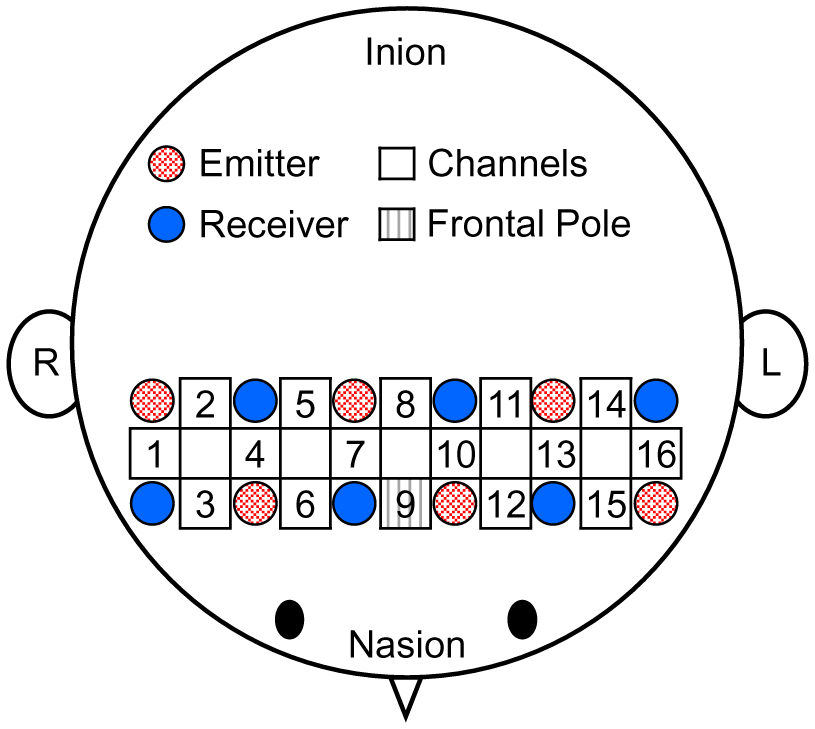
A new method of environmental control that does not depend on motor functions is eagerly awaited to support independent living for people with severe quadriplegia. In this study, we conducted an exploratory case study of brain state discrimination in a quadriplegic subject to develop a brain-computer interface controlled by a mental task execution. We measured near-infrared spectroscopy (NIRS) signals in a patient with a cervical spinal cord injury while performing mental tasks. A block design with a task and a rest separated by 30 seconds was used to measure brain function. The utilized mental tasks were mental arithmetic and Japanese word chains. Seventeen trials of the NIRS signal were acquired for each task, and 52 samples with 24-dimensional features per trial data were extracted. Random forest was used as the classifier, and the number of correct responses in the binary discrimination of the brain states were calculated by cross-validation. The exact binomial test was used for the statistical analysis, and a two-tailed test with a significance level of 5% was performed. The results showed that the number of correct responses was 15 out of 17 (p = 0.002) for the mental arithmetic task and 14 out of 17 (p = 0.013) for the Japanese word chains task, for an overall accuracy of 85%. These results indicate that this method can discriminate the brain state of a patient with quadriplegia from the NIRS signal. By applying these findings to a brain-computer interface, it will be possible to provide a new means of environmental control for individuals with quadriplegia.
