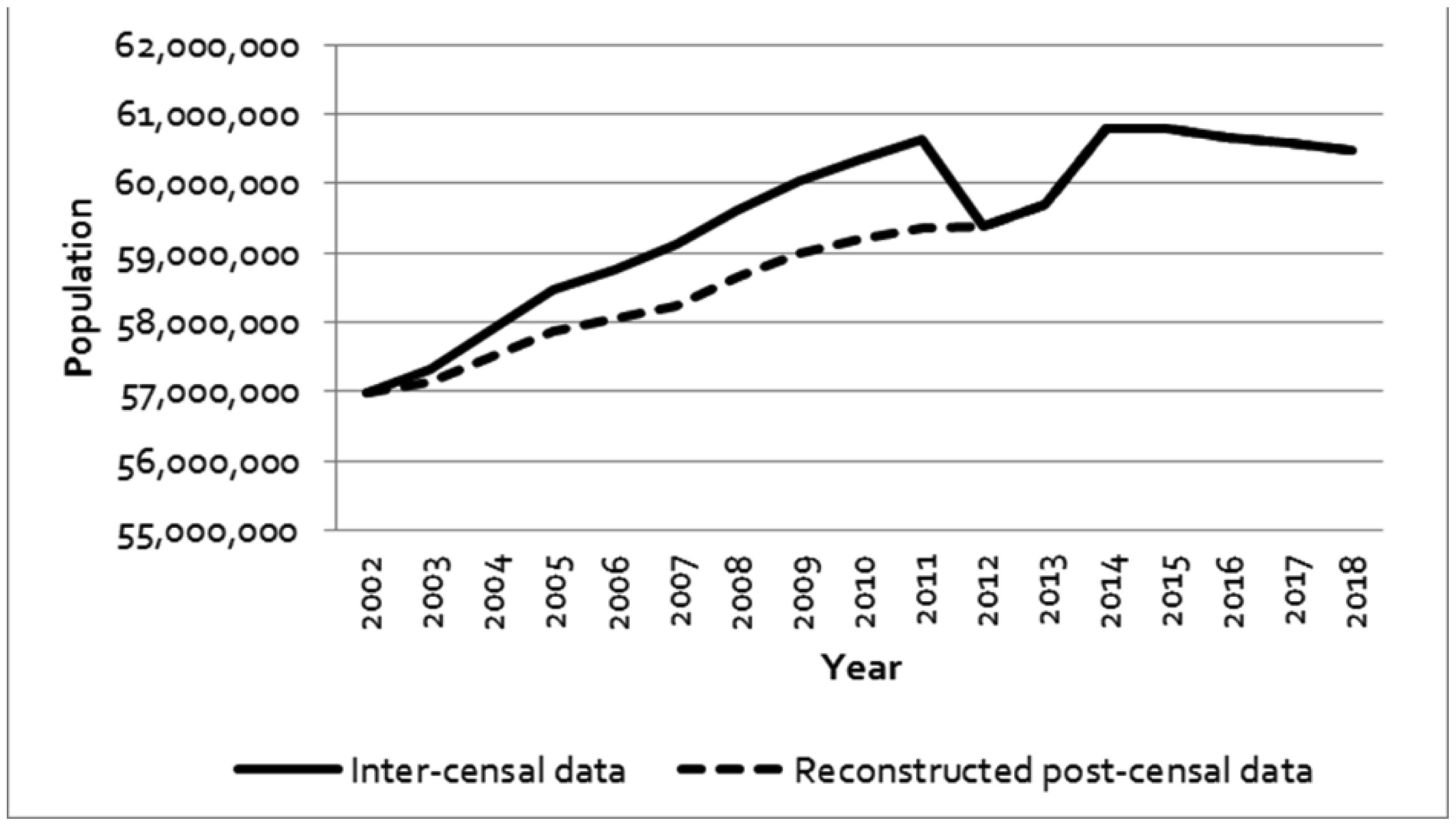
Citation: Gretchen H. Thompson, Rachel Lenzi, James M. MacCarthy, Aulive Msoma, Bagrey Ngwira, Heather Chotvacs. Health communication effects on food safety behaviors: A regression analysis of the determinants of food safety behaviors in health communication for life rural program districts[J]. AIMS Agriculture and Food, 2020, 5(4): 578-598. doi: 10.3934/agrfood.2020.4.578
[1] | Aaron C Shang, Kristen E Galow, Gary G Galow . Regional forecasting of COVID-19 caseload by non-parametric regression: a VAR epidemiological model. AIMS Public Health, 2021, 8(1): 124-136. doi: 10.3934/publichealth.2021010 |
[2] | Julia Metelka, Colin Robertson, Craig Stephen . Japanese Encephalitis: Estimating Future Trends in Asia. AIMS Public Health, 2015, 2(4): 601-615. doi: 10.3934/publichealth.2015.4.601 |
[3] | Mathieu Philibert, Mark Daniel . Predictors of Residential Mobility among Older Canadians and Impact on Analyses of Place and Health Relationships. AIMS Public Health, 2015, 2(1): 115-131. doi: 10.3934/publichealth.2015.1.115 |
[4] | Diane Hindmarsh, David Steel . Creating local estimates from a population health survey: practical application of small area estimation methods. AIMS Public Health, 2020, 7(2): 403-424. doi: 10.3934/publichealth.2020034 |
[5] | Helga Hallgrimsdottir, Leah Shumka, Catherine Althaus, Cecilia Benoit . Fear, Risk, and the Responsible Choice: Risk Narratives and Lowering the Rate of Caesarean Sections in High-income Countries. AIMS Public Health, 2017, 4(6): 615-632. doi: 10.3934/publichealth.2017.6.615 |
[6] | Okan Bulut, Cheryl N. Poth . Rapid assessment of communication consistency: sentiment analysis of public health briefings during the COVID-19 pandemic. AIMS Public Health, 2022, 9(2): 293-306. doi: 10.3934/publichealth.2022020 |
[7] | Rosemary Mamka Anyona, Maximilian de Courten . An Analysis of the Policy Environment Surrounding Noncommunicable Diseases Risk Factor Surveillance in Kenya. AIMS Public Health, 2014, 1(4): 256-274. doi: 10.3934/publichealth.2014.4.256 |
[8] | Margo Barr, Raymond Ferguson, Jason van Ritten, Phil Hughes, David Steel . Summary of the Impact of the Inclusion of Mobile Phone Numbers into the NSW Population Health Survey in 2012. AIMS Public Health, 2015, 2(2): 210-217. doi: 10.3934/publichealth.2015.2.210 |
