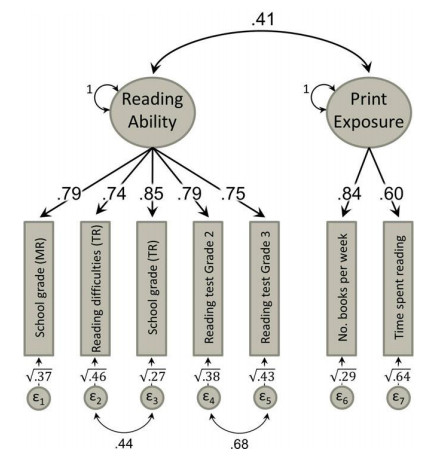
The paper established a differential equation model for 194 children with ADHD in outpatient clinics from September 2019 to August 2020 and compiled a children's clinical diagnostic interview scale based on the fourth edition of the American Diagnostic and Statistical Manual of Mental Disorders (DSM-Ⅳ). The CDIS standard divides it into three phenotypes: attention deficit predominant (ADHD-I), hyperactivity-impulsive predominance (ADHD-HI) and mixed (ADHD-C). The results of the study showed that the distribution of subtypes in the study cases: ADHD-I accounted for 45.9% (89 cases), ADHD-HI accounted for 7.7% (15 cases), ADHD-C accounted for 46.4% (90 cases); ADHD-C: ADHD-I is 1:1. CDIS scale total score: 194 cases of attention deficit symptoms were (7.2 ± 1.4) points, and hyperactivity-impulsive symptoms were (5.4 ± 2.2) points. The frequency of attention deficit symptoms in 194 cases was (79.5 ± 2.9) %, and the frequency of hyperactivity-impulsive symptoms was (59.8 ± 3.5) %. Therefore, it can be concluded that DSM-IV defines three phenotypes in this sample. The proportion of ADHD-HI is low, and the proportion of ADHD-I and ADHD-C is similar; age influences the phenotype distribution.
Citation: Wei Zhang, Ai Ma, Aseel Takshe, Bishr Muhamed Muwafak. Establishment of differential model of recovery treatment for children with minor brain injury and mental disorder syndrome[J]. Mathematical Biosciences and Engineering, 2021, 18(5): 5614-5624. doi: 10.3934/mbe.2021283
[1] | Austin Baird, Laura Oelsner, Charles Fisher, Matt Witte, My Huynh . A multiscale computational model of angiogenesis after traumatic brain injury, investigating the role location plays in volumetric recovery. Mathematical Biosciences and Engineering, 2021, 18(4): 3227-3257. doi: 10.3934/mbe.2021161 |
[2] | Rong Qiang, Wanbiao Ma, Ke Guo, Hongwu Du . The differential equation model of pathogenesis of Kawasaki disease with theoretical analysis. Mathematical Biosciences and Engineering, 2019, 16(5): 3488-3511. doi: 10.3934/mbe.2019175 |
[3] | Zijian Wang, Yaqin Zhu, Haibo Shi, Yanting Zhang, Cairong Yan . A 3D multiscale view convolutional neural network with attention for mental disease diagnosis on MRI images. Mathematical Biosciences and Engineering, 2021, 18(5): 6978-6994. doi: 10.3934/mbe.2021347 |
[4] | Rong Qiang, Wanbiao Ma, Ke Guo, Hongwu Du . The differential equation model of pathogenesis of Kawasaki disease with theoretical analysis. Mathematical Biosciences and Engineering, ${relative.year}, (): 3488-3511. doi: 10.3934/mbe.2019175 |
[5] | Justin Gomez, Nathanael Holmes, Austin Hansen, Vikram Adhikarla, Margarita Gutova, Russell C. Rockne, Heyrim Cho . Mathematical modeling of therapeutic neural stem cell migration in mouse brain with and without brain tumors. Mathematical Biosciences and Engineering, 2022, 19(3): 2592-2615. doi: 10.3934/mbe.2022119 |
[6] | Nannan Long, Yongxiang Lei, Lianhua Peng, Ping Xu, Ping Mao . A scoping review on monitoring mental health using smart wearable devices. Mathematical Biosciences and Engineering, 2022, 19(8): 7899-7919. doi: 10.3934/mbe.2022369 |
[7] | Sandra Cole, M. Foster Olive, Stephen Wirkus . The dynamics of heroin and illicit opioid use disorder, casual use, treatment, and recovery: A mathematical modeling analysis. Mathematical Biosciences and Engineering, 2024, 21(2): 3165-3206. doi: 10.3934/mbe.2024141 |
[8] | Rui Zhu, Yuhang Chen, Qianqian Yu, Siqing Liu, Jianjie Wang, Zhili Zeng, Liming Cheng . Effects of contusion load on cervical spinal cord: A finite element study. Mathematical Biosciences and Engineering, 2020, 17(3): 2272-2283. doi: 10.3934/mbe.2020120 |
[9] | Jiaxi Lu, Yingwei Guo, Mingming Wang, Yu Luo, Xueqiang Zeng, Xiaoqiang Miao, Asim Zaman, Huihui Yang, Anbo Cao, Yan Kang . Determining acute ischemic stroke onset time using machine learning and radiomics features of infarct lesions and whole brain. Mathematical Biosciences and Engineering, 2024, 21(1): 34-48. doi: 10.3934/mbe.2024002 |
[10] | Hangle Hu, Chunlei Cheng, Qing Ye, Lin Peng, Youzhi Shen . Enhancing traditional Chinese medicine diagnostics: Integrating ontological knowledge for multi-label symptom entity classification. Mathematical Biosciences and Engineering, 2024, 21(1): 369-391. doi: 10.3934/mbe.2024017 |