Citation: Akira Masuo, Takuto Sakuma, Shohei Kato. Performance evaluation of brain state discrimination using near-infrared spectroscopy for brain-computer interface: an exploratory case study[J]. AIMS Bioengineering, 2024, 11(2): 173-184. doi: 10.3934/bioeng.2024010
[1] | Abdulmajeed Alsufyani . Performance comparison of deep learning models for MRI-based brain tumor detection. AIMS Bioengineering, 2025, 12(1): 1-21. doi: 10.3934/bioeng.2025001 |
[2] | Meher Langote, Saniya Saratkar, Praveen Kumar, Prateek Verma, Chetan Puri, Swapnil Gundewar, Palash Gourshettiwar . Human–computer interaction in healthcare: Comprehensive review. AIMS Bioengineering, 2024, 11(3): 343-390. doi: 10.3934/bioeng.2024018 |
[3] | Jacinthe Gagnon, Rachel A. Caruso, Katharina M. Fromm . Embedding CeO2 nanocontainers in a TiO2 coating on glass surfaces. AIMS Bioengineering, 2017, 4(1): 171-178. doi: 10.3934/bioeng.2017.1.171 |
[4] | Thi Kim Thoa Thieu, Roderick Melnik . Coupled effects of channels and synaptic dynamics in stochastic modelling of healthy and Parkinson's-disease-affected brains. AIMS Bioengineering, 2022, 9(2): 213-238. doi: 10.3934/bioeng.2022015 |
[5] | Lindsey M. Kline, Nicole Labbé, Christopher Boyer, T. Edward Yu, Burton C. English, James A. Larson . Investigating the impact of biomass quality on near-infrared models for switchgrass feedstocks. AIMS Bioengineering, 2016, 3(1): 1-22. doi: 10.3934/bioeng.2016.1.1 |
[6] | Marianthi Logotheti, Eleftherios Pilalis, Nikolaos Venizelos, Fragiskos Kolisis, Aristotelis Chatziioannou . Development and validation of a skin fibroblast biomarker profile for schizophrenic patients. AIMS Bioengineering, 2016, 3(4): 552-565. doi: 10.3934/bioeng.2016.4.552 |
[7] | N. Supraja, T.N.V.K.V. Prasad, M. Soundariya, R. Babujanarthanam . Synthesis, characterization and dose dependent antimicrobial and anti-cancerous activity of phycogenic silver nanoparticles against human hepatic carcinoma (HepG2) cell line. AIMS Bioengineering, 2016, 3(4): 425-440. doi: 10.3934/bioeng.2016.4.425 |
[8] | Moawiah M Naffaa . Innovative therapeutic strategies for traumatic brain injury: integrating regenerative medicine, biomaterials, and neuroengineering. AIMS Bioengineering, 2025, 12(1): 90-144. doi: 10.3934/bioeng.2025005 |
[9] | Carlos Marcuello . Current and future perspectives of atomic force microscopy to elicit the intrinsic properties of soft matter at the single molecule level. AIMS Bioengineering, 2022, 9(3): 293-306. doi: 10.3934/bioeng.2022020 |
[10] | Baraa Chasib Mezher AL Kasar, Shahab Khameneh Asl, Hamed Asgharzadeh, Seyed Jamaleddin Peighambardoust . Denoising deep brain stimulation pacemaker signals with novel polymer-based nanocomposites: Porous biomaterials for sound absorption. AIMS Bioengineering, 2024, 11(2): 241-265. doi: 10.3934/bioeng.2024013 |
A new method of environmental control that does not depend on motor functions is eagerly awaited to support independent living for people with severe quadriplegia. In this study, we conducted an exploratory case study of brain state discrimination in a quadriplegic subject to develop a brain-computer interface controlled by a mental task execution. We measured near-infrared spectroscopy (NIRS) signals in a patient with a cervical spinal cord injury while performing mental tasks. A block design with a task and a rest separated by 30 seconds was used to measure brain function. The utilized mental tasks were mental arithmetic and Japanese word chains. Seventeen trials of the NIRS signal were acquired for each task, and 52 samples with 24-dimensional features per trial data were extracted. Random forest was used as the classifier, and the number of correct responses in the binary discrimination of the brain states were calculated by cross-validation. The exact binomial test was used for the statistical analysis, and a two-tailed test with a significance level of 5% was performed. The results showed that the number of correct responses was 15 out of 17 (p = 0.002) for the mental arithmetic task and 14 out of 17 (p = 0.013) for the Japanese word chains task, for an overall accuracy of 85%. These results indicate that this method can discriminate the brain state of a patient with quadriplegia from the NIRS signal. By applying these findings to a brain-computer interface, it will be possible to provide a new means of environmental control for individuals with quadriplegia.