[9] | Gareth Morgan . Does Utilitarian Policy such as Smoking Cessation Lend Support to Wider Aspirin Use?. AIMS Public Health, 2015, 2(2): 223-226. doi: 10.3934/publichealth.2015.2.223 |
[10] | Mehreen Tariq, Margaret Haworth-Brockman, Seyed M Moghadas . Ten years of Pan-InfORM: modelling research for public health in Canada. AIMS Public Health, 2021, 8(2): 265-274. doi: 10.3934/publichealth.2021020 |
Population estimates are of paramount importance in epidemiology since they represent denominators of occurrence measures and can be involved in calculation of risk rate ratios. For this reason, almost all high income countries in the world have developed national open-access databases that can be freely used by all scientists for obtaining population data stratified by gender, age and area level (state, region, province, city etc) [1]. In Europe these data are collected on an annual basis by the European Commission and include data on general population, live births and deaths occurred during the reference year and, usually, the total number of immigrated and emigrated subjects during the course of the year [2].
All these datasets are daily consulted by researchers for calculating incidence rates and prevalence of diseases as well as trends over time, comparing different areas or sub-populations with different demographic patterns.
In a large majority of studies that use public demographic data, scientists have to consider the role of data accuracy and completeness, since variations in these measures could lead to biased estimates and, consequently, to imprecise or erroneous epidemiological conclusions. In particular, in the last decades there has been an increasing interest in this active area of research with analysts trying to improve denominator estimates, evaluating the importance of this uncertainty and developing methods to handle the problem. In this sense, in Italy some criticisms in population registration process seem to be occurred in the years following the 2011 national Census [3]. There is the suspicion that largely fluctuating population sizes, when numerator (“cases of diseases”) is stable over time, could statistically significantly affect differences between occurrence rates.
