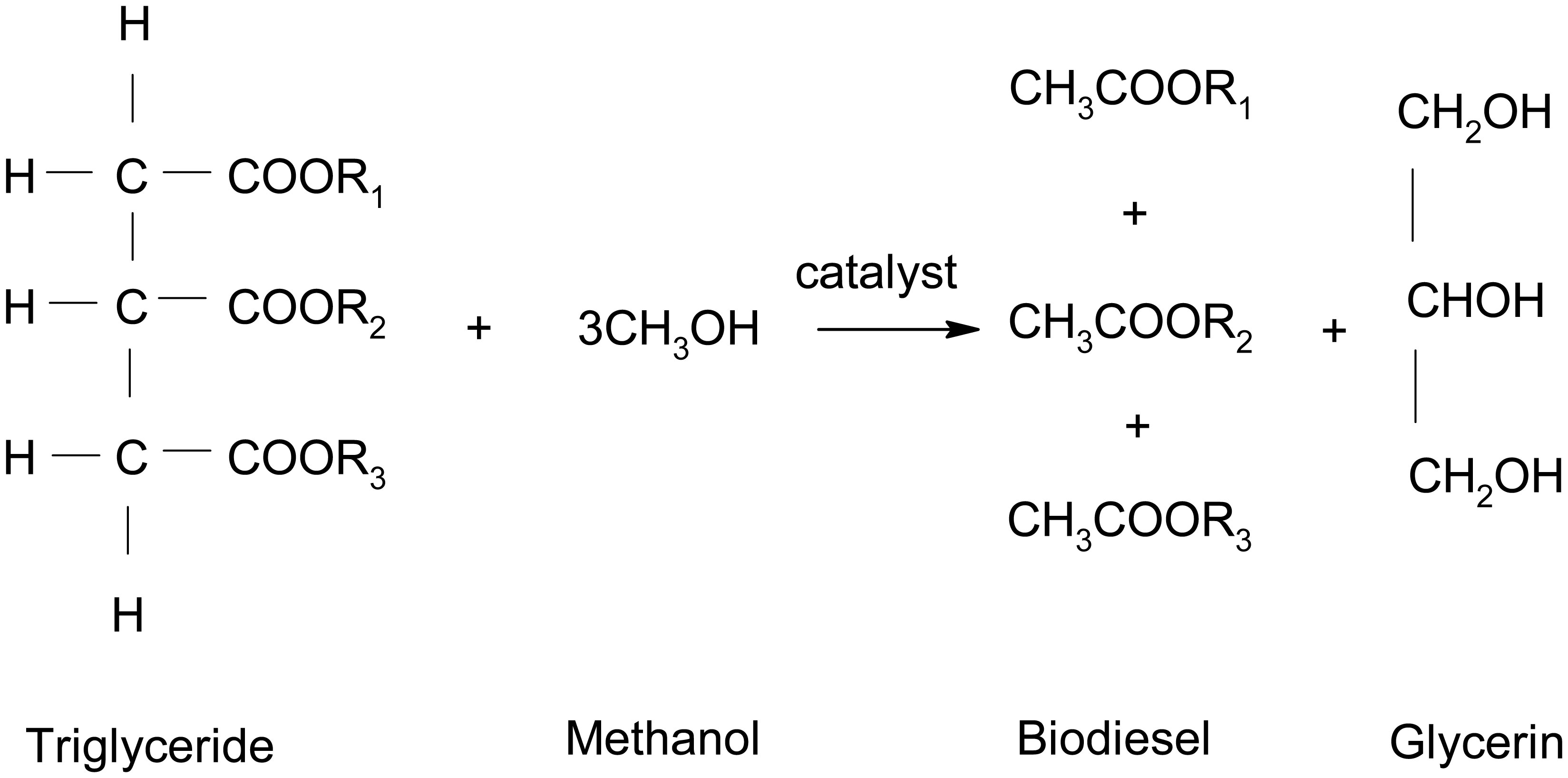
In this research work, various machine learning models such as linear regression (LR), KNN and MLP were created to predict the optimized synthesis of biodiesel from pre-treated and non-treated Linseed oil in base transesterification reaction mode. Three input parameters were included for modelling, reaction time, catalyst concentrated ion, and methanol/oil-molar ratio. In biodiesel transesterification reaction 180 samples run with non-Pre-treated Linseed Methyl Ester (NPLME), Water Pre-treated Linseed Methyl Ester (WPLME) and Enzymatic Pre-treated Linseed Methyl Ester (EPLME) oil as feed stocks and optimized parameters are find out for maximum biodiesel yield to be 8:1 molar ratio, 0.4% weight catalyst, 60 °C reaction temperature.To test the technique, R2 and MAPE parameters were used. The average R2 values for linear regression, KNN, and MLP are 0.7030, 0.8554 and 0.7864 respectively. Moreover, the average MAPE values for these models are 11.1886, 6.0873 and 8.0669 respectively. Hence, it is observed that the KNN model outperforms other models with higher accuracy and low MAPE score.
Citation: Sunil Gautam, Sangeeta Kanakraj, Azriel Henry. Computational approach using machine learning modelling for optimization of transesterification process for linseed biodiesel production[J]. AIMS Bioengineering, 2022, 9(4): 319-336. doi: 10.3934/bioeng.2022023
[1] | Islam Uddin, Salman A. AlQahtani, Sumaiya Noor, Salman Khan . Deep-m6Am: a deep learning model for identifying N6, 2′-O-Dimethyladenosine (m6Am) sites using hybrid features. AIMS Bioengineering, 2025, 12(1): 145-161. doi: 10.3934/bioeng.2025006 |
[2] | Shital Hajare, Rajendra Rewatkar, K.T.V. Reddy . Design of an iterative method for enhanced early prediction of acute coronary syndrome using XAI analysis. AIMS Bioengineering, 2024, 11(3): 301-322. doi: 10.3934/bioeng.2024016 |
[3] | Kayode Oshinubi, Augustina Amakor, Olumuyiwa James Peter, Mustapha Rachdi, Jacques Demongeot . Approach to COVID-19 time series data using deep learning and spectral analysis methods. AIMS Bioengineering, 2022, 9(1): 1-21. doi: 10.3934/bioeng.2022001 |
[4] | Sumaiya Noor, Salman A. AlQahtani, Salman Khan . Chronic liver disease detection using ranking and projection-based feature optimization with deep learning. AIMS Bioengineering, 2025, 12(1): 50-68. doi: 10.3934/bioeng.2025003 |
[5] | Marianthi Logotheti, Eleftherios Pilalis, Nikolaos Venizelos, Fragiskos Kolisis, Aristotelis Chatziioannou . Development and validation of a skin fibroblast biomarker profile for schizophrenic patients. AIMS Bioengineering, 2016, 3(4): 552-565. doi: 10.3934/bioeng.2016.4.552 |
[6] | Rabia Aziz, C.K. Verma, Namita Srivastava . Dimension reduction methods for microarray data: a review. AIMS Bioengineering, 2017, 4(1): 179-197. doi: 10.3934/bioeng.2017.1.179 |
[7] | Urooj Ainuddin, Maria Waqas . Finite state machine and Markovian equivalents of the lac Operon in E. coli bacterium. AIMS Bioengineering, 2022, 9(4): 400-419. doi: 10.3934/bioeng.2022029 |
[8] | Kuna Dhananjay Rao, Mudunuru Satya Dev Kumar, Paidi Pavani, Darapureddy Akshitha, Kagitha Nagamaleswara Rao, Hafiz Tayyab Rauf, Mohamed Sharaf . Cardiovascular disease prediction using hyperparameters-tuned LSTM considering COVID-19 with experimental validation. AIMS Bioengineering, 2023, 10(3): 265-282. doi: 10.3934/bioeng.2023017 |
[9] | Xiao-Li Gu, Li Yu, Yu Du, Xiu-Peng Yang, Yong-Gang Xu . Identification and validation of aging-related genes and their classification models based on myelodysplastic syndromes. AIMS Bioengineering, 2023, 10(4): 440-465. doi: 10.3934/bioeng.2023026 |
[10] | Yi Deng, Zhiguo Wang, Xiaohui Li, Yu Lei, Owen Omalley . Advancing biomedical engineering through a multi-modal sensor fusion system for enhanced physical training. AIMS Bioengineering, 2023, 10(4): 364-383. doi: 10.3934/bioeng.2023022 |
In this research work, various machine learning models such as linear regression (LR), KNN and MLP were created to predict the optimized synthesis of biodiesel from pre-treated and non-treated Linseed oil in base transesterification reaction mode. Three input parameters were included for modelling, reaction time, catalyst concentrated ion, and methanol/oil-molar ratio. In biodiesel transesterification reaction 180 samples run with non-Pre-treated Linseed Methyl Ester (NPLME), Water Pre-treated Linseed Methyl Ester (WPLME) and Enzymatic Pre-treated Linseed Methyl Ester (EPLME) oil as feed stocks and optimized parameters are find out for maximum biodiesel yield to be 8:1 molar ratio, 0.4% weight catalyst, 60 °C reaction temperature.To test the technique, R2 and MAPE parameters were used. The average R2 values for linear regression, KNN, and MLP are 0.7030, 0.8554 and 0.7864 respectively. Moreover, the average MAPE values for these models are 11.1886, 6.0873 and 8.0669 respectively. Hence, it is observed that the KNN model outperforms other models with higher accuracy and low MAPE score.
