Items | ECO (1) | ROA (2) | ECO (3) |
PL | −0.195*** | −0.027*** | −0.034 |
ROA | — | — | 5.980*** |
covariates | Yes | Yes | Yes |
Cons | −1.323 | 0.028 | −1.488 |
N | 2056 | 2056 | 2056 |
R−squared | 0.1285 | 0.4460 | 0.1877 |
Notes: *** stands for significant levels of 1%. |
Industry | Covariate | CSR | CER | SOC | ECO |
Heavy polluting industry | Without covariates | 0.287 (1.05) |
1.079*** (7.15) |
−0.056 (−0.60) |
−0.600*** (−1.80) |
With covariates | 0.334 (1.36) |
0.985*** (7.26) |
−0.054 (−0.66) |
−0.544*** (−1.74) |
|
Non-heavy polluting industries | Without covariates | 0.629** (2.18) |
1.200*** (8.82) |
−0.061 (−0.70) |
−0.310* (−1.80) |
With covariates | 0.772*** (2.94) |
1.247*** (10.45) |
−0.064 (−0.86) |
−0.264* (−1.74) |
|
Notes: **, *** denote significant levels of 10%, 5% and 1%, respectively. The values in brackets are Z-statistics. |
Owner | Covariate | CSR | CER | SOC | ECO |
State-owned enterprises | Without covariates | 0.300 (1.23) |
1.054*** (8.72) |
−0.034 (−0.46) |
−0.341** (−2.52) |
With covariates | 0.372* (1.68) |
1.031*** (10.06) |
−0.020 (−0.31) |
−0.336*** (−2.69) |
|
Non-state-owned enterprises | Without covariates | 1.151*** (3.71) |
1.493*** (6.98) |
0.019 (0.19) |
−0.172 (−1.29) |
With covariates | 1.297*** (5.61) |
1.516*** (9.28) |
0.021 (0.17) |
−0.175 (−1.59) |
|
Notes: **, *** mean significant levels of 5% and 1%, respectively. The values in brackets are Z-statistics. |