According to these perplexities, by using data collected by the Italian National Institute of Statistics [4], this study has carried out a simulation of the impact of population size variability on incidence rates between couple of consecutive years with the same number of incident cases.
All data used in this study have been downloaded by the demo.istat web section of the ISTAT [5], that is an Italian public research organization producing official statistics in the service of citizens and policy-makers. In particular data from 1st January to 2002 to 1st January 2015 were considered with stratification by age, sex and Italian region of residency. These data are based on a continuous observation of the municipalities existing and considering the territorial evolution through time (birth and death of municipalities by aggregation, disaggregation, transfer from a province or region to another).
For years from 2002 to 2011 inter-censal resident population estimates, reconstructed backward by the ISTAT after the release of the Legal Population of Municipalities at the 2011 Population Census, were also used [5].
For the simulation analysis, years from 2011 to 2014 were considered. For each year (denoted as “index year”), expected cases were calculated at increasing arbitrarily fixed incidence rates (1/100,000; 10/100,000; 100/100,000 and 1,000/100,000), and the number of cases found in the “index year” (e.g. 2011) weas used as numerator for the “following year” (e.g. 2012), thus hypothesizing a constant number of cases between consecutive years. Arbitrarily fixed incidence of each “index year” was then compared with incidence in the “following year” in order to evaluate statistically significant differences, on a regional and national basis. For each comparison, relative risks (RRs) were calculated considering the “index year” as reference. P-value for testing the hypothesis that the true RR is equal to 1 and 95% confidence intervals were computed according to Mantel-Haenszel method proposed by Rothman and Greendland [6].
All calculations have been made by using R software version 3.5.2 [7].
Figure 1 depicts trends of annual Italian population from 2002 to 2018. For years 2002 to 2011 data included both inter-censal and reconstructed post-censal series. As shown, in 2011 data from Census reported 1,261,752 less residents than those calculated in inter-censal series (59,433,744 vs. 60,785,753, respectively). From 2013 to 2014 a significant increase in Italian population was recorded (from 59,685,227 in 2013 to 60,782,668 in 2014).
In Figure 2 the population percent change during a four year period (2011 to 2014) was reported for all Italian regions. In 2012 a decrease in population size was registered in all Italian regions (from −4.0% for Lazio to −0.73% for Trentino-Alto Adige) whereas in 2014 all regions recorded an increase in population size ranging from +5.6% (Lazio) to +0.38% (Basilicata).
In Table 1, trends of annual percentage changes stratified by age and sex have been reported. A particular decrease was evident in 2012, in both males and females, for 0–19 (−2.51% and −2.24%, respectively) and 20–39 (−5.22% and 4.33%, respectively) years old age groups. Otherwise subjects aged 80 years or more had a substantial increase in 2014 (+4.03% in males and +2.77% in females).