Spinal cord injuries (SCI) can occur because of compulsion, incision, or contusion [1], and causes severe disabilities, including either temporary or permanent motor, sensory, bladder, and bowel dysfunctions. Particularly in cervical spinal cord injuries (CSCI), which pertain to injuries of the cervical spine, profound and widespread motor paralysis can affect the limbs and trunk, which poses severe challenges in activities of daily living. For instance, paralysis of the upper limbs greatly hinders tasks such as operating a television or an assistive device. Consequently, despite the preservation of cognitive function in patients with CSCI, compromised motor function necessitates the need of caregiver assistance for a comprehensive environmental control. Therefore, occupational therapy for spinal cord injuries supports functional rehabilitation to improve motor function [2], the acquisition of compensatory measures by operating assistive devices with residual function [3], and improve independence. However, motor dysfunction caused by a CSCI is intractable, and residual motor function declines with age. Therefore, current occupational therapies using residual motor function have limitations, and there is a need to establish new methods to support environmental control by utilizing other functions.
Brain-computer interfaces (BCIs) [4] have attracted attention in recent years as a technology to realize device control using physiological signals obtained by measuring brain functions. Typically, a BCI consists of a brain function measurement section, a preprocessing section, a feature selection and extraction section, a discrimination section, and a device control section [5]. In the brain function measurement section, physiological signals related to the brain state during cognitive tasks are measured, and unwanted components such as noise and artifacts are removed through preprocessing. Features related to brain information are selected and extracted from the physiological signals, and a discriminative model of the brain state is constructed using machine learning methods. Based on this model, the newly acquired signals are used to identify the brain state, thereby realizing device control using physiological signals. A BCI can provide a novel method of environmental control for patients with SCI's and is desirable for medical applications.
Most BCI studies have used electroencephalography [6],[7]. In contrast, this study focused on near-infrared spectroscopy (NIRS) [8] to measure brain function. NIRS is based on the modified Beer-Lambert law [9], which describes the relationship between light attenuation and the concentration of light-absorbing substances when a scattering medium containing light-absorbing substances is exposed to light. NIRS is used for the noninvasive measurement of changes in the hemoglobin-oxygen metabolism of active brain regions using near-infrared light in the 700–1000 nm wavelength range, which has a high biological transparency. NIRS can be measured in a natural environment because it is portable, compact, and resistant to electrical noise [5]. In addition, NIRS has recently been examined for its application as a therapeutic tool in rehabilitation owing to the development of signal processing technologies [10]. Therefore, NIRS helps measure brain function in patients with neurological diseases prone to body movement noise, such as spasticity.
In a study of a BCI using NIRS, Hwang et al. investigated mental tasks suitable do discriminate various brain states in seven healthy participants. They reported the effectiveness of multiplication, right-hand motor recall, and figure-rotation tasks [11]. Naseer et al. reported that they could discriminate the brain states of 14 healthy participants during mental arithmetic and resting tasks, which achieved an accuracy of 82.1% [12]. In a study using motor-related tasks, Sitaram et al. reported an accuracy of 89.1% using left and right finger-tapping recall tasks in five healthy participants [13]. Furthermore, Hamid et al. reported an accuracy of 88.5% when discriminating brain states during walking and resting-standing tasks in nine healthy participants [14]. However, these reports were laboratory-based studies that involved healthy participants, and their applicability to patients with quadriplegia was not validated. Schreuder et al. [15] have emphasized the importance of targeting potential end-users. Moreover, it has been suggested that performing motor imagery tasks could be difficult for patients with long-term motor impairments [16]. Therefore, for the practical application of a BCI, it is necessary to construct a brain state discrimination technique for a patient with motor dysfunction using mental tasks that consider the disability characteristics of the end-user. The novelty of this study is that we verified the performance of machine learning to discriminate brain states using non-motor-related mental tasks in a case study of a patient with quadriplegia.