The paper established a differential equation model for 194 children with ADHD in outpatient clinics from September 2019 to August 2020 and compiled a children's clinical diagnostic interview scale based on the fourth edition of the American Diagnostic and Statistical Manual of Mental Disorders (DSM-Ⅳ). The CDIS standard divides it into three phenotypes: attention deficit predominant (ADHD-I), hyperactivity-impulsive predominance (ADHD-HI) and mixed (ADHD-C). The results of the study showed that the distribution of subtypes in the study cases: ADHD-I accounted for 45.9% (89 cases), ADHD-HI accounted for 7.7% (15 cases), ADHD-C accounted for 46.4% (90 cases); ADHD-C: ADHD-I is 1:1. CDIS scale total score: 194 cases of attention deficit symptoms were (7.2 ± 1.4) points, and hyperactivity-impulsive symptoms were (5.4 ± 2.2) points. The frequency of attention deficit symptoms in 194 cases was (79.5 ± 2.9) %, and the frequency of hyperactivity-impulsive symptoms was (59.8 ± 3.5) %. Therefore, it can be concluded that DSM-IV defines three phenotypes in this sample. The proportion of ADHD-HI is low, and the proportion of ADHD-I and ADHD-C is similar; age influences the phenotype distribution.
Recent studies have shown that children with attention deficit hyperactivity disorder (ADHD) have heterogeneous characteristics, and different subtypes have different prognoses. Therefore, the descriptive study of clinical classification and its characteristics is of great significance for exploring its etiology, prognosis and treatment [1]. The American Diagnostic and Statistical Manual of Mental Disorders Fourth Edition (DSM-Ⅳ) divides ADHD into three phenotypes according to the symptom dimension: (1) Attention deficit-based (ADHDI). It is mainly manifested as difficulty maintaining concentration, ease to be distracted, beginning and end in doing things, and disorganized daily life. (2) Hyperactivity-impulsive predominance (ADHD-HI). Mainly manifested as excessive activity, noise and impatience. (3) Hybrid (ADHD-C). Symptoms of attention deficit and hyperactivity are more prominent. There is no subtype in the classification scheme and diagnostic criteria of mental illness in China. This study aims to explore the clinical subtypes and characteristics of children with ADHD under the cultural background of our country and provide a scientific basis for diagnosing and treating ADHD.
The cases selected in the paper are children who went to the hospital outpatient clinic from October 2019 to August 2020. According to the DSM-IV diagnostic criteria, the patient was first diagnosed with ADHD by two psychiatrists (one of whom was the deputy chief physician or above), without knowing each other. Then the children's clinical diagnostic interviews were used for those with the same diagnosis. Exclude hyperactivity or attention deficits caused by the nervous system's organic diseases, extensive developmental disorders, mental retardation, psychotic disorders, or other primary mental disorders (such as to conduct, mood disorders, anxiety or dissociative disorders). A total of 194 cases met the criteria [2]. Among them, 161 cases were male (83.4%), 32 cases were female (16.6%), male: female was 5:1; age was 6–16 years old, average (10.3 ± 2.3) years old; course of the disease was 0.5–15.0 years, the average was (5 ± 2.3) 3 years.
The Children's Clinical Diagnostic Interview Scale compiled by the American Child Disorders Working Group is introduced. The scale is based on the DSM-IV diagnostic criteria and consists of four criteria: symptom criteria, disease course criteria, severity criteria and exclusion criteria. In the symptom standard, each symptom is divided into two levels: "Yes" and "No". Yes: refers to the symptom that frequently occurs and scores 1; No: refers to the occasional or never occurrence of this symptom, and scores 0. The attention-deficit factor is composed of 9 attention deficit symptoms, and the other is the hyperactivity-impulsive factor composed of 9 hyperactivity-impulsive symptoms, and the scoring range is 0–9 points. The scale is a fixed-form interview scale, which a psychiatrist and parents evaluate interviewed [3]. After field-testing abroad, ADHD is divided into four subtypes according to the characteristics of symptom distribution: (1) ADHD-C is classified as attention deficit symptoms and hyperactivity-impulsive symptoms ≥ 6; (2) only attention deficit symptoms ≥ 6; (3) Only those with hyperactivity-impulsive symptoms ≥ six are considered as ADHD-HI; (4) Those who do not meet the symptom criteria of the above three types, but have obvious clinical manifestations, are untyped (because the untyped does not meet The diagnostic criteria of ADHD in DSM-IV are not discussed in this article). The scale has been translated and revised and initially tested 109 children with outpatient complaints of hyperactivity, inattention, and learning difficulties. The sensitivity was 97.2%, the specificity was 100%, the test-retest reliability PA = 0.89, and the inter-rater consistency Kappa = 0.74 (P < 0.01), which meets the needs of research.