The energy crisis has aided the growth of biodiesel manufacturing. Charcoal, petroleum, and natural gas are finite resources that will be depleted by the end of the century. As a result, finding alternate energy sources is critical. Vegetable oils have a similar energetic content as diesel fuel and are a renewable, theoretically limitless source of energy. Since its introduction as an alternative fuel for diesel engines, bio-diesel has gained popularity due to its advantages for the environment and its production from renewable resources. Since edible oil consumption exceeds local production, India buys crude oil and petroleum products from Gulf nations in addition to having a large number of trees in its forests that produce a variety of oilseeds. In order to save the environment and withstand economic downturns, researchers searched for a diesel fuel substitute.
Bio-based fuels like vegetable oil have caught the attention of the scientific community due to fuel shortages and environmental issues. Bio-diesel is the ideal alternative fuel since it is technically sound, environmentally friendly, and readily available.Io-diesel, a fuel that can be utilised in diesel engines, is made up of B fatty acid methyl/ethyl esters [1]. It is a renewable diesel fuel made by transesterifying monohydric alcohols with renewable biological sources like vegetable oils and animal fats [2]–[4]. Biodiesel is any diesel-equivalent biofuel that can be used in any diesel engine vehicle that hasn't been modified [5]. Around the world, a variety of feedstock oils, including soybean, rapeseed, sunflower, safflower, and others, are used to produce biodiesel. These oils are primarily edible in nature [6]–[9].
Non-edible oils such as Karanj, Mahua, Rice bran, Jatropha, and Linseed oil have been used to make bio-diesel, mostly in India [10]. Producing renewable energy from vegetable oils like linseed and jatropha will help India and other developing nations meet their energy goals [11]. Our country's climate and soil conditions are ideal for growing the Linseed (Linumusitatissimum) crop [12]. The main Linseed oil production location is in village [13]. Linseed seed contains roughly 40% oleaginous (oil) content, which can be turned to biodiesel [14].
Sirajuddin et al. organic heterogeneous catalysts called organotin (IV) carboxylates to make biodiesel from corn oil. 83% with the assistance of the created catalysts, biodiesel production from corn oil was accomplished. In light of this, one of the best catalysts for the production of biodiesel can be used in the future with the newly created organotin (IV) carboxylate complexes [15].
Tariq et al. presents the FTIR, XRD, and SEM characterization of cadmium oxide in the nano range that is produced by a hydrothermal technique. This cadmium oxide is then used as a catalyst to prepare biodiesel by transesterifying linseed oil. Utilizing FTIR and 1HNMR spectroscopy, the produced biodiesel was evaluated. Linseed oil biodiesel's fatty acid makeup was investigated using GC-MS analysis [16].
Tariq et al. describe the hydrothermal procedure used to produce nanoscale cadmium oxide, which was then studied by FTIR, XRD, and SEM. This cadmium oxide was then used as a catalyst to prepare biodiesel by transesterifying linseed oil. FTIR and HNMR spectroscopy were used to evaluate the biodiesel that had been created. To investigate the fatty acid composition of linseed oil biodiesel, GC-MS analysis was conducted [17].
Tariq et al. creating new biologically active chemicals, six 3-(4-flourophenyl)-2-methylacrylic acid organotin (IV) carboxylate complexes were created. FT-IR, NMR (1H and 13C), and X-ray crystallography were used to describe the complexes. With SSDNA, UV-visible spectroscopy and viscosity measurements were used to examine the interactions between the ligand HL and complexes 1 and 4 [18].
Tariq et al. Developedsynthesise di- and tri-organotin (IV) carboxylates of the form R4nSnLn, where R is Me, n-Bu, or Ph, L is 2-(4-ethylbenzylidene) but anoic acid, and n is 1 or 2. Elemental analysis, FT-IR, NMR (1H, 13C, and 119Sn), and single crystal X-ray analysis were used to describe these compounds. The antibacterial, antifungal, and cytotoxic properties of the produced compounds were evaluated [19].
In order to maximise the amount of biodiesel produced from a feedstock, this study shows how to employ various machine learning algorithms, including linear regression, multilayer perceptron (MLP), and K-Nearest Neighbor (KNN). The technique used three different input metrics, including reaction duration, catalyst concentration, and methanol quantity/molar ratio. The anticipated output for NPLME, WPLME, and EPLME is the production yield. R2 and MAPE parameters were used to assess the model's performance.
Biodiesel production overview: Biodiesel is described as monoalkyl esters of long chain fatty acids generated from sustainable feed stocks (vegetable oil/fats) by Srivastava and Prasad. Due to its similarities to diesel, it can be used as a natural alternative to petroleum-based diesel and has the potential to minimise dangerous emissions. Biodiesel is an alternative fuel created by transesterication reaction from renewable sources, mostly vegetable oils; animal fats, or recycled cooking oils, according to Mathiyazhagan et al. It will have to play a larger role as the world's energy source in the future.
Bio-diesel can be made in a variety of ways. In the transesterification process, triglycerides react with three molecules of alcohol in the presence of a catalyst to create mono-alkyl esters of long-chain fatty acids and glycerol. Acids, bases, and enzymes can all catalyse the reaction. It is the most popular and commonly applied technique for converting vegetable oil into biodiesel [20]–[22]. It describes a chemical reaction that results in glycerol and mono-alkyl esters of long-chain fatty acids (biodiesel) from an oil (triglyceride) and an alcohol (methyl/ethanol) [23],[24]. The concentration of alcohol, the quantity of free fatty acids, the presence of water, the catalytic reaction, temperature, pressure, and duration are the primary variables impacting tranesterication [25],[26].
Vegetable oil's performance can be improved by altering it through the transesterification process. This procedure is used to reduce the viscosity of vegetable oil. Fatty acid chains joined chemically to one methanol molecule provide the basis of biodiesel [27]. Long chain fatty acids and three carbon backbones are features of vegetable oil. Triglyceride and alcohol are chemically reacting with one another in the presence of a catalyst [28]. Figure 1, depicts the chemical reactions involved in biodiesel synthesis [28],[29].
The most prevalent and widely used method for producing biodiesel is base catalysis. To create low-fat feed oil, it is employed. When a triglyceride and an alcohol interact in the presence of a catalyst, like a strong base (KOH/NaOH), they produce fatty alkyl esters (biodiesel) and glycerol [30]. Triglycerides are trancesterified using an alkaline catalyst in alcoholysis. The transesterification of vegetable oils via a base-catalyzed process is substantially faster than the acid-catalyzed reaction [31].