Therefore, this study aimed to develop a brain-state discrimination method for a BCI application for the environmental control by physically disabled individuals. NIRS signals were measured in a patient with a CSCI while performing two types of mental tasks, and a binary discrimination model was constructed through an offline analysis using machine learning. The discriminant performance of the constructed model was evaluated, and its applicability to a BCI system that could assist physically disabled people to control their environments was examined.
The participant was a man in his 60s who was diagnosed with a CSCI at the fifth cervical level, and whose dominant hand was determined to be the right hand based on the Edinburgh Handedness Test. The participant developed quadriplegia because of a sports injury 25 years ago, and currently lives at home with home care services. The severity of the injury was B2 according to the modified Frankel classification [17], with complete paralysis of the limbs and trunk muscles below the injury site. The participant could partially enter text on a computer using compensatory movements with shoulder girdle elevation and voice control, though he required total assistance with environmental control, such as manipulating switches for lights and motorized beds. The functional independence measure scores for his activities of daily living were 13 for motor items and 35 for cognitive items. In contrast, the participant required total assistance with all physical activities, but was independent in conversational communication and had no intellectual functioning problems.
This study was conducted following the ethical principles of the Helsinki Declaration and was reviewed and approved by the Ethics Committee of the Nagoya Institute of Technology (Approval No. 2019–010); the consent of the participant was obtained.
The OEG-SpO2 (Spectratech Inc., Yokohama, Japan) was used to measure the NIRS signals. Figure 1 presents an overview of the equipment used in this study. The prefrontal region was set as the region of interest, and the head module was mounted such that nine channels were positioned directly above the center of the frontal poles (Fp1 and Fp2) following the international 10–20 system [18]. NIRS signals were measured at a distance of 3 cm between the light emitter and receiver, and a sampling frequency of 12.4 Hz for 16 channels was utilized. Oxygenated hemoglobin (HbO) is the most sensitive indicator of changes in regional cerebral blood flow [19] and is appropriate to detect neural activity in different brain states. Therefore, we used HbO as an indicator in this study.
In this study, considering the disability characteristics of the CSCI, we used mental tasks unrelated to motor function. The participant was instructed to determine the tasks they could execute from those used in a previous study [20]; mental arithmetic (MA) and Japanese word chain (JWC) tasks were selected. Before the NIRS signal measurement, each task was practiced and the participant was required to verbally respond to confirm that they had sufficient cognitive function to execute the task. The definitions of each task are as follows.
MA: A task in which single-digit numbers (e.g., 7) were sequentially added by mental arithmetic (e.g., 7: 7, 14, 21, 28, ...). These numbers were randomly chosen.
JWC: This task is called Shiritori in Japanese, which repeats a Japanese word chain as quickly as possible (e.g., Ringo, Gorilla, Rappa, ...). In this task, words ending in “n” were not selected.
In this study, two subjective assessments were conducted to evaluate the mental effort required by the participant to execute a mental task, that is, to control the BCI.
State anxiety items from the STAI-Form JYZ were used to assess the psychological burden transiently perceived during the execution of the mental task. The state anxiety items were scored on a four-point scale of “not at all true,” “somewhat true,” “fairly true,” and “very true” on 20 questions related to worry, nervousness, tension, and anxiety. A higher total score indicated a higher level of anxiety, and the state anxiety was rated on a five-point scale according to the score.
Eight items related to the usability of the mental tasks were defined according to previous studies [22],[23]: flexibility, ease of performance, practicality, ease of understanding, difficulty, discomfort, concentration, and drowsiness. The subjective rating for each task was assessed using a visual analogue scale. Each item was numerically scored on a 100-point scale, with a high score indicating a high usability. The scores for each item and the overall average were calculated.