The paper uses the epidemiological research data management and analysis system software (EPI INFO software) compiled by the National Center for Disease Control and the World Health Organization. Statistical analysis uses χ2 test, t-test, and analysis of variance.
The thesis assumes that the intensity of the consciousness transfer before the client did not receive the consultation is m, and the intensity of the consciousness transfer after the consultation is M. The intensity of the consciousness transfer of the client after the first term is attacked is:
P1=[(M−m)/V1]×P0(P0=1) | (1) |
After the progressive term after the previous alternative is attacked, the intensity of the person's consciousness transfer can be obtained for the same reason as the above formula:
P2=[(M−m)/(V2+V1)]×P1 | (2) |
At this time, there is a functional relationship between the intensity of the party's consciousness transfer after the previous term was attacked and the intensity of the party's consciousness transfer when the term was attacked again. k(vx),k(vx) is a function that increases as the consultation process runs:
Ps=K(Vx)×Px | (3) |
It can be supposed that after the client's number of terms has reached a certain cumulative level, Px at this time is the intensity of consciousness transfer of the client to be reached in the consultation, and Ps is the intensity of consciousness transfer derived from the client's consultation [4]. The intensityPx of the party's self-consciousness transfer will increase due to the injection from the consultation, as shown in Figure 1.
Assuming that the change of the party's consciousness orientation (in terms of data, the increase in the intensity of consciousness transfer) is a flow process of development and operation, the intensity of the party's self-derived consciousness transfer is relative to the increased rate of the attack on the terms derived from the consultation dPx/dVx It is proportional to the intensity of the person's source of self-consciousness and the intensity difference Px−0 before the attacked termα, that is
dPxdVx=a(Px−0)lnPx=aVx+cPX=eaVx+c | (4) |
The paper uses boundary conditions to determine the parameter c
Substitute boundary condition VX=0,Px=P2
P2=ecc=lnP2 | (5) |
Have
Ps=k(vx)×eavx+lnP2 | (6) |
After obtaining the consciousness transfer intensity function Ps of the party's self, we calculate the integral of the lexical item alternative quantity Vxby Ps, and we can obtain the party's consciousness transfer intensity function F(vx) that the lexical item alternative is attacked.
F(vx)=∫vx0Psdvx | (7) |
Here, it is needed to determine the ratio of the parameter k(vx) (the ratio of the intensity of the party's consciousness transfer before the progressive term is attacked to the intensity of the party's consciousness transfer before the attacked term). The parameter α. k(vx) is a proportional function of the intensity of the consciousness transfer of the client after the progressive process of the consultation term [5]. Before the unaccepted vocabulary is attacked, k(vx) is a small amount. As the accepted vocabulary is attacked, k(vx) increases continuously. The intensity of the consciousness transfer of the party is equal to the intensity of the consciousness transfer before the unconsented (This is the completion of a thing from a quantitative change to a qualitative change, moving towards the opposite of the thing, and the new thing negating the old thing). It can be assumed that when vx = 0, that is, at the beginning of the consultation, k(vx) is relatively most miniature and [k(vx)]min is taken; after the consultation, [k(vx)]max can be measured by the party's self-satisfaction with the consultation effect), as shown in Figure 2. In the model hypothesis, considering the fixation of the client's conscious tendency, the intensity of the client's consciousness transfer from receiving counseling is three times the intensity of the consciousness transfer from the self. The functional relationship can be established:
k(vx)=3+e−vx+2 | (8) |
When vx→∞,[k(vx)]min; when vx=0, [k(vx)]min and the function is incremented. The parameter α can be calculated from the ratio of the number of optional cumulative terms for any N times and the intensity of the person's consciousness transfer from the consultation, and the average value is used. After the parameter [k(vx),a] is determined, the analytical formula of F(vx) is
F(vx)=∫vx0(3e−vx+2)eavx+lnP2dvx | (9) |
It can be seen that the change function of the intensity of consciousness transfer after the party accepts the vocabulary is attacked. g(vx)[g(vx)=. The number of times the vocabulary has been attacked [6]. The alternative cumulative quantity of the vocabulary is
g(vx)=r−p1v1−F(vx)v2+M−m | (10) |
g(vx)=E | (11) |
The cumulative total of available terms (term flow) is as follows:
W=v1+v2+vx | (12) |
Among 194 cases, 89 cases (45.9%) met ADHD-I, 15 cases (7.7%) met ADHD-HI, and 90 cases (46.4%) met ADHD-C. The ratio of ADHD-C: ADHD-I is 1:1.