According to Maa and Hanna, the mechanism of the alkali-catalyzed reaction was devised in 3 stages. The first included the triglyceride's carbonyl carbon being struck by an alcohol anion, which led to the formation of a tetrahedral intermediate. In the second phase, the intermediate then interacts with an alcohol to regenerate the alcohol's anion. A fatty acid ester and a diglyceride are produced as a result of the third phase's rearranging of the tetraheldral intermediate [32]. Methanolysis is the term used when methanol is utilised in a transesterification reaction. The overall transesterification reaction is shown in Figure 1. However, it is believed that three consecutive and reversible processes take place. Triglycerides are first transformed into diglycerides, then into monoglycerides, and lastly into monoglycerides, which yield one methyl ester molecule from each glyceride. R1, R2, and R3 stand in for the triglyceride's fatty acid hydrocarbon chains.
Karanj and mahua oil (H2SO4) are strong acids or acid catalysed biodiesel feed oils with a large level of free fatty acids [33]. The high FFA concentration of the vegetable oil is reduced by the acid-catalyzed transesterification process to roughly 2% FFA. As shown, free fatty acids and methanol react when an acid catalyst like sulphuric acid is present [34],[35].
Haldar and Nag [36] reported that employing Lipase enzyme and methanol, they were able to produce bio-diesel through enzyme catalysed transesterification. Because of the ease of glycerol recovery, side reactions, and quick product separation, enzymatic biodiesel synthesis offers more advantages than chemical biodiesel manufacturing [37],[38].
With Novozym-435 lipase and supercritical carbon dioxide, biodiesel was created enzymatically. Lipase enzyme can be utilised as a catalyst in the transesterification process, according to Rathore and Giridhar. Microorganisms can be used to make lipase. The main drawback of the enzyme catalytic process is the high cost of lipases as a catalyst in given equation [39].
Supercritical methanol is used in a catalyst-free transesterification process. Purification becomes much easier, and the yield increases [40],[41]. A variety of feedstocks can be used with this approach since free fatty acids are converted to methyl esters instead of soap. Process of removing the catalyst is also skipped [42]. Production energy costs are on par with or lower than those of catalytic processes [43].
Reaction time, catalyst quantity, and the molar ratio of alcohol to oil are the most critical variables that influence the transesterification reaction. Each of these will be discussed separately in the further.
Alkali metal alkoxide has been reported to be more efficient than acidic catalysts for the triglyceride tranesterification reaction than enzyme or heterogeneous catalysts [44]. The conversion to vegetable oil esters was determined to be 94–99% at a concentration of 0.5–1% (w/w). When too much base catalyst is used in transesterification processes, the glycerides react with the base catalyst, resulting in the saponification process [45].
The molar ratio to triglyceride was one of the most crucial variables impacting the ester yield. The molar ratio calculates the ratio of glycerides to alcohol moles in an oil sample. To make three moles of fatty acid alkyl esters and one mole of glycerol through transestrification, three moles of alcohol and one mole of triglyceride are needed [46]. A higher molar ratio leads to a larger ester yield more quickly.
The conversion rate rises as reaction time increases. Since the conversion rate rises with reaction time, the reaction's outcome is clearly influenced by the reaction's reaction time [47]. A portion of the oil will remain unreacted if the reaction period is not long enough to provide a high ester yield [48].
The objectives of this experimental research are to identify the optimum reaction conditions for the methanolysis (transesterification) of enzymatic degummed and non-degummed Linseed oil for biodiesel production. The important process variables for transesterifiaction such as concentration of catalyst, alcohol/oil ratio and reaction time are optimized. Very few researchers have been worked with degummed feedstock's using alternative process.Vegetable oils must be degummed prior to transesterification process.
The methyl ester synthesis method was carried out using a single step alkali base catalysed transesterification from crude or pre-treated Linseed oil. Because the FFA content of these oils was less than 2 mg KOH/g, no pre-treatment of the feed stocks was required. As a result, the transesterification was carried out using methanol (CH3OH) and NaOH as a catalyst. The trials were carried out on a laboratory scale.
The three-necked glass flask was filled with linseed oil, either unrefined or refined. The flask was incubated for five hours between 48 and 50 degrees Celsius in a water bath with gentle agitation. The oil was heated to 80 °C for 15 minutes after five hours to inactivate the enzyme. After the enzyme was inactivated, the oil was heated to 60 °C, and 3 wt% hot distilled water was then added to the reaction flask. The system's temperature was held at 55 degrees Celsius for 15 minutes. The mixture was moved to a centrifuge while the stirrer was spinning at 400 rpm.
The methyl ester yield recovered by transesterification at various molar ratios, catalyst concentrations, and reaction times is shown in Table 1. Throughout the experiment, the reaction temperature (60 °C) and settling time (24 hours) were kept constant.
S. No | Reaction time (Hour) | Catalyst concentration % | Methanol quantity (gm) | Molar ratio | NPLME Ester yield % | WPLME Ester Yield% | EPLME Ester yield % |
1. | 0.5 | 0.2 | 15 | 4:1 | 50 | 70 | 75 |
2. | 0.5 | 0.4 | 15 | 4:1 | 60 | 83 | 85 |
3. | 0.5 | 0.6 | 15 | 4:1 | 55 | 79 | 80 |
4. | 0.5 | 0.8 | 15 | 4:1 | 49 | 72 | 74 |
5. | 0.5 | 1 | 15 | 4:1 | 45 | 59 | 60 |
6. | 1 | 0.2 | 15 | 4:1 | 52 | 89 | 83 |
7. | 1 | 0.4 | 15 | 4:1 | 56 | 88 | 90 |
8. | 1 | 0.6 | 15 | 4:1 | 63 | 85 | 87 |
9. | 1 | 0.8 | 15 | 4:1 | 60 | 82 | 84 |
10. | 1 | 1 | 15 | 4:1 | 55 | 78 | 79 |
11. | 1.5 | 0.2 | 15 | 4:1 | 54 | 75 | 80 |
12. | 1.5 | 0.4 | 15 | 4:1 | 62 | 84 | 87 |
13. | 1.5 | 0.6 | 15 | 4:1 | 59 | 82 | 84 |
14. | 1.5 | 0.8 | 15 | 4:1 | 50 | 74 | 76 |
15. | 1.5 | 1 | 15 | 4:1 | 40 | 63 | 64 |
16. | 0.5 | 0.2 | 22.2 | 6:1 | 52 | 78 | 81 |
17. | 0.5 | 0.4 | 22.2 | 6:1 | 63 | 85 | 86 |
18. | 0.5 | 0.6 | 22.2 | 6:1 | 56 | 81 | 84 |
19. | 0.5 | 0.8 | 22.2 | 6:1 | 53 | 75 | 79 |
20. | 0.5 | 1 | 22.2 | 6:1 | 50 | 72 | 78 |
21 | 1 | 0.2 | 22.2 | 6:1 | 60 | 85 | 87 |
22. | 1 | 0.4 | 22.2 | 6:1 | 68 | 89 | 93 |
23. | 1 | 0.6 | 22.2 | 6:1 | 65 | 87 | 90 |
24. | 1 | 0.8 | 22.2 | 6:1 | 62 | 85 | 87 |
25. | 1 | 1 | 22.2 | 6:1 | 59 | 83 | 85 |
26. | 1.5 | 0.2 | 22.2 | 6:1 | 54 | 80 | 82 |
27. | 1.5 | 0.4 | 22.2 | 6:1 | 65 | 89 | 90 |
28. | 1.5 | 0.6 | 22.2 | 6:1 | 60 | 84 | 86 |
29. | 1.5 | 0.8 | 22.2 | 6:1 | 55 | 82 | 85 |
30. | 1.5 | 1 | 22.2 | 6:1 | 52 | 81 | 84 |
31. | 0.5 | 0.2 | 30 | 8:1 | 58 | 75 | 83 |
32. | 0.5 | 0.4 | 30 | 8:1 | 65 | 88 | 89 |
33. | 0.5 | 0.6 | 30 | 8:1 | 62 | 85 | 87 |