Males |
Females |
|||||||
2011 | 2012 | 2013 | 2014 | 2011 | 2012 | 2013 | 2014 | |
0–19 | 0.03% | −2.51% | 0.04% | 1.43% | 0.01% | −2.24% | −0.09% | 1.03% |
20–39 | −1.22% | −5.22% | −1.32% | 0.45% | −1.10% | −4.33% | −1.50% | −0.14% |
40–59 | 1.22% | −1.36% | 1.69% | 3.40% | 1.49% | −0.53% | 1.57% | 3.13% |
60–79 | 1.04% | −0.66% | 1.19% | 2.25% | 0.52% | −0.91% | 0.72% | 1.60% |
80–100 | 4.74% | 1.96% | 3.58% | 4.03% | 3.47% | 0.77% | 2.31% | 2.77% |
All age groups | 0.43% | −2.33% | 0.57% | 2.06% | 0.52% | −1.75% | 0.42% | 1.63% |
2012 vs. 2011 | 2013 vs. 2012 | 2014 vs. 2013 | |
Abruzzo | 1.0275d | 0.9954 | 0.9839 |
Aosta Valley | 1.0127 | 0.9904 | 0.9942 |
Apulia | 1.0102 | 0.9998 | 0.9904 |
Basilicata | 1.0172 | 1.0024 | 0.9962 |
Calabria | 1.0271d | 1.0001 | 0.9887 |
Campania | 1.0121d | 0.9991 | 0.9829d |
Emilia Romagna | 1.021d | 0.9917 | 0.9845d |
Friuli-Venezia Giulia | 1.0148 | 0.9967 | 0.9939 |
Lazio | 1.0416c,d | 0.9897 | 0.9467c,d |
Liguria | 1.0315d | 1.0014 | 0.9832 |
Lombardy | 1.0224d | 0.9904d | 0.9821d |
Marche | 1.016 | 0.9971 | 0.9949 |
Molise | 1.0212 | 0.9994 | 0.9956 |
Piedmont | 1.0229d | 0.9963 | 0.9859d |
Sardinia | 1.0229d | 0.9985 | 0.9859 |
Sicily | 1.0102 | 0.9999 | 0.9814d |
Tuscany | 1.0224d | 0.9932 | 0.9846d |
Trentino-Alto Adige | 1.0073 | 0.9900 | 0.9886 |
Umbria | 1.0263 | 0.9966 | 0.9883 |
Veneto | 1.0173d | 0.9942 | 0.9909 |
Italy | 1.0207c,d | 0.9951d | 0.9819c,d |
Note: Statistically significant difference with “index year” fixed at incidence rate: (a) 1/100,000; (b) 10/100,000; (c) 100/100,000 and (d) 1,000/100,000.
Table 2 summarizes comparisons between each couple of years (“index year” as reference). Statistically significant higher RRs (ranging from 1.012 and 1.0416) were found in 2012 vs. 2011 in Italy and in other 11 regions for “index year” incidence fixed at 1,000/100,000 cases per year and in Italy and Lazio for “index year” incidence fixed at 100/100,000 cases per year. In 2013 vs. 2012 Italy and Lazio showed statistically significant lower RRs for “index year” incidence rates fixed at 1,000/100,000 cases per year.
In 2014 vs. 2013 statistically significant lower RRs (ranging from 0.9467 and 0.9846) were found in Italy and in other 6 regions for “index year” incidence fixed at 1,000/100,000 cases per year and in Italy and Lazio for “index year” incidence fixed at 100/100,000 cases per year.
The present paper intended to evaluate a methodological aspect that seems to be commonly neglected during epidemiological investigations on general population including those carried out by cancer and mortality registries, epidemiological surveillance systems and health agency reports. The question under discussion is if population data, used as denominator in epidemiological studies, can represent a source of bias and how large can be the weight of this bias when present. Some authors suggest that errors in denominator terms can have a non-trivial impact on the results. In particular, in suburbanized areas population size can vary up to a 20%. When discrepancies of this magnitude occur an estimated incidence rate ratio can be 1.5 times the true ratio [8]. Other authors have estimated that over 10-year periods starting at the previous census, a substantial amount of error may accumulate ranging between as low as 10% and as high as 80% within any age/sex five-year age group [9],[10].
For investigating this issue, a simulation on Italian data collected by a national research organization (ISTAT) has been performed. The hypothesis was that a relatively large variation of population size between years with a constant number of cases could determine statistically significant differences in incidence rates. These differences are of great interest since they could represent the basis for implementing public health control strategies that could be avoided with unbiased population estimations. The simulation seems to confirm that significant differences due to population size could be found between years, both at a regional or national level, when investigating diseases with medium-high incidence rates (usually >100/100,000). This finding is quite expected since as the number of cases or events (numerator) and the study population (denominator) increase the relative width of the confidence interval becomes narrower [11]. In particular in 2012, the important decrease in population size at fixed number of cases determined a consequent increase in incidence rates with higher RRs, that were statistically significant at a national level and also in some regions. Otherwise in 2014 the increase in population size lowered incidence rates with protective RRs with respect to 2013.