The total score of attention deficit symptoms of 194 cases was (7.2 ± 1.4) points, and the total score of hyperactivity-impulsive symptoms was (5.4 ± 2.2) points. (1) Comparison between the three phenotypes: In the total score of attention deficit symptoms, ADHD-I is (7.2 ± 1.0) points, ADHD-HI is (4.1 ± 1.3) points, and ADHD-C is (7.6 ± 1.1) points; After the analysis of variance, the differences between the three groups are very significant (P < 0.01). (2) Comparison between different genders and different ages: the total scores of male and female children with attention deficit symptoms are (7.1 ± 1.4) points and (7.5 ± 1.3) points, respectively, and the total scores of hyperactivity-impulsive symptoms are (5.5 ± 2.2) points, respectively and (5.0 ± 2.3) points. The difference was not significant (P > 0.05). Different ages have a particular influence on the total score of attention deficit symptoms (P = 0.05); the influence of the hyperactivity-impulsive symptom factor, the analysis of variance, shows that the total symptom score gradually decreases with age 6–8 years old, 9–12 years old, 9–12 Years old and 13–16 years old were (6.0 ± 2.2), (5.5 ± 2.1) and (3.9 ± 2.0) points, respectively; P < 0.01.
Table 1 shows that in the total sample of ADHD, the frequency of 9 attention deficit symptoms exceeds 60.0%. Among the nine hyperactivity-impulsive symptoms, only 4 (small movements, leaving the seat on inappropriate occasions, talking the frequency of occurrence is more than 60.0%, which indicates that the children enrolled in the group have more symptoms of attention deficit and more minor hyperactivity-impulsive symptoms [7].
Core symptoms | ADHD | ADHD-I | χ2 | ||
Number | % | Number | % | ||
Attention-deficit symptoms | |||||
Not paying attention to details | 181 | 93.3 | 82 | 92.1 | 12.64 |
Pay attention to keep it difficult | 169 | 87.1 | 82 | 92.1 | 32.33 |
Not listening | 146 | 75.3 | 62 | 69.7 | 9.45 |
Can't always follow instructions | 153 | 78.9 | 74 | 83.1 | 14.84 |
Impaired organization | 169 | 87.1 | 79 | 88.8 | 24.17 |
Unwilling to do homework | 139 | 71.6 | 64 | 71.9 | 8.52 |
Often lose things | 128 | 66 | 62 | 69.7 | 11.21 |
easily distracted | 182 | 93.8 | 84 | 94.4 | 12.15 |
Forget things often | 121 | 62.4 | 54 | 60.7 | 18.27 |
Average frequency | 79.5 ± 2.9 | 80.3 ± 2.9 | 11.23 | ||
Hyperactivity-impulsive symptoms | |||||
Many small movements | 171 | 88.1 | 69 | 77.5 | 17.87 |
Leave your seat on inappropriate occasions | 128 | 66 | 42 | 47.2 | 26.04 |
Running around in inappropriate situations | 115 | 59.3 | 32 | 36 | 37.48 |
Uproar | 65 | 33.5 | 12 | 13.5 | 30.3 |
Overactive | 110 | 56.7 | 32 | 36 | 29.06 |
Talkative | 127 | 65.5 | 36 | 40.4 | 45.85 |
Rob talk | 113 | 58.2 | 28 | 31.5 | 48.93 |
Can't wait in line | 94 | 48.5 | 18 | 20.2 | 52.87 |
Disturb others | 121 | 62.4 | 35 | 39.3 | 38.94 |
Average frequency | 59.8 ± 3.5 | 38.0 ± 3.5 | 31.91 | ||
Core symptoms | ADHD-HI | ADHD-C | P | ||
Number | % | Number | % | ||
Attention-deficit symptoms | |||||
Not paying attention to details | 11 | 73.3 | 88 | 97.8 | < 0.01 |
Pay attention to keep it difficult | 6 | 40 | 81 | 90 | < 0.01 |
Not listening | 8 | 53.3 | 76 | 84.4 | < 0.01 |
Can't always follow instructions | 6 | 40 | 73 | 81.1 | < 0.01 |
Impaired organization | 7 | 46.7 | 83 | 92.2 | < 0.01 |
Unwilling to do homework | 6 | 40 | 69 | 76.7 | < 0.05 |
Often lose things | 4 | 26.7 | 62 | 68.9 | < 0.01 |
Easily distracted | 11 | 73.3 | 87 | 96.7 | < 0.01 |
Forget things often | 2 | 13.3 | 65 | 72.2 | < 0.01 |
Average frequency | 45.2 ± 3.6 | 84.4 ± 2.6 | < 0.01 | ||
Hyperactivity-impulsive symptoms | |||||
Many small movements | 15 | 100 | 87 | 96.7 | < 0.01 |
Leave your seat on inappropriate occasions | 13 | 86.7 | 73 | 81.1 | < 0.01 |