34. | 0.5 | 0.8 | 30 | 8:1 | 58 | 84 | 85 |
35. | 0.5 | 1 | 30 | 8:1 | 56 | 83 | 84 |
36. | 1 | 0.2 | 30 | 8:1 | 68 | 80 | 90 |
37. | 1 | 0.4 | 30 | 8:1 | 74 | 95 | 98 |
38. | 1 | 0.6 | 30 | 8:1 | 70 | 95 | 96.2 |
39. | 1 | 0.8 | 30 | 8:1 | 65 | 94 | 95 |
40. | 1 | 1 | 30 | 8:1 | 60 | 92 | 94 |
41. | 1.5 | 0.2 | 30 | 8:1 | 60 | 82 | 85 |
42. | 1.5 | 0.4 | 30 | 8:1 | 68 | 91 | 93 |
43. | 1.5 | 0.6 | 30 | 8:1 | 65 | 87 | 90 |
44. | 1.5 | 0.8 | 30 | 8:1 | 60 | 85 | 89 |
45. | 1.5 | 1 | 30 | 8:1 | 58 | 84 | 87.1 |
46. | 0.5 | 0.2 | 59.2 | 16:1 | 30 | 52 | 55 |
47. | 0.5 | 0.4 | 59.2 | 16.1 | 34 | 58 | 60 |
48. | 0.5 | 0.6 | 59.2 | 16:1 | 32 | 56 | 57 |
49. | 0.5 | 0.8 | 59.2 | 16:1 | 20 | 49 | 51 |
50. | 0.5 | 1 | 59.2 | 16:1 | 15 | 39 | 40 |
51. | 1 | 0.2 | 59.2 | 16:1 | 28 | 40 | 55 |
52. | 1 | 0.4 | 59.2 | 16:1 | 39 | 64 | 66 |
53. | 1 | 0.6 | 59.2 | 16:1 | 35 | 59 | 60 |
54. | 1 | 0.8 | 59.2 | 16:1 | 30 | 54 | 55 |
55. | 1 | 1 | 59.2 | 16:1 | 20 | 45 | 46 |
56. | 1.5 | 0.2 | 59.2 | 16:1 | 30 | 53 | 55 |
57. | 1.5 | 0.4 | 59.2 | 16:1 | 36 | 58 | 64 |
58. | 1.5 | 0.6 | 59.2 | 16:1 | 34 | 57 | 58 |
59. | 1.5 | 0.8 | 59.2 | 16:1 | 25 | 52 | 53 |
60. | 1.5 | 1 | 59.2 | 16:1 | 18 | 40 | 42 |
The optimum condition was identified based on the higher % yield of methyl esters given in Table 2.
The following formula was used to compute the methyl esters' yield:
S. No. | Reaction temperature (ºC) | Reaction time (Hour) | Catalyst concentration % | Molar Ratio | NPLME Maximum Ester yield % | WPLME Maximum Ester yield % | EPLME Maximum Ester yield % |
1. | 60 | 1 | 0.4 | 4:1 | 56 | 86 | 90 |
2. | 60 | 1 | 0.4 | 6:1 | 73 | 89 | 93 |
3. | 60 | 1 | 0.4 | 8:1 | 74 | 95 | 98 |
4. | 60 | 1 | 0.4 | 16:1 | 36 | 64 | 66 |
It is an artificial intelligence algorithm built on the idea of supervised learning. Regression creates a model for the target prediction value based on the independent values or parameters. the main purpose of using a regression technique is mostly for calculating the relationship between variables and prediction. The kind of link between the dependent and independent instances they are analysing, together with the amount of independent variables being employed, completely decide the types of regression models.
The task of predicting a dependent variable's value (y) based on an independent variable is carried out using linear regression (x). As a result, this regression method determines that x and y have a linear relationship.
In Figure 2, X represents job experience as an input and Y represents a person's pay as an output. The regression line is the line that fits our model the best. The hypothesis function for Linear regression is
We are provided x and y while training the model. Where y is the data label and x is the input training data. In order to predict the value of y given a value for x, the model fits the best line during training. By choosing the optimal a and b values, the technique creates the best regression fit line. Where a is the intercept and b is the coefficient of x.
The best fit line is obtained after determining the best a and b values. As a result, when we ultimately use our model to make predictions, it will forecast the value of y given x as an input [49].
The KNN algorithm estimates the nearness of a data point to that of the other member of one group or another. It looks at the data points around the given data point to define the group of that data point. For example, for a given class- Group X and Group Y the algorithm, to determine its Group will look at the data points that are near to the given data point to see the Group with the majority of data points [50]. Distance between the members is measured by a distance function.
Algorithm 1: based on distance: building
Step 1. The division of data space.
Step 2. For each partition, pick a single reference point.
Step 3. Calculate the distance between the reference point and all of the data in the partition.
Algorithm 2: based on distance: KNN search
Step 1. Calculate the distances between the query item and each of the reference points for a given query item Q.
Step 2. Sort the divisions in a non-decreasing order based on their distances from Q.
Step 3. Using the triangle inequality from the nearest partition, look for KNNs of Q.
One of the methods to find the distance with the Euclidean distance formula.
where k is the number of neighbour members to be considered and x and y are the members between whose distance is measured [51],[52].
A MLP is one of the feedforward neural networks. As seen in Figure 3, a MLP contains three layers: input, hidden, and an output layer. All nodes, with the exception of the input nodes, are neurons, and has a nonlinear activation function. During training, MLP uses the supervised learning technique known as back propagation. Due to its multiple layers and non-linear activation, MLP differs from linear perceptron. Data that cannot be separated linearly can be differentiated using MLP [53],[54].
Algorithm 1: Multilayer perceptron learning process
Step 1. Starts with the inputs: The sum of the product of inputs and their weights.
Step 2. A bias Factor is added.
Step 3. Feeds the sum with the Activation Function
Step 4. The output of the perceptron is the outcome.
The analysis of the performances of the machine learning models is carried out using the R2 value and Mean Absolute Percentage Error (MAPE) value. R-squared (R2) is a statistical measure that shows how much of a dependent variable's variance can be accounted for by one or more independent variables in a model. The following is the R2 formula.
R-squared (R2)
Where, the actual data values and the modelled values' respective means are represented by the letters yi and ŷi, and yi stands for the modelled values and the original data values, respectively.
R2 is a percentage between 0 and 1 with no value closer to 1, indicating that the model captures a bigger amount of variation. For example, the R2 value of 0.94 means that the fit explains 94% of the total variation in the given data [55],[56].
Where yi, ŷi are the original and modelled data respectively (Best value = 0; Worst value = +∞)
MAPE has a fairly simple interpretation in terms of relative error. It is utilised in activities where sensitivity to relative variations over absolute variations is more crucial. However, it also has some drawbacks, the most significant of which are that it can only be used with strictly positive data by definition and that it is biassed toward low forecasts, making it inappropriate for predictive models where big mistakes are anticipated [57],[58].
As discussed above R2 and MAPE scores are used to test each model used in this study. Figures 4–6 and Table 3 show the performance of the models for each yield [59],[60]. The plots show that the KNN model has more points close to the lines with an R2 score close to 1 and a low MAPE score which implies that the prediction accuracy is better compared to Linear regression and MLP. Also, the R2 score of Linear regression and MLP is higher for Non Pre-treated Linseed Methyl Ester (NPLME). Moreover, the MAPE score of all models is less when calculated with Enzymatic Pre-treated Linseed Methyl Ester (EPLME) [60]–[64].