It should be noted that the ISTAT have clarified that, between 2011 and 2014, the variability in population size could be at least in part attributable to some discrepancies between 2011 Census and Legal Population of Municipalities data. In this period, these discrepancies have determined 1,243,957 corrections (1,610,058 in plus and 366,101 in minus) of which about 85% was performed in 2013 [3].
In the pre-census period, some Italian authors have found that using inter-censal population as denominator for the year 2002–2011 produces a remarkable distortion of both temporal trend and geographical comparisons for cancer incidence rates, confirming some perplexities reported in this paper [12]. Some other authors have highlighted that particular race-specific estimates could prove far less reliable, with severe overestimates and underestimates of all racial groups in various counties nationwide [13].
Considering the reported problems in estimating population size and in absence of gold standard data, researchers should be encouraged to implement actions for reducing the risk of biased population denominators, especially for years where denominators are known to or have been declared to be fluctuating [14]–[17].
Ignoring the problem could be considered a possible solution when the numerator has a quit small incidence rates (<100/100,000 as we have simulated) or when variations in the size of the population are likely to be very small compared to the estimated effects. Otherwise, when one of the two previous situations is not satisfied, the international literature suggests several ways to try to take into account these problems. The first of these possibilities is to add to the statistical model a variable that allow the researchers to control for a proxy that is theoretically related to the size of the exposed population. A second possibility is to perform a mathematical estimation of the denominator by using some other data (for example interpolation by using other years data or moving means) or evaluating results by a sensitivity analysis [18] or other methods [19]. Finally, it can be also considered the possibility to deal with problem by study design as in the case of case-crossover design where population denominators are not used. More recently Jung et al have reported that, in some circumstances, new adjustment method dramatically enhances the statistical validity of global and local spatial autocorrelation statistics [20].
Of course, the present study has several limitations. First of all, it is a simulation and thus it could be not representative of events in the real life. As second point, demographic data could be more accurate than that assumed in this paper and thus, in this last case, calculated RRs would have estimated the true incidence rate ratios. Moreover, we have chosen to not add a further random noise to the numerator since our model considers that numerators (and, thus, the cases of diseases) are observed with a very high accuracy whereas when considering diseases with concerns of case-finding our model could be subjected to a type I error.
Finally, incidence rates were compared without adjustment for age and sex that could have an impact on estimates. In this sense, the large variability found in Italian population changes by age groups in different years seems to suggest that adjusted-rates could increase the statistical significant differences between estimates.
Despite these possible limits, this paper shows that in epidemiological studies the attention directed toward the ascertainment of accurate numerators can be not considered enough to warrant unbiased estimates when denominators are fluctuating and potentially inaccurate.
[1] | Shephard GS (2008) Impact of mycotoxins on human health in developing countries. Food Addit & Contam: Part A 25: 146-151. |
[2] |
Bennett J, Klich M (2003) Mycotoxins. Clin Microbiol Rev 16: 497-516. doi: 10.1128/CMR.16.3.497-516.2003