Running around in inappropriate situations | 13 | 86.7 | 70 | 77.8 | < 0.01 |
Uproar | 9 | 60 | 44 | 48.9 | < 0.01 |
Overactive | 12 | 80 | 66 | 73.3 | < 0.01 |
talkative | 12 | 80 | 79 | 87.8 | < 0.01 |
Rob talk | 11 | 73.3 | 74 | 82.2 | < 0.01 |
Can't wait in line | 12 | 80 | 64 | 71.1 | < 0.01 |
Disturb others | 10 | 66.7 | 76 | 84.4 | < 0.01 |
Average frequency | 79.3 ± 2.9 | 78.1 ± 3.0 | < 0.01 |
The elemental distribution of the frequency of the three phenotypic symptoms, (1) ADHD-I: In the frequency of attention deficit symptoms, nine items are more than 60%; in the frequency of hyperactivity-impulsive symptoms, except for 1 item (more small movements), more than 70.0%, eight items are all lower than 50.0%. (2) ADHD-HI: In the frequency of attention deficit symptoms, except for three items (not paying attention to details, easy to be distracted, and hearing but not listening), the rest are less than 50.0%; in the frequency of hyperactivity-impulsive symptoms, nine items Both exceed 60%. (3) ADHD-C: Among the 18 symptom frequencies, except for 2 (noisy and frequent loss of things), 16 more than 70.0%. The three types have significant differences in the frequency of various symptoms (P < 0.05).
Children aged 6–8, 9–12, and 13–16 (described in this order below) increase with age, seem to hear but not hear (77.8%, 81.4%, 52.9%, respectively), and have more small movements (93.7%, 90.7%, 70.6%, respectively), running around in inappropriate situations (79.4%, 53.6%, 38.2%, respectively), loud (39.7%, 37.1%, 11.8%, respectively), and hyperactivity (74.6%, respectively), 57.7%, 20.6%) decreased (P < 0.01 or P < 0.05), and the frequency of reluctance to do homework increased (57.1%, 76.3%, 85.3%, respectively; P < 0.01).
Among the three phenotypes, the comparison of the distribution of different genders and ages is shown in Table 2. In boys and girls, the three phenotypes' frequency is similar, suggesting that gender does not affect the phenotype [8]. As age increases, ADHDHI and ADHD-C decrease, while ADHD-I increases.
Male | Female | χ2 | P | ||
ADHD-C | 90(46.4) | 75(46.3) | 15(46.9) | 0.02 | > 0.05 |
ADHD-I | 89(45.9) | 73(45.1) | 16(50.0) | 0.1 | > 0.05 |
ADHD-HI | 15(7.7) | 14(8.6) | 1(3.1) | 1.15 | > 0.05 |
Total | 194 | 162 | 32 | ||
6–8 | 9–12 | 13–16 | χ2 | P | |
ADHD-C | 31(49.2) | 51(52.6) | 8(23.5) | 8.84 | < 0.05 |
ADHD-I | 22(34.9) | 42(43.3) | 25(73.5) | 13.78 | < 0.01 |
ADHD-HI | 10(15.9) | 4(4.1) | 1(2.9) | 8.72 | < 0.05 |
Total | 63 | 97 | 34 |
We used the DSM-IV symptom criteria to investigate the distribution of symptoms in children with ADHD and found that there were more symptoms of attention deficit and fewer hyperactivity-impulsive symptoms, suggesting that the level of hyperactivity and impulse in this group of ADHD children is relatively low, that is, the amount of activity is relatively high. Slight and less urgency to the stimulus. This conclusion is similar to the literature [9] in the cross-cultural comparison of samples of children with ADHD in China (Hong Kong) and the United Kingdom. Some scholars have found in a cross-ethnic comparison study of infant temperament that Chinese 4-month-old babies have lower motor activity and irritability than European and American white babies of the same age; this suggests that babies of different races have behavioral characteristics. There are inherent differences. Some scholars found the distribution of Chinese children's temperament types compared with foreign data. Chinese children have fewer difficult-to-nourish types; speculation may be related to ethnic background and cultural environment. Therefore, the characteristics mentioned earlier should be considered when formulating the clinical diagnostic criteria for ADHD in my country. It is necessary to formulate appropriate standards using our local norms.