Model | NPLME |
WPLME |
EPLME |
|||
R2 | MAPE | R2 | MAPE | R2 | MAPE | |
Linear Regression | 0.7309 | 14.4841 | 0.6824 | 9.8820 | 0.6959 | 9.1998 |
KNN | 0.8680 | 7.9753 | 0.8217 | 5.5745 | 0.8765 | 4.7121 |
MLP | 0.8415 | 8.5512 | 0.7761 | 7.9917 | 0.7417 | 7.6580 |
Comparative results with existing model | ||||||
Models | R2 | MAPE | ||||
Boosted MLP [58] | 0.998 | 0.052 | ||||
Boosted LR [58] | 0.926 | 0.056 | ||||
Forest of decision trees[58] | 0.992 | 0.098 |
The brain's auto-learning and self-improving ability to answer the study's challenging questions is the inspiration for the machine learning approach, which offers greater prediction with the highest accuracy. Consequently, it is advantageous for the transesterification process and for real-time monitoring of the biodiesel system. Applications of machine learning in the procedure phase include quality optimization and estimation of the conditions of the process, with yield biodiesel serving as the output parameter and reaction time, catalyst concentration method, methanol quantity, and molar ratio serving as the input parameters.
This paper demonstrates the use of different machine learning techniques namely linear regression, Multilayer Perceptron (MLP), and K-Nearest Neighbour (KNN) to maximise biodiesel output from a feedstock. Three different input metrics such as reaction time, catalyst concentration, and methanol quantity/molar ratio was used in the technique, while the production yield is the predicted output for NPLME, WPLME, and EPLME. To evaluate the performance of the model, R2 and MAPE parameters were used. The average R2 values for linear regression, KNN, and MLP are 0.7030, 0.8554 and 0.7864 respectively. Moreover, the average MAPE values for these models are 11.1886, 6.0873 and 8.0669 respectively. Hence, it is observed that KNN model outperforms other models with higher accuracy and low MAPE score.
[1] | McDonnell K, Ward S, Leahy J, et al. (1999) Properties of rapeseed oil for use as a diesel fuel extender. J Am Oil Chem Soc 76: 539-543. https://doi.org/10.1007/s11746-999-0001-y |
[2] | Zufarov O, Schmidt S, Sekretar S (2008) Degumming of rapseed and sunflower oils. Acta Chemica Slovaca 1: 321-328. |
[3] | Fan X (2008) Optimization of biodiesel production from crude cottonseed oil and waste vegetable oil: Conventional and ultrasonic irradiation methods [PhD thesis]. USA: Clemson University. |
[4] | Ragit S (2011) Process standardization, characterization and experimental investigation on the performance of biodiesel fuelled C.I. engine [PhD thesis]. Patiala: Thapar university. |
[5] | Rashid U, Anwar F, Jamil A, et al. (2010) Jatropha curcas seed oil as a viable source for biodiesel. Pak J Bot 42: 575-582. |
[6] | Navindgi M, Dutta M, Kumar B (2012) Performance evaluation, emission characteristics and economic analysis of four non-edible straight vegetable oils on a single cylinder CI engine APRN. J Eng Appl Sci 7: 173-179. |
[7] | Giva S, Abdullah L, Adam N (2010) Investigating Egusi (Citrullus Colocynthis L.) seed oil as potential biodiesel feedstock. Energies 3: 607-618. https://doi.org/10.3390/en3040607 |
[8] | Heiden R (1996) Analytical methodologies for the determination of biodiesel ester purity determination of total methyl esters. National Biodiesel board . |
[9] | Sharma Y, Singh B, Upadhyay S (2008) Advanced in development and characterization of biodiesel: A review. Fuel 87: 2355-2373. https://doi.org/10.1016/j.fuel.2008.01.014 |
[10] | Leung DYC, Guo Y (2006) Transesterification of neat and used frying oil: optimization for biodiesel production. Fuel Process Technol 87: 883-890. https://doi.org/10.1016/j.fuproc.2006.06.003 |
[11] | Somnuk K, Smithmait P, Prateepchaikul G (2012) Feasibility of using ultrasound assisted biodiesel production from degummed deacidifies mixed crude palm oil using small scale Circulation. Agric Nat Reso 46: 662-669. |
[12] | Saqib M, Mumtaz MW, Mahmood A, et al. (2012) Optimized biodiesel production and environmental assessment produced biodiesel. Biotechnol Bioprocess Eng 17: 617-623. https://doi.org/10.1007/s12257-011-0569-6 |
[13] | Ramadhas AS, Jayaraj S, Muraleedharan C (2005) Biodiesel production from high FFA rubber seed oil. Fuel 84: 335-340. https://doi.org/10.1016/j.fuel.2004.09.016 |
[14] | Peddroso L, Ferreira J, Falco J, et al. PortugalBiodiesel alternative (2020). Available from https://ec.europa.eu/environment/nature/natura2000/management/docs/Annex%20report.pdf. |
[15] | Srivastava A, Prasad R (2000) Triglycerides-based diesel fuels. Renew Sust Energy Rev 4: 111-133. https://doi.org/10.1016/S1364-0321(99)00013-1 |
[16] | Bari S, Lim T, Yu C (2002) Effects of preheating crude palm oil (CPO) on injection system, performance and emission of a diesel engine. Renew Energy 27: 171-181. https://doi.org/10.1016/S0960-1481(02)00010-1 |
[17] | Pehan S, Jerman M, Kegl M, et al. (2009) Biodiesel influence on tribology characteristics of a diesel engine. Fuel 88: 970-979. https://doi.org/10.1016/j.fuel.2008.11.027 |
[18] | Devi J, Pachwania S (2018) Recent advancements in DNA interaction studies of organotin (IV) complexes. Inorg Chem Commun 91: 44-62. https://doi.org/10.1016/j.inoche.2018.03.012 |
[19] | Tariq M, Ali S, Muhammad N, et al. (2014) Biological screening, DNA interaction studies, and catalytic activity of organotin (IV) 2-(4-ethylbenzylidene) butanoic acid derivatives: synthesis, spectroscopic characterization, and X-ray structure. J Coord Chem 67: 323-340. https://doi.org/10.1080/00958972.2014.884217 |
[20] | Rathore V, Giridhar M (2007) Synthesis of bio-diesel from edible and non-edible oils in supercritical alcohols and enzymatic synthesis in supercritical carbon dioxide. Fuel 86: 2650-2659. https://doi.org/10.1016/j.fuel.2007.03.014 |