The experiment was conducted while the participant was seated in a wheelchair in the living room. A block design of three trials was used for the experiment, with each trial consisting of 30 seconds of rest and 30 seconds for the task. Figures 2 and 3 show the experimental environment and block design, respectively. During the rest period, the participant was instructed to cease cognitive activity and remain relaxed, whereas during the task period, he was instructed to perform the MA or JWC task that were provided beforehand. The participant performed the mental task according to the instructions presented on the display. The participant was instructed to maintain a fixed gaze point throughout the experiment to minimize the influence of eye movement noise on the NIRS signal. The order in which the mental tasks were executed was randomized in each block design to minimize the effects of habituation. Considering the influence of mental fatigue on the task execution, a break of a few minutes was allowed between each brain function measurement. The NIRS signal was measured over two days, with 18 trials per mental task for a total of 36 minutes. Subjective assessments were conducted after the NIRS measurements on the first day.
Data from one trial containing artifacts due to body motion noise and data from channels with missing values were excluded from preprocessing the acquired NIRS signals. Thus, the HbO from 14 and 16 channels for 17 trials and their derivative values, namely ΔHbO, were included in the analysis. The data were standardized using each trial's mean and standard deviation of the 5-s pre-task rest period.
For the feature extraction, we segmented the task interval data with a time window of 5 s and a step width of 0.5 s, from which we extracted six time-domain features: maximum, minimum, arithmetic mean, standard deviation, kurtosis, and skewness. Thus, 52 samples that were comprised of 24 dimensions per trial were extracted. The dataset was constructed by assigning a type of mental task to a label.
This dataset was used to train the discriminant model. We used Random Forest [24], which is an ensemble learning algorithm with multiple decision trees, as weak learners. Three types of discriminant models were constructed in this study.
(1) Model_ALL: A model trained using all features.
(2) Model_Boruta: A model trained with only features contributing to discrimination using Boruta [25], which is a feature selection method based on a binomial test.
(3) Model_UMAP: A model trained using data reduced to two dimensions using a Uniform Manifold Approximation and Projection (UMAP) [26], which is a nonlinear dimensionality reduction method.
The Random Forest was tuned by a grid search using two hyperparameters: sqrt and log2 for max_features and 5, 10, 15, ..., 50 for max_depth. The model was trained, and its performance was evaluated by k-fold cross-validation, where k was 17, which was the number of trials used to measure the brain function in each mental task.
The number of correct responses was used as a measure of the model performance. The number of correct responses was defined as the number of true positives or true negatives of the predictions obtained by majority voting on the predictions from the 52 samples. A statistical analysis of the discriminant model performance was conducted using an exact binomial test and a two-tailed test with a significance level of 5%.
Figure 4 shows the standardized waveform of the NIRS signal obtained by averaging data from all 17 trials. Bandpass filtering at 0.01–0.2 Hz was applied for visualization. The 14-channel signal exhibited a fluctuation pattern in which the MA increased more than the JWC did throughout the task interval. In contrast, the 16-channel JWC task revealed a fluctuation pattern that decreased in the early part of the task interval and subsequently increased slightly compared with MA.
Figure 5 shows the confusion matrix of the model predictions. Model_ALL and model_UMAP had the highest total number of true positives and true negatives, indicating excellent predictive results. Table 1 lists the discriminant performance of the models. The number of correct responses was 29 out of 34 trials for both the model_ALL and model_UMAP and 27 out of 34 trials for the model_Boruta, both of which were significantly different (p < .001). Regarding the accuracy of each class, the model_ALL exhibited high performances in the MA and JWC classes, with 88.2% and 82.4%, respectively.
Task | Model_ALL | Model_Boruta | Model_UMAP |
Total (N = 34) | 29***(85.3%) | 27***(79.4%) | 29***(85.3%) |
MA (n = 17) | 15 (88.2%) | 13 (76.5%) | 17 (100%) |
JWC (n = 17) | 14 (82.4%) | 14 (82.4%) | 12 (70.6%) |
Data are presented as numbers (percent). *** An exact binomial test, p < .001.
Figures 6 and 7 display the results of the STAI and the usability evaluation, respectively. On the STAI, MA scored 40, stage 2, which indicates a low anxiety. JWC scored 42, stage 3, which indicates a normal state. Regarding the usability evaluation, the average scores were 78.0 for MA and 75.9 for JWC, although JWC had a low score of 11 for the item of difficulty.