In this study, the same evaluation tools as similar foreign studies is used, and the results are comparable to a certain extent. According to literature reports in the United States, ADHD-I accounted for 28.0%–30.0% of children with ADHD, ADHD-C accounted for 61.0–64.7%, and ADHD-HI accounted for 7.3%–9.0%; in this study, the three types accounted for 45.9%, 46.4%, 7.7%. In the sample of this study, the proportion of ADHD-I is more than that of the United States, ADHD-C is less than that of the United States, and the proportion of ADHD-HI is similar to that of the United States; the ratio of the number of ADHD-C to ADHD-I in the United States is about 2:1, This group is 1:1. The possible reasons are: (1) Combined with the results of core symptom analysis, this group of children with ADHD has a lower level of hyperactivity impulse, leading to an increase in the proportion of ADHD-I; (2) The age composition of the sample will also affect the proportion of each subtype.
This study shows that age has a significant impact on the distribution of core symptoms and phenotypes. As age increases, symptoms of hyperactivity decrease, and learning problems increase. ADHD-HI is more in the 6–8 years old group, and it is significantly reduced over nine years old; ADHD-C is more in 6–12 years old, the most is 9–12 years old, and it decreases over 13 years old; while ADHD-I is significantly more than 13 years old in the young age group. This is similar to the research results of many scholars at home and abroad [10]. Some scholars believe that the symptoms of hyperactivity can be observed at a young age, and attention deficits can only arouse the attention of adults when the children go to school, the academic pressure increases and the demand for attention increases; ADHD-HI is likely to be in ADHD-C Part of the children who went to the doctor early did not find the symptoms of attention-deficit when they went to the doctor. With the increase of age, the attention deficit showed up, which could be attributed to ADHD-C. Through a review of the previous literature, some scholars found that the attention deficit disorder of children with hyperactivity remained relatively stable with age, while the hyperactivity-impulsive symptoms gradually decreased.
To confirm the stable subtype, the influence of age must be considered. The stage with the most abundant symptoms in the entire course of the disease should be selected. The age group with both hyperactivity and attention deficit is the basis for diagnostic classification, generally between 8–12 years old. Because this study is the first domestic use of DSM-IV diagnostic classification criteria for clinical classification of ADHD, the above results need to be expanded and repeated experimental verification.
The authors declare no conflict of interest.
[1] |
D. L. Van Praag, M. C. Cnossen, S. Polinder, L. Wilson, A. I. Maas, Post-traumatic stress disorder after civilian traumatic brain injury: A systematic review and meta-analysis of prevalence rates, J. Neurotrauma, 36 (2019), 3220-3232. doi: 10.1089/neu.2018.5759
![]() |
[2] | J. J. Barton, P. J. Ranalli, Vision therapy: ocular motor training in mild traumatic brain injury. Ann. Neurol., 88 (2020), 453-461. |
[3] | R. J. Novorolsky, M. Nichols, J. S. Kim, E. V. Pavlov, J. J. Woods, J. J. Wilson, et al., The cell-permeable mitochondrial calcium uniporter inhibitor Ru265 preserves cortical neuron respiration after lethal oxygen glucose deprivation and reduces hypoxic/ischemic brain injury, J. Cereb. Blood Flow Metab., 40 (2020), 1172-1181. |
[4] | D. Pavlovic, S. Pekic, M. Stojanovic, V. Popovic, Traumatic brain injury: neuropathological, neurocognitive and neurobehavioral sequelae, Pituitary, 22 (2019), 270-282. |