![]() |
[3] | Sun G, Wang S, Hu X, et al. (2007) Fumonisin B1 contamination of home-grown corn in high-risk areas for esophageal and liver cancer in China. Food Addit & Contam 24: 181-185. |
[4] |
Khlangwiset P, Shephard GS, Wu F (2011) Aflatoxins and growth impairment: A review. Crit Rev Toxicol 41: 740-755. doi: 10.3109/10408444.2011.575766
![]() |
[5] | Ferlay J, Soerjomataram I, Dikshit R, et al. (2014) Cancer incidence and mortality worldwide: Sources, methods and major patterns in GLOBOCAN 2012. Int J Cancer 136: E359-E386. |
[6] |
Abdallah MF, De Boevre M, Audenaert K, et al. (2018) Highlight report: Mycotoxins as food contaminants in Africa-challenges and perspectives. Arch Toxicol 92: 2151-2152. doi: 10.1007/s00204-018-2203-2
![]() |
[7] | Stepman F (2018) Scaling-up the impact of aflatoxin research in Africa. The role of social sciences. Toxins 10: 136. |
[8] | Khonga E (1985) Survey of fungi and mycotoxins in malts used in brewing opaque beer in Malawi. Luso: J Sci Technol 6: 49-56. |
[9] |
Matumba L, Monjerezi M, Biswick T, et al. (2014) A survey of the incidence and level of aflatoxin contamination in a range of locally and imported processed foods on Malawian retail market. Food Control 39: 87-91. doi: 10.1016/j.foodcont.2013.09.068
![]() |
[10] |
Matumba L, Monjerezi M, van Poucke C, et al. (2013) Evaluation of the bright greenish yellow fluorescence test as a screening technique for aflatoxin-contaminated maize in Malawi. World Mycotoxin J 6: 367-373. doi: 10.3920/WMJ2013.1563
![]() |
[11] | Matumba L, Sulyok M, Monjerezi M, et al. (2014) Fungal metabolites diversity in maize and associated human dietary exposures relate to micro-climatic patterns in Malawi. World Mycotoxin J 8: 269-282. |
[12] |
Matumba L, Van Poucke C, Monjerezi M, et al. (2015) Concentrating aflatoxins on the domestic market through groundnut export: A focus on Malawian groundnut value and supply chain. Food Control 51: 236-239. doi: 10.1016/j.foodcont.2014.11.035
![]() |
[13] |
Monyo E, Njoroge SMC, Coe R, et al. (2012) Occurrence and distribution of aflatoxin contamination in groundnuts (Arachis hypogaea L) and population density of aflatoxigenic aspergilli in Malawi. Crop Prot 42: 149-155. doi: 10.1016/j.cropro.2012.07.004
![]() |
[14] |
Misihairabgwi JM, Ezekiel CN, Sulyok M, et al. (2019) Mycotoxin contamination of foods in Southern Africa: A 10-year review (2007-2016). Crit Rev Food Sci Nutr 59: 43-58. doi: 10.1080/10408398.2017.1357003
![]() |
[15] |
Alberts JF, Lilly M, Rheeder JP, et al. (2017) Technological and community-based methods to reduce mycotoxin exposure. Food Control 73: 101-109. doi: 10.1016/j.foodcont.2016.05.029
![]() |
[16] | Matumba L, Monjerezi M, Kankwamba H, et al. (2015) Knowledge, attitude, and practices concerning presence of molds in foods among members of the general public in Malawi. Mycotoxin Res 32: 27-36. |
[17] |
Strosnider H, Azziz-Baumgartner E, Banziger M, et al. (2006) Workgroup report: Public health strategies for reducing aflatoxin exposure in developing countries. Environ Health Perspect 114: 1898-1903. doi: 10.1289/ehp.9302
![]() |
[18] |
Wu F, Mitchell NJ, Male D, et al. (2014) Reduced foodborne toxin exposure is a benefit of improving dietary diversity. Toxicol Sci 141: 329-334. doi: 10.1093/toxsci/kfu137
![]() |
[19] | Lovo S, Veronesi M (2015) Crop diversification and child health: Empirical evidence from Tanzania. London: The Centre for Climate Change Economics and Policy (CCCEP); The Grantham Research Institute on Climate Change and the Environment. |
[20] | Wu F, Khlangwiset P (2010) Evaluating the technical feasibility of aflatoxin risk reduction strategies in Africa. Food Addit&Contam Part A, Chem, Anal, Control, Exposure&Risk Assess 27: 658-676. |
[21] | Ansari-Lari M, Soodbakhsh S, Lakzadeh L (2009) Knowledge, attitudes and practices of workers on food hygienic practices in meat processing plants in Fars, Iran. Food Control 21: 260-263. |