In this study, we performed brain state discrimination in a CSCI patient using NIRS signals and achieved a discrimination accuracy of 85.3%. Consequently, by applying this knowledge to a BCI, for example, associating “MA” with “ON” and “JWC” with “OFF” and operating the BCI using mental tasks as operating commands, environmental control such as lighting, air conditioning, and welfare equipment can be achieved.
In a binary BCI, the upper confidence interval for the discrimination performance obtained by chance has been reported to be 70% [27], and an accuracy of 70% is considered to be a practical criterion for a BCI. Previous studies have reported discrimination accuracies of 82–89% [12]–[14]. However, these studies had the disadvantage of laboratory-based results from healthy subjects. In addition, it has been indicated that motor tasks and motor imagery tasks may not be feasible for patients with quadriplegia, and their applicability to end-users has not been verified. In contrast, the proposed method has the advantage that it was examined using non-motor-related tasks on a patient with a CSCI to verify its applicability to a BCI. Moreover, the discrimination performance of the proposed method was comparable to previous studies, thus indicating the effectiveness of our method as a means of environmental control for patients with motor dysfunction. In addition, innovative rehabilitation techniques using robots have recently received considerable attention. Caliandro et al. [28] reported that a wearable powered exoskeleton enhanced prefrontal cortex activation. The brain state identification model in this study could be applied to the autonomous robotic control of patients who require robotic assistance. In the field of occupational therapy, there is no practical environmental control system that uses brain functions to support the daily living of people with physical disabilities, and these findings can contribute to the establishment of a new occupational therapy approach for patients with quadriplegia.
The psychological burden and usability of executing mental tasks for a BCI control were evaluated and showed an acceptable level for the participant. Nakai et al. proposed a BCI that used a meal experience recall task [29], but the practicality of the recall task was not mentioned. Weyand et al. reported that the discrimination performance and usability are necessary for a BCI [30]. Therefore, in contrast to previous studies that only examined the discrimination performance [12]–[14],[29], this study also investigated the BCI performance from the perspective of a subjective evaluation and obtained practical-level results for both the discrimination performance and the usability. Considering that opportunities for environmental control frequently occur in daily life (e.g., turning lights and welfare equipment on and off), mental tasks used for a BCI control should be easy to perform with little burden. Moreover, it is expected that these mental tasks would be equally accessible to perform, given that the end-users of the BCIs (i.e., patients with motor dysfunction) have normal brain function. Thus, using MA and JWC will contribute to developing environmentally controlled BCIs for patients with motor dysfunction. This study did not have a sufficient practice period to improve the task performance. Therefore, the psychological burden and ease of use could be further improved by enhancing recall skills and familiarity through mental task execution training. In the future, we plan to examine the effects of task execution practice for skill acquisition on the BCI's discrimination performance and usability.
As a limitation, this study was conducted in a controlled environment, and we did not consider the potential fluctuations of NIRS signals that may occur with aging, psychological state, or environmental factors. In addition, it is essential to note that this study presents a single-case analysis, limiting the generalizability of our findings to a broader population. In the future, we plan to address these limitations by constructing a robust model that utilizes a large dataset collected over an extended period, thereby allowing us to assess the BCI's performance during long-term utilization. In addition, we plan to conduct comparative experiments with healthy subjects to examine the effectiveness and versatility of this model. Additionally, we plan to explore alternative BCI designs by incorporating various mental tasks. Then, we plan to increase the number of participants to investigate the generalizability of this method and apply it to a BCI system of environmental control for patients with CSCI's.
We constructed a model to discriminate brain states from NIRS signals and evaluated its performance to be applied to a novel environmental control system for people with quadriplegia. Consequently, the brain-state discrimination of MA and JWC fulfilled the practical level of the BCI and had a high usability. This method applies to BCI's for environmental control to support the daily lives of patients with severe quadriplegia. The findings of this study would contribute to the establishment of a novel augmentative and alternative communication using the residual functions of people with quadriplegia in occupational therapies.