[5] | N. Kreitzer, R. Ancona, C. McCullumsmith, B. G. Kurowski, B. Foreman, L. B. Ngwenya, et al., The effect of antidepressants on depression after traumatic brain injury: a meta-analysis. J. Head trauma Rehabil., 34 (2019), E47-E54. |
[6] |
A. Slowinski, R. Coetzer, C. Byrne, Pharmacotherapy effectiveness in treating depression after traumatic brain injury: a meta-analysis, J. Neuropsychiatry Clin. Neurosci., 31 (2019), 220-227. doi: 10.1176/appi.neuropsych.18070158
![]() |
[7] | C. J. Nederpelt, S. J. van der Aalst, M. G. Rosenthal, P. Krijnen, M. V. Huisman, W. C. Peul, et al., Consequences of pre-injury utilization of direct oral anticoagulants in patients with traumatic brain injury: A systematic review and meta-analysis, J. Trauma Acute Care Surg., 88 (2020), 186-194. |
[8] |
G. W. Fuller, R. Evans, L. Preston, H. B. Woods, S. Mason, Should adults with mild head injury who are receiving direct oral anticoagulants undergo computed tomography scanning? A systematic review, Ann. Emerg. Med., 73 (2019), 66-75. doi: 10.1016/j.annemergmed.2018.07.020
![]() |
[9] |
S. Svensson, T. Vedin, L. Clausen, P. A. Larsson, M. Edelhamre, Application of NICE or SNC guidelines may reduce the need for computerized tomographies in patients with mild traumatic brain injury: a retrospective chart review and theoretical application of five guidelines, Scand. J. Trauma, Resusc. Emerg. Med., 27 (2019), 1-10. doi: 10.1186/s13049-018-0583-1
![]() |
[10] |
J. Chen, X. Qu, Z. Li, D. Zhang, L. Hou, Peak neutrophil-to-lymphocyte ratio correlates with clinical outcomes in patients with severe traumatic brain injury, Neurocrit. Care, 30 (2019), 334-339. doi: 10.1007/s12028-018-0622-9
![]() |
Core symptoms | ADHD | ADHD-I | χ2 | ||
Number | % | Number | % | ||
Attention-deficit symptoms | |||||
Not paying attention to details | 181 | 93.3 | 82 | 92.1 | 12.64 |
Pay attention to keep it difficult | 169 | 87.1 | 82 | 92.1 | 32.33 |
Not listening | 146 | 75.3 | 62 | 69.7 | 9.45 |
Can't always follow instructions | 153 | 78.9 | 74 | 83.1 | 14.84 |
Impaired organization | 169 | 87.1 | 79 | 88.8 | 24.17 |
Unwilling to do homework | 139 | 71.6 | 64 | 71.9 | 8.52 |
Often lose things | 128 | 66 | 62 | 69.7 | 11.21 |
easily distracted | 182 | 93.8 | 84 | 94.4 | 12.15 |
Forget things often | 121 | 62.4 | 54 | 60.7 | 18.27 |
Average frequency | 79.5 ± 2.9 | 80.3 ± 2.9 | 11.23 | ||
Hyperactivity-impulsive symptoms | |||||
Many small movements | 171 | 88.1 | 69 | 77.5 | 17.87 |
Leave your seat on inappropriate occasions | 128 | 66 | 42 | 47.2 | 26.04 |
Running around in inappropriate situations | 115 | 59.3 | 32 | 36 | 37.48 |
Uproar | 65 | 33.5 | 12 | 13.5 | 30.3 |
Overactive | 110 | 56.7 | 32 | 36 | 29.06 |
Talkative | 127 | 65.5 | 36 | 40.4 | 45.85 |
Rob talk | 113 | 58.2 | 28 | 31.5 | 48.93 |
Can't wait in line | 94 | 48.5 | 18 | 20.2 | 52.87 |
Disturb others | 121 | 62.4 | 35 | 39.3 | 38.94 |
Average frequency | 59.8 ± 3.5 | 38.0 ± 3.5 | 31.91 | ||
Core symptoms | ADHD-HI | ADHD-C | P | ||
Number | % | Number | % | ||
Attention-deficit symptoms | |||||
Not paying attention to details | 11 | 73.3 | 88 | 97.8 | < 0.01 |
Pay attention to keep it difficult | 6 | 40 | 81 | 90 | < 0.01 |
Not listening | 8 | 53.3 | 76 | 84.4 | < 0.01 |
Can't always follow instructions | 6 | 40 | 73 | 81.1 | < 0.01 |
Impaired organization | 7 | 46.7 | 83 | 92.2 | < 0.01 |
Unwilling to do homework | 6 | 40 | 69 | 76.7 | < 0.05 |
Often lose things | 4 | 26.7 | 62 | 68.9 | < 0.01 |
Easily distracted | 11 | 73.3 | 87 | 96.7 | < 0.01 |
Forget things often | 2 | 13.3 | 65 | 72.2 | < 0.01 |
Average frequency | 45.2 ± 3.6 | 84.4 ± 2.6 | < 0.01 | ||
Hyperactivity-impulsive symptoms | |||||
Many small movements | 15 | 100 | 87 | 96.7 | < 0.01 |
Leave your seat on inappropriate occasions | 13 | 86.7 | 73 | 81.1 | < 0.01 |