[21] | Dixit S, Rehman A (2012) Linseed oil as a potential resource for bio-diesel: a review. Renew Sust Energy Rev 16: 4415-4421. https://doi.org/10.1016/j.rser.2012.04.042 |
[22] | Wilson P (2010) Biodiesel production from Jatropha curcas: A review. Sci Res Essays 5: 1796-1808. |
[23] | Gupta KK, Rehman A, Sarviya RM (2010) Evaluation of soya bio-diesel as a gas turbine fuel. Iran J Energy Environ 1: 205-210. |
[24] | Tiwari P, Kumar R, Garg S (2006) Transesterification, modeling and simulation of batch kinetics of non-edible vegetable oils for biodiesel production. Annu Alche Meet. San Francisco: . |
[25] | Agarwal A (2007) Biofuels (alcohols and biodiesel) applications as fuels for internal combustion engines. Prog Energy Combust Sci 33: 233-271. https://doi.org/10.1016/j.pecs.2006.08.003 |
[26] | Rao Y, Voleti R, Raju A (2009) Experimental investigations on jatropha biodiesel and additive in diesel engine YV Hanumantha Rao1, Ram Sudheer Voleti1, AV Sitarama Raju2 and P. Nageswara Reddy3. Indian J Sci Technol 2: 25-31. https://doi.org/10.17485/ijst/2009/v2i4.13 |
[27] | Chatterjee M, Mitra J, Saha S (2010) Bio-diesel: Production through the Enzymatic Route [MD thesis]. India: Jadavpur University. |
[28] | Mathiyazhagan M, Ganapathi A, Jaganath B, et al. (2011) Production of biodiesel from non-edible plant oils having high FFA content. Int J Chem Environ Eng 2: 119-122. |
[29] | Raheman H, Phadatare AG (2003) Karanja esterified oil an alternative renewable fuel for diesel engines in controlling air pollution. Bioenergy News 7: 17-23. |
[30] | Srinivas P, Gopalakrishnan KV (1991) Vegetable oils and their methyl esters as fuels for diesel engines. Indian J Technol 29: 292-297. |
[31] | Shereena KM, Thangaraj T (2009) Bio-diesel: an alternative fuel produced from vegetable oils by transesterification. Elec J Biol 5: 67-74. |
[32] | Gondra Z Study of factors influencing the quality and yield of biodiesel produced by transesterification of vegetable oils (2009). |
[33] | Padhi S, Singh R (2010) Optimization of esterification and transesterification of Mahua (Madhuca Indica) oil for production of biodiesel. J Chem Pharm Res 2: 599-608. |
[34] | Naik M, Meher, L, Naik S, et al. (2008) Production of biodiesel from high free fatty acid Karanja (Pongamia pinnata) oil. Biomass Bioenergy 32: 354-357. https://doi.org/10.1016/j.biombioe.2007.10.006 |
[35] | Gandhi M, Ramu N, Raj SB (2011) Methyl ester production from Schlichera oleosa. Int J Pharm Sci Res 2: 1244-1250. |
[36] | Haldar S, Nag A (2008) Utilization of three non-edible vegetable oils for the production of biodiesel catalysed by enzyme. The Open Chem Eng J 2: 79-83. https://doi.org/10.2174/1874123100802010079 |
[37] | Shah S, Sharma S, Gupta M (2003) Enzymatic transesterification for biodiesel production. IJBB 40: 392-399. |
[38] | Ju Y, Vali S (2005) Rice bran oil as a potential resource for biodiesel: a review. J Sci Ind Res 64: 866-882. |
[39] | Helwani Z, Othman M, Aziz N, et al. (2009) Technologies for production of biodiesel focusing on green catalytic techniques: a review. Fuel Process Technol 90: 1502-1514. https://doi.org/10.1016/j.fuproc.2009.07.016 |
[40] | Bunkakiat K (2006) Continuous production of biodiesel via transesterifi-cation from vegetable oils in supercritical methanol. Energy Fuels 20: 812-817. https://doi.org/10.1021/ef050329b |
[41] | Kusdiana D, Saka S (2004) Effects of water on biodiesel fuel production by supercritical methanol treatment. Bioresource Technol 91: 289-295. https://doi.org/10.1016/s0960-8524(03)00201-3 |
[42] | Vera CR, D'Ippolito SA, Pieck CL, et al. Production of biodiesel by a two-step supercritical reaction process with adsorption refining, 2nd Mercosur Congress on Chemical Engineering. Rio de Janeiro (2005)2005: 14-18. |
[43] | Andualem B, Gessesse A (2012) Methods for refining of brebra (Millettiaferruginea) Oil for the production of biodiesel. World App Sci J 17: 407-413. |
[44] | Mamilla V, Mllikarjun M, Laxmi G, et al. (2011) Preparation of biodiesel from karanj oil. Int J Energy Eng 1: 94-100. https://doi.org/10.5963/IJEE0102008 |
[45] | Somnuk K, Smithmaitrie P, Prateepchaikul G (2012) Feasibility of using ultrasound-assisted biodiesel production from degummed-deacidified mixed crude palm oil using small-scale circulation. Agric Nat Res 46: 662-669. |
[46] | Hossain A, Boyce A, Salleh A, et al. (2010) Impacts of alcohol type, ratio and stirring time on the biodiesel production from waste canola oil. Afr J Agric Res 5: 1851-1859. https://doi.org/10.5897/AJAR09.135 |
[47] | Freedman B, Pryde E, Mounts T (1984) Variables affecting the yields of fatty esters from transesterified vegetable oils. J Am Oil Chem Soc 61: 1638-1643. https://doi.org/10.1007/BF02541649 |
[48] | Ramadhas A, Jayaraj S, Muraleedharan C (2005) Biodiesel production from high FFA rubber seed oil. Fuel 84: 335-340. https://doi.org/10.1016/j.fuel.2004.09.016 |
[49] | Schmidt A, Finan C (2018) Linear regression and the normality assumption. J Clin Epidemiol 98: 146-151. https://doi.org/10.1016/j.jclinepi.2017.12.006 |
[50] | Katrina W A guide to machine learning algorithms and their applications (2022). Available from: https://www.sas.com/en_us/insights/articles/analytics/machine-learning-algorithms-guide.html. |
[51] | Yang K, Shahabi C (2007) An efficient k nearest neighbor search for multivariate time series. Inform Comput 205: 65-98. https://doi.org/10.1016/j.ic.2006.08.004 |
[52] | Baran B (2021) Air quality Index prediction in besiktas district by artificial neural networks and k nearest neighbors. J Eng Sci Design 9: 52-63. https://doi.org/10.21923/jesd.671836 |
[53] | Li Y, Cao W (2019) An extended multilayer perceptron model using reduced geometric algebra. IEEE Access 7: 129815-129823. https://doi.org/10.1109/ACCESS.2019.2940217 |