[1] | Nas K, Yazmalar L, Şah V, et al. (2015) Rehabilitation of spinal cord injuries. World J Orthop 6: 8-16. https://doi.org/10.5312/wjo.v6.i1.8 |
[2] | Zbogar D, Eng JJ, Miller WC, et al. (2017) Movement repetitions in physical and occupational therapy during spinal cord injury rehabilitation. Spinal Cord 55: 172-179. https://doi.org/10.1038/sc.2016.129 |
[3] | Yao DPG, Inoue K, Sy MP, et al. (2020) Experience of Filipinos with spinal cord injury in the use of assistive technology: An occupational justice perspective. Occup Ther Int 1–10. https://doi.org/10.1155/2020/6696296 |
[4] | Wolpaw JR, Birbaumer N, Heetderks WJ, et al. (2000) Brain-computer interface technology: A review of the first international meeting. IEEE Trans Rehabil Eng 8: 164-173. https://doi.org/10.1109/tre.2000.847807 |
[5] | Naseer N, Hong KS (2015) fNIRS-based brain-computer interfaces: A review. Front Hum Neurosci 9: 3. https://doi.org/10.3389/fnhum.2015.00003 |
[6] | Farwell LA, Donchin E (1988) Talking off the top of your head: Toward a mental prosthesis utilizing event-related brain potentials. Electroencephalogr Clin Neurophysiol 70: 510-523. https://doi.org/10.1016/0013-4694(88)90149-6 |
[7] | Said RR, Heyat MB, Song K, et al. (2022) A systematic review of virtual reality and robot therapy as recent rehabilitation technologies using EEG-brain-computer interface based on movement-related cortical potentials. Biosensors 12: 1134. https://doi.org/10.3390/bios12121134 |
[8] | Jöbsis FF (1977) Noninvasive, infrared monitoring of cerebral and myocardial oxygen sufficiency and circulatory parameters. Science 198: 1264-1267. https://doi.org/10.1126/science.929199 |
[9] | Delpy DT, Cope M, van der Zee P, et al. (1988) Estimation of optical pathlength through tissue from direct time of flight measurement. Phys Med Biol 33: 1433-1442. https://doi.org/10.1088/0031-9155/33/12/008 |
[10] | Mihara M, Miyai I (2016) Review of functional near-infrared spectroscopy in neurorehabilitation. Neurophotonics 3: 031414. https://doi.org/10.1117/1.NPh.3.3.031414 |
[11] | Hwang HJ, Lim JH, Kim DW, et al. (2014) Evaluation of various mental task combinations for near-infrared spectroscopy-based brain-computer interfaces. J Biomed Opt 19: 77005. https://doi.org/10.1117/1.JBO.19.7.077005 |
[12] | Naseer N, Hong MJ, Hong KS (2014) Online binary decision decoding using functional near-infrared spectroscopy for the development of brain-computer interface. Exp Brain Res 232: 555-564. https://doi.org/10.1007/s00221-013-3764-1 |
[13] | Sitaram R, Zhang H, Guan C, et al. (2007) Temporal classification of multichannel near-infrared spectroscopy signals of motor imagery for developing a brain-computer interface. Neuroimage 34: 1416-1427. https://doi.org/10.1016/j.neuroimage.2006.11.005 |
[14] | Hamid H, Naseer N, Nazeer H, et al. (2022) Analyzing classification performance of fNIRS-BCI for gait rehabilitation using deep neural networks. Sensors 22: 1932. https://doi.org/10.3390/s22051932 |
[15] | Schreuder M, Riccio A, Risetti M, et al. (2013) User-centered design in brain-computer interfaces-a case study. Artif Intell Med 59: 71-80. https://doi.org/10.1016/j.artmed.2013.07.005 |
[16] | Kasahara T, Terasaki K, Ogawa Y, et al. (2012) The correlation between motor impairments and event-related desynchronization during motor imagery in ALS patients. BMC Neurosci 13: 66. https://doi.org/10.1186/1471-2202-13-66 |