Running around in inappropriate situations | 13 | 86.7 | 70 | 77.8 | < 0.01 |
Uproar | 9 | 60 | 44 | 48.9 | < 0.01 |
Overactive | 12 | 80 | 66 | 73.3 | < 0.01 |
talkative | 12 | 80 | 79 | 87.8 | < 0.01 |
Rob talk | 11 | 73.3 | 74 | 82.2 | < 0.01 |
Can't wait in line | 12 | 80 | 64 | 71.1 | < 0.01 |
Disturb others | 10 | 66.7 | 76 | 84.4 | < 0.01 |
Average frequency | 79.3 ± 2.9 | 78.1 ± 3.0 | < 0.01 |
Male | Female | χ2 | P | ||
ADHD-C | 90(46.4) | 75(46.3) | 15(46.9) | 0.02 | > 0.05 |
ADHD-I | 89(45.9) | 73(45.1) | 16(50.0) | 0.1 | > 0.05 |
ADHD-HI | 15(7.7) | 14(8.6) | 1(3.1) | 1.15 | > 0.05 |
Total | 194 | 162 | 32 | ||
6–8 | 9–12 | 13–16 | χ2 | P | |
ADHD-C | 31(49.2) | 51(52.6) | 8(23.5) | 8.84 | < 0.05 |
ADHD-I | 22(34.9) | 42(43.3) | 25(73.5) | 13.78 | < 0.01 |
ADHD-HI | 10(15.9) | 4(4.1) | 1(2.9) | 8.72 | < 0.05 |
Total | 63 | 97 | 34 |
Core symptoms | ADHD | ADHD-I | χ2 | ||
Number | % | Number | % | ||
Attention-deficit symptoms | |||||
Not paying attention to details | 181 | 93.3 | 82 | 92.1 | 12.64 |
Pay attention to keep it difficult | 169 | 87.1 | 82 | 92.1 | 32.33 |
Not listening | 146 | 75.3 | 62 | 69.7 | 9.45 |
Can't always follow instructions | 153 | 78.9 | 74 | 83.1 | 14.84 |
Impaired organization | 169 | 87.1 | 79 | 88.8 | 24.17 |
Unwilling to do homework | 139 | 71.6 | 64 | 71.9 | 8.52 |
Often lose things | 128 | 66 | 62 | 69.7 | 11.21 |
easily distracted | 182 | 93.8 | 84 | 94.4 | 12.15 |
Forget things often | 121 | 62.4 | 54 | 60.7 | 18.27 |
Average frequency | 79.5 ± 2.9 | 80.3 ± 2.9 | 11.23 | ||
Hyperactivity-impulsive symptoms | |||||
Many small movements | 171 | 88.1 | 69 | 77.5 | 17.87 |
Leave your seat on inappropriate occasions | 128 | 66 | 42 | 47.2 | 26.04 |
Running around in inappropriate situations | 115 | 59.3 | 32 | 36 | 37.48 |
Uproar | 65 | 33.5 | 12 | 13.5 | 30.3 |
Overactive | 110 | 56.7 | 32 | 36 | 29.06 |
Talkative | 127 | 65.5 | 36 | 40.4 | 45.85 |
Rob talk | 113 | 58.2 | 28 | 31.5 | 48.93 |
Can't wait in line | 94 | 48.5 | 18 | 20.2 | 52.87 |
Disturb others | 121 | 62.4 | 35 | 39.3 | 38.94 |
Average frequency | 59.8 ± 3.5 | 38.0 ± 3.5 | 31.91 | ||
Core symptoms | ADHD-HI | ADHD-C | P | ||
Number | % | Number | % | ||
Attention-deficit symptoms | |||||
Not paying attention to details | 11 | 73.3 | 88 | 97.8 | < 0.01 |
Pay attention to keep it difficult | 6 | 40 | 81 | 90 | < 0.01 |
Not listening | 8 | 53.3 | 76 | 84.4 | < 0.01 |
Can't always follow instructions | 6 | 40 | 73 | 81.1 | < 0.01 |
Impaired organization | 7 | 46.7 | 83 | 92.2 | < 0.01 |
Unwilling to do homework | 6 | 40 | 69 | 76.7 | < 0.05 |
Often lose things | 4 | 26.7 | 62 | 68.9 | < 0.01 |
Easily distracted | 11 | 73.3 | 87 | 96.7 | < 0.01 |
Forget things often | 2 | 13.3 | 65 | 72.2 | < 0.01 |
Average frequency | 45.2 ± 3.6 | 84.4 ± 2.6 | < 0.01 | ||
Hyperactivity-impulsive symptoms | |||||
Many small movements | 15 | 100 | 87 | 96.7 | < 0.01 |
Leave your seat on inappropriate occasions | 13 | 86.7 | 73 | 81.1 | < 0.01 |
Running around in inappropriate situations | 13 | 86.7 | 70 | 77.8 | < 0.01 |
Uproar | 9 | 60 | 44 | 48.9 | < 0.01 |
Overactive | 12 | 80 | 66 | 73.3 | < 0.01 |
talkative | 12 | 80 | 79 | 87.8 | < 0.01 |
Rob talk | 11 | 73.3 | 74 | 82.2 | < 0.01 |
Can't wait in line | 12 | 80 | 64 | 71.1 | < 0.01 |
Disturb others | 10 | 66.7 | 76 | 84.4 | < 0.01 |
Average frequency | 79.3 ± 2.9 | 78.1 ± 3.0 | < 0.01 |
Male | Female | χ2 | P | ||
ADHD-C | 90(46.4) | 75(46.3) | 15(46.9) | 0.02 | > 0.05 |
ADHD-I | 89(45.9) | 73(45.1) | 16(50.0) | 0.1 | > 0.05 |
ADHD-HI | 15(7.7) | 14(8.6) | 1(3.1) | 1.15 | > 0.05 |
Total | 194 | 162 | 32 | ||
6–8 | 9–12 | 13–16 | χ2 | P | |
ADHD-C | 31(49.2) | 51(52.6) | 8(23.5) | 8.84 | < 0.05 |
ADHD-I | 22(34.9) | 42(43.3) | 25(73.5) | 13.78 | < 0.01 |
ADHD-HI | 10(15.9) | 4(4.1) | 1(2.9) | 8.72 | < 0.05 |
Total | 63 | 97 | 34 |