[22] | Awuah RT, Agyemang KO, Fialor SC, et al. (2008) Are Ghanaians aware of the alatoxin menace? In: Leslie JF, Bandyopadhyay R, Visconti A, Mycotoxins: Detection Methods, Management, Public Health and Agricultural Trade. Cambridge, MA: CAB International, 327-334. |
[23] | Bas M, Ersun AS, Gokhan K (2004) The evaluation of food hygiene knowledge, attitudes, and practices of food handlers in food businesses in Turkey. Food Control 17: 317-322. |
[24] | Nee SO, Sani NA (2011) Assessment of knowledge, attitudes and practices (KAP) among food handlers at residential colleges and canteen regarding food safety. Sains Malaysiana 40: 403-410. |
[25] |
Soares LS, Almeida RCC, Cerqueira ES, et al. (2012) Knowledge, attitudes and practices in food safety and the presence of coagulasepositive staphylococci on hands of food handlers in the schools of Camaçari, Brazil. Food Control 27: 206-213. doi: 10.1016/j.foodcont.2012.03.016
![]() |
[26] |
Azaman NNM, Kamarulzaman NH, Shamsudin MN, et al. (2016) Stakeholders' knowledge, attitude, and practices (KAP) towards aflatoxins contamination in peanut-based products. Food Control 70: 249-256. doi: 10.1016/j.foodcont.2016.05.058
![]() |
[27] |
Ilesanmi FF, Ilesanmi OS (2011) Knowledge of aflatoxin contamination in groundnut and the risk of its ingestion among health workers in Ibadan, Nigeria. Asian Pac J Tropical Biomed 1: 493-495. doi: 10.1016/S2221-1691(11)60108-1
![]() |
[28] |
Jolly CM, Bayard B, Awuah RT, et al. (2009) Examining the structure of awareness and perceptions of groundnut aflatoxin among Ghanaian health and agricultural professionals and its influence on their actions. J Socio-Econ 38: 280-287. doi: 10.1016/j.socec.2008.05.013
![]() |
[29] |
Ezekiel CN, Sulyok M, Babalola DA, et al. (2013) Incidence and consumer awareness of toxigenic Aspergillus section Flavi and aflatoxin B1 in peanut cake from Nigeria. Food Control 30: 596-601. doi: 10.1016/j.foodcont.2012.07.048
![]() |
[30] | Sanders M, De Middelseer G, Vervaet S, et al. (2014) The awareness about mycotoxin contamination of food and feed: A survey in the Flemish population. World Mycotoxin J 8: 375-380. |
[31] |
Anitha S, Tsusaka TW, Njoroge SM, et al. (2019) Knowledge, attitude and practice of Malawian farmers on pre- and post-harvest crop management to mitigate aflatoxin contamination in groundnut, maize and sorghum-implication for behavioral change. Toxins 11: 716. doi: 10.3390/toxins11120716
![]() |
[32] |
Mboya RM, Kolanisi U (2014) Subsistence farmers' mycotoxin contamination awareness in the SADC Region: Implications on Millennium Development Goal 1, 4 and 6. J Human Ecol 46: 21-31. doi: 10.1080/09709274.2014.11906702
![]() |
[33] | Magembe KS, Mwatawala MW, Mamiro DP, et al. (2016) Assessment of awareness of mycotoxins infections in stored maize (Zea mays L.) and groundnut (arachis hypogea L.) in Kilosa District, Tanzania. Int J Food Contam 3: 12. |
[34] |
Beyene AA, Woldegiorgis AZ, Adish AA, et al. (2016) Assessment of mothers' knowledge and practice towards aflatoxin contamination in complementary foods in Ethiopia: From pre-harvest to household. World Mycotoxin J 9: 535-544. doi: 10.3920/WMJ2016.2088
![]() |
[35] |
Kumar GDS, Popat MN (2010) Farmers' perceptions, knowledge and management of aflatoxins in groundnuts (Arachis hypogaea L.) in India. Crop Prot 29: 1534-1541. doi: 10.1016/j.cropro.2010.08.019
![]() |
[36] | Hochbaum G (1958) Public Participation in Medical Screening Programs: A Socio-Psychological study. Washington, DC: US Department of Health, Education and Welfare. |
[37] | Fisher W, Fisher J, Harman J (2003) The information-motivation-behavioral skills model: A general social psychological approach to understanding and promoting health behavior. In: Suls J, Wallston K, Social Psychological Foundations of Health and Illness. Malden, MA: Blackwell, 82-116. |
[38] |
Ajzen I