[17] | Bradford DS, McBride GG (1987) Surgical management of thoracolumbar spine fractures with incomplete neurologic deficits. Clin Orthop Relat Res 218: 201-216. https://journals.lww.com/clinorthop/abstract/1987/05000 |
[18] | Jasper H (1958) Report of the committee on methods of clinical examination in electroencephalography: 1957. Electroencephalogr Clin Neurophysiol 10: 370-375. https://doi.org/10.1016/0013-4694(58)90053-1 |
[19] | Hoshi Y, Kobayashi N, Tamura M (2001) Interpretation of near-infrared spectroscopy signals: A study with a newly developed perfused rat brain model. J Appl Physiol 90: 1657-1662. https://doi.org/10.1152/jappl.2001.90.5.1657 |
[20] | Masuo A, Sakuma T, Kato S (2023) Discriminating brain activation state in a patient with Duchenne muscular dystrophy using near-infrared spectroscopy for communication: An exploratory case study. Asian J Occup Ther 19: 1-8. https://doi.org/10.11596/asiajot.19.55 |
[21] | Hidano N, Fukuhara M, Iwawaki S, et al. (2000) Manual for the State-trait Anxiety Inventory-Form JYZ (in Japanese). Tokyo: Jitsumu Kyoiku-Shuppan 23-29. |
[22] | Scherer R, Müller-Putz GR, Pfurtscheller G (2009) Flexibility and practicality graz brain-computer interface approach. Int Rev Neurobiol 86: 119-131. https://doi.org/10.1016/S0074-7742(09)86009-1 |
[23] | Coyle S, Ward T, Markham C, et al. (2004) On the suitability of near-infrared (NIR) systems for next-generation brain-computer interfaces. Physiol Meas 25: 815-822. https://doi.org/10.1088/0967-3334/25/4/003 |
[24] | Breiman L (2001) Random forests. Mach Learn 45: 5-32. https://doi.org/10.1023/A:1010933404324 |
[25] | Kursa M, Rudnicki W (2010) Feature selection with the boruta package. J Stat Softw 36: 1-13. https://doi.org/10.18637/jss.v036.i11 |
[26] | Becht E, McInnes L, Healy J, et al. (2018) Dimensionality reduction for visualizing single-cell data using UMAP. Nat Biotechnol 37: 38-44. https://doi.org/10.1038/nbt.4314 |
[27] | Müller-Putz G, Scherer R, Brunner C, et al. (2008) Better than random? A closer look on BCI results. Int J Bioelectromagn 10: 52-55. https://www.ijbem.org |
[28] | Caliandro P, Molteni F, Simbolotti C, et al. (2020) Exoskeleton-assisted gait in chronic stroke: An EMG and functional near-infrared spectroscopy study of muscle activation patterns and prefrontal cortex activity. Clin Neurophysiol 131: 1775-1781. https://doi.org/10.1016/j.clinph.2020.04.158 |
[29] | Nakai Y, Nakamura M, Tomida M, et al. (2022) Brain-computer interface using fNIRS waveforms when recalling the experience of eating savory and spicy instant noodle. J Adv Inf Technol 13: 381-386. https://doi.org/10.12720/jait.13.4.381-386 |
[30] | Weyand S, Schudlo L, Takehara-Nishiuchi K, et al. (2015) Usability and performance-informed selection of personalized mental tasks for an online near-infrared spectroscopy brain-computer interface. Neurophotonics 2: 025001. https://doi.org/10.1117/1.NPh.2.2.025001 |
Task | Model_ALL | Model_Boruta | Model_UMAP |
Total (N = 34) | 29***(85.3%) | 27***(79.4%) | 29***(85.3%) |
MA (n = 17) | 15 (88.2%) | 13 (76.5%) | 17 (100%) |
JWC (n = 17) | 14 (82.4%) | 14 (82.4%) | 12 (70.6%) |
Data are presented as numbers (percent). *** An exact binomial test, p < .001.