[54] | Sreedharan M, Khedr A, Bannany M (2020) A multi-layer perceptron approach to financial distress prediction with genetic algorithm. Autom Control Comput Sci 54: 475-482. https://doi.org/10.3103/S0146411620060085 |
[55] | Schmidt A, Finan C (2018) Linear regression and the normality assumption. J Clin Epidemiol 98: 146-151. https://doi.org/10.1016/j.jclinepi.2017.12.006 |
[56] | Coben D, Colwell D, Macrae S, et al. (2003) Adult numeracy: Review of research and related literature. London: National Research and Development Centre for adult literacy and numeracy. |
[57] | Chicco D, Warrens MJ, Jurman G (2021) The coefficient of determination R-squared is more informative than SMAPE, MAE, MAPE, MSE and RMSE in regression analysis evaluation. PeerJ Comput Sci 7: e623. https://doi.org/10.7717/peerj-cs.623 |
[58] | Abdelbasset W, Elkholi S, Opulencia C, et al. (2022) Development of multiple machine-learning computational techniques for optimization of heterogenous catalytic biodiesel production from waste vegetable oil. Arabian J Chem 15: 103843. https://doi.org/10.1016/j.arabjc.2022.103843 |
[59] | Mathiyazhagan M, Ganapathi A (2011) Factor affecting biodiesel production. Res Plant Biol 1: 1-5. |
[60] | Singh P, Singh D (2010) Bio-diesel production through the use of different sources and characterization of oils and their esters as the substitute of diesel: a review. Renew Sust Energy Rev 14: 200-216. https://doi.org/10.1016/j.rser.2009.07.017 |
[61] | Sirajuddin M, Tariq M, Ali S (2015) Organotin (IV) carboxylates as an effective catalyst for the conversion of corn oil into biodiesel. J Organomet Chem 779: 30-38. https://doi.org/10.1016/j.jorganchem.2014.12.019 |
[62] | Tariq M, Qureshi A, Imran M, et al. (2019) biodiesel-a transesterified product of non-edible castor oil. Rev Roum Chim 64: 1027-1036. https://doi.org/10.33224/rrch/2019.64.12.02 |
[63] | Tariq M, Qureshi A, Karim S, et al. (2021) Synthesis, characterization and fuel parameters analysis of linseed oil biodiesel using cadmium oxide nanoparticles. Energy 222: 120014. https://doi.org/10.1016/j.energy.2021.120014 |
[64] | Karthikumar S, Ragavanandham V, Kanagaraj S, et al. (2014) Preparation, characterization and engine performance characteristics of used cooking sunflower oil based bio-fuels for a diesel engine. Adv Mater Res 984: 913-923. https://doi.org/10.4028/www.scientific.net/AMR.984-985.913 |
1. | Omojola Awogbemi, Daramy Vandi Von Kallon, Application of machine learning technologies in biodiesel production process—A review, 2023, 11, 2296-598X, 10.3389/fenrg.2023.1122638 | |
2. | Ria Aniza, Wei-Hsin Chen, Anélie Pétrissans, Anh Tuan Hoang, Veeramuthu Ashokkumar, Mathieu Pétrissans, A review of biowaste remediation and valorization for environmental sustainability: Artificial intelligence approach, 2023, 324, 02697491, 121363, 10.1016/j.envpol.2023.121363 | |
3. | Sojung Kim, Junyoung Seo, Sumin Kim, Machine Learning Technologies in the Supply Chain Management Research of Biodiesel: A Review, 2024, 17, 1996-1073, 1316, 10.3390/en17061316 | |
4. | Fahimeh Esmi, Ajay K. Dalai, Yongfeng Hu, Comparison of various machine learning techniques for modeling the heterogeneous acid-catalyzed alcoholysis process of biodiesel production from green seed canola oil, 2024, 12, 23524847, 321, 10.1016/j.egyr.2024.06.029 | |
5. | Vishal Sharma, Mei-Ling Tsai, Chiu-Wen Chen, Pei-Pei Sun, Parushi Nargotra, Cheng-Di Dong, Advances in machine learning technology for sustainable biofuel production systems in lignocellulosic biorefineries, 2023, 886, 00489697, 163972, 10.1016/j.scitotenv.2023.163972 | |
6. | Gurunathan Baskar, Rajendran Sivakumar, Seifedine Kadry, Cheng-Di Dong, Reeta Rani Singhania, Ramanujam Praveenkumar, Elumalai Raja Sathendra, Comparative studies on modeling and optimization of fermentation process conditions for fungal asparaginase production using artificial intelligence and machine learning techniques, 2024, 1082-6068, 1, 10.1080/10826068.2024.2367692 | |
7. | Christos Kyriklidis, Aikaterini Koutouvou, Konstantinos Moustakas, Vayos Karayannis, Constantinos Tsanaktsidis, Artificial Intelligence and Nature-Inspired Techniques on Optimal Biodiesel Production: A Review—Recent Trends, 2025, 18, 1996-1073, 768, 10.3390/en18040768 |
S. No | Reaction time (Hour) | Catalyst concentration % | Methanol quantity (gm) | Molar ratio | NPLME Ester yield % | WPLME Ester Yield% | EPLME Ester yield % |
1. | 0.5 | 0.2 | 15 | 4:1 | 50 | 70 | 75 |
2. | 0.5 | 0.4 | 15 | 4:1 | 60 | 83 | 85 |
3. | 0.5 | 0.6 | 15 | 4:1 | 55 | 79 | 80 |
4. | 0.5 | 0.8 | 15 | 4:1 | 49 | 72 | 74 |
5. | 0.5 | 1 | 15 | 4:1 | 45 | 59 | 60 |
6. | 1 | 0.2 | 15 | 4:1 | 52 | 89 | 83 |
7. | 1 | 0.4 | 15 | 4:1 | 56 | 88 | 90 |
8. | 1 | 0.6 | 15 | 4:1 | 63 | 85 | 87 |
9. | 1 | 0.8 | 15 | 4:1 | 60 | 82 | 84 |
10. | 1 | 1 | 15 | 4:1 | 55 | 78 | 79 |
11. | 1.5 | 0.2 | 15 | 4:1 | 54 | 75 | 80 |
12. | 1.5 | 0.4 | 15 | 4:1 | 62 | 84 | 87 |
13. | 1.5 | 0.6 | 15 | 4:1 | 59 | 82 | 84 |
14. | 1.5 | 0.8 | 15 | 4:1 | 50 | 74 | 76 |
15. | 1.5 | 1 | 15 | 4:1 | 40 | 63 | 64 |
16. | 0.5 | 0.2 | 22.2 | 6:1 | 52 | 78 | 81 |
17. | 0.5 | 0.4 | 22.2 | 6:1 | 63 | 85 | 86 |
18. | 0.5 | 0.6 | 22.2 | 6:1 | 56 | 81 | 84 |
19. | 0.5 | 0.8 | 22.2 | 6:1 | 53 | 75 | 79 |
20. | 0.5 | 1 | 22.2 | 6:1 | 50 | 72 | 78 |
21 | 1 | 0.2 | 22.2 | 6:1 | 60 | 85 | 87 |
22. | 1 | 0.4 | 22.2 | 6:1 | 68 | 89 | 93 |
23. | 1 | 0.6 | 22.2 | 6:1 | 65 | 87 | 90 |
24. | 1 | 0.8 | 22.2 | 6:1 | 62 | 85 | 87 |
25. | 1 | 1 | 22.2 | 6:1 | 59 | 83 | 85 |
26. | 1.5 | 0.2 | 22.2 | 6:1 | 54 | 80 | 82 |
27. | 1.5 | 0.4 | 22.2 | 6:1 | 65 | 89 | 90 |
28. | 1.5 | 0.6 | 22.2 | 6:1 | 60 | 84 | 86 |
29. | 1.5 | 0.8 | 22.2 | 6:1 | 55 | 82 | 85 |
30. | 1.5 | 1 | 22.2 | 6:1 | 52 | 81 | 84 |
31. | 0.5 | 0.2 | 30 | 8:1 | 58 | 75 | 83 |
32. | 0.5 | 0.4 | 30 | 8:1 | 65 | 88 | 89 |
33. | 0.5 | 0.6 | 30 | 8:1 | 62 | 85 | 87 |
34. | 0.5 | 0.8 | 30 | 8:1 | 58 | 84 | 85 |
35. | 0.5 | 1 | 30 | 8:1 | 56 | 83 | 84 |
36. | 1 | 0.2 | 30 | 8:1 | 68 | 80 | 90 |
37. | 1 | 0.4 | 30 | 8:1 | 74 | 95 | 98 |
38. | 1 | 0.6 | 30 | 8:1 | 70 | 95 | 96.2 |
39. | 1 | 0.8 | 30 | 8:1 | 65 | 94 | 95 |
40. | 1 | 1 | 30 | 8:1 | 60 | 92 | 94 |
41. | 1.5 | 0.2 | 30 | 8:1 | 60 | 82 | 85 |
42. | 1.5 | 0.4 | 30 | 8:1 | 68 | 91 | 93 |
43. | 1.5 | 0.6 | 30 | 8:1 | 65 | 87 | 90 |
44. | 1.5 | 0.8 | 30 | 8:1 | 60 | 85 | 89 |
45. | 1.5 | 1 | 30 | 8:1 | 58 | 84 | 87.1 |
46. | 0.5 | 0.2 | 59.2 | 16:1 | 30 | 52 | 55 |
47. | 0.5 | 0.4 | 59.2 | 16.1 | 34 | 58 | 60 |
48. | 0.5 | 0.6 | 59.2 | 16:1 | 32 | 56 | 57 |
49. | 0.5 | 0.8 | 59.2 | 16:1 | 20 | 49 | 51 |
50. | 0.5 | 1 | 59.2 | 16:1 | 15 | 39 | 40 |
51. | 1 | 0.2 | 59.2 | 16:1 | 28 | 40 | 55 |
52. | 1 | 0.4 | 59.2 | 16:1 | 39 | 64 | 66 |
53. | 1 | 0.6 | 59.2 | 16:1 | 35 | 59 | 60 |
54. | 1 | 0.8 | 59.2 | 16:1 | 30 | 54 | 55 |
55. | 1 | 1 | 59.2 | 16:1 | 20 | 45 | 46 |
56. | 1.5 | 0.2 | 59.2 | 16:1 | 30 | 53 | 55 |
57. | 1.5 | 0.4 | 59.2 | 16:1 | 36 | 58 | 64 |
58. | 1.5 | 0.6 | 59.2 | 16:1 | 34 | 57 | 58 |
59. | 1.5 | 0.8 | 59.2 | 16:1 | 25 | 52 | 53 |
60. | 1.5 | 1 | 59.2 | 16:1 | 18 | 40 | 42 |
S. No. | Reaction temperature (ºC) | Reaction time (Hour) | Catalyst concentration % | Molar Ratio | NPLME Maximum Ester yield % | WPLME Maximum Ester yield % | EPLME Maximum Ester yield % |
1. | 60 | 1 | 0.4 | 4:1 | 56 | 86 | 90 |
2. | 60 | 1 | 0.4 | 6:1 | 73 | 89 | 93 |
3. | 60 | 1 | 0.4 | 8:1 | 74 | 95 | 98 |
4. | 60 | 1 | 0.4 | 16:1 | 36 | 64 | 66 |
Model | NPLME |
WPLME |
EPLME |
|||
R2 | MAPE | R2 | MAPE | R2 | MAPE | |
Linear Regression | 0.7309 | 14.4841 | 0.6824 | 9.8820 | 0.6959 | 9.1998 |
KNN | 0.8680 | 7.9753 | 0.8217 | 5.5745 | 0.8765 | 4.7121 |
MLP | 0.8415 | 8.5512 | 0.7761 | 7.9917 | 0.7417 | 7.6580 |
Comparative results with existing model | ||||||
Models | R2 | MAPE | ||||
Boosted MLP [58] | 0.998 | 0.052 | ||||
Boosted LR [58] | 0.926 | 0.056 | ||||
Forest of decision trees[58] | 0.992 | 0.098 |
S. No | Reaction time (Hour) | Catalyst concentration % | Methanol quantity (gm) | Molar ratio | NPLME Ester yield % | WPLME Ester Yield% | EPLME Ester yield % |
1. | 0.5 | 0.2 | 15 | 4:1 | 50 | 70 | 75 |
2. | 0.5 | 0.4 | 15 | 4:1 | 60 | 83 | 85 |
3. | 0.5 | 0.6 | 15 | 4:1 | 55 | 79 | 80 |
4. | 0.5 | 0.8 | 15 | 4:1 | 49 | 72 | 74 |
5. | 0.5 | 1 | 15 | 4:1 | 45 | 59 | 60 |
6. | 1 | 0.2 | 15 | 4:1 | 52 | 89 | 83 |
7. | 1 | 0.4 | 15 | 4:1 | 56 | 88 | 90 |
8. | 1 | 0.6 | 15 | 4:1 | 63 | 85 | 87 |
9. | 1 | 0.8 | 15 | 4:1 | 60 | 82 | 84 |
10. | 1 | 1 | 15 | 4:1 | 55 | 78 | 79 |
11. | 1.5 | 0.2 | 15 | 4:1 | 54 | 75 | 80 |
12. | 1.5 | 0.4 | 15 | 4:1 | 62 | 84 | 87 |
13. | 1.5 | 0.6 | 15 | 4:1 | 59 | 82 | 84 |
14. | 1.5 | 0.8 | 15 | 4:1 | 50 | 74 | 76 |
15. | 1.5 | 1 | 15 | 4:1 | 40 | 63 | 64 |
16. | 0.5 | 0.2 | 22.2 | 6:1 | 52 | 78 | 81 |
17. | 0.5 | 0.4 | 22.2 | 6:1 | 63 | 85 | 86 |
18. | 0.5 | 0.6 | 22.2 | 6:1 | 56 | 81 | 84 |
19. | 0.5 | 0.8 | 22.2 | 6:1 | 53 | 75 | 79 |
20. | 0.5 | 1 | 22.2 | 6:1 | 50 | 72 | 78 |
21 | 1 | 0.2 | 22.2 | 6:1 | 60 | 85 | 87 |
22. | 1 | 0.4 | 22.2 | 6:1 | 68 | 89 | 93 |
23. | 1 | 0.6 | 22.2 | 6:1 | 65 | 87 | 90 |
24. | 1 | 0.8 | 22.2 | 6:1 | 62 | 85 | 87 |
25. | 1 | 1 | 22.2 | 6:1 | 59 | 83 | 85 |
26. | 1.5 | 0.2 | 22.2 | 6:1 | 54 | 80 | 82 |
27. | 1.5 | 0.4 | 22.2 | 6:1 | 65 | 89 | 90 |
28. | 1.5 | 0.6 | 22.2 | 6:1 | 60 | 84 | 86 |
29. | 1.5 | 0.8 | 22.2 | 6:1 | 55 | 82 | 85 |
30. | 1.5 | 1 | 22.2 | 6:1 | 52 | 81 | 84 |
31. | 0.5 | 0.2 | 30 | 8:1 | 58 | 75 | 83 |
32. | 0.5 | 0.4 | 30 | 8:1 | 65 | 88 | 89 |
33. | 0.5 | 0.6 | 30 | 8:1 | 62 | 85 | 87 |
34. | 0.5 | 0.8 | 30 | 8:1 | 58 | 84 | 85 |
35. | 0.5 | 1 | 30 | 8:1 | 56 | 83 | 84 |
36. | 1 | 0.2 | 30 | 8:1 | 68 | 80 | 90 |
37. | 1 | 0.4 | 30 | 8:1 | 74 | 95 | 98 |
38. | 1 | 0.6 | 30 | 8:1 | 70 | 95 | 96.2 |
39. | 1 | 0.8 | 30 | 8:1 | 65 | 94 | 95 |
40. | 1 | 1 | 30 | 8:1 | 60 | 92 | 94 |
41. | 1.5 | 0.2 | 30 | 8:1 | 60 | 82 | 85 |
42. | 1.5 | 0.4 | 30 | 8:1 | 68 | 91 | 93 |
43. | 1.5 | 0.6 | 30 | 8:1 | 65 | 87 | 90 |
44. | 1.5 | 0.8 | 30 | 8:1 | 60 | 85 | 89 |
45. | 1.5 | 1 | 30 | 8:1 | 58 | 84 | 87.1 |
46. | 0.5 | 0.2 | 59.2 | 16:1 | 30 | 52 | 55 |
47. | 0.5 | 0.4 | 59.2 | 16.1 | 34 | 58 | 60 |
48. | 0.5 | 0.6 | 59.2 | 16:1 | 32 | 56 | 57 |
49. | 0.5 | 0.8 | 59.2 | 16:1 | 20 | 49 | 51 |
50. | 0.5 | 1 | 59.2 | 16:1 | 15 | 39 | 40 |
51. | 1 | 0.2 | 59.2 | 16:1 | 28 | 40 | 55 |
52. | 1 | 0.4 | 59.2 | 16:1 | 39 | 64 | 66 |
53. | 1 | 0.6 | 59.2 | 16:1 | 35 | 59 | 60 |
54. | 1 | 0.8 | 59.2 | 16:1 | 30 | 54 | 55 |
55. | 1 | 1 | 59.2 | 16:1 | 20 | 45 | 46 |
56. | 1.5 | 0.2 | 59.2 | 16:1 | 30 | 53 | 55 |
57. | 1.5 | 0.4 | 59.2 | 16:1 | 36 | 58 | 64 |
58. | 1.5 | 0.6 | 59.2 | 16:1 | 34 | 57 | 58 |
59. | 1.5 | 0.8 | 59.2 | 16:1 | 25 | 52 | 53 |
60. | 1.5 | 1 | 59.2 | 16:1 | 18 | 40 | 42 |
S. No. | Reaction temperature (ºC) | Reaction time (Hour) | Catalyst concentration % | Molar Ratio | NPLME Maximum Ester yield % | WPLME Maximum Ester yield % | EPLME Maximum Ester yield % |
1. | 60 | 1 | 0.4 | 4:1 | 56 | 86 | 90 |
2. | 60 | 1 | 0.4 | 6:1 | 73 | 89 | 93 |
3. | 60 | 1 | 0.4 | 8:1 | 74 | 95 | 98 |
4. | 60 | 1 | 0.4 | 16:1 | 36 | 64 | 66 |
Model | NPLME |
WPLME |
EPLME |
|||
R2 | MAPE | R2 | MAPE | R2 | MAPE | |
Linear Regression | 0.7309 | 14.4841 | 0.6824 | 9.8820 | 0.6959 | 9.1998 |
KNN | 0.8680 | 7.9753 | 0.8217 | 5.5745 | 0.8765 | 4.7121 |
MLP | 0.8415 | 8.5512 | 0.7761 | 7.9917 | 0.7417 | 7.6580 |
Comparative results with existing model | ||||||
Models | R2 | MAPE | ||||
Boosted MLP [58] | 0.998 | 0.052 | ||||
Boosted LR [58] | 0.926 | 0.056 | ||||
Forest of decision trees[58] | 0.992 | 0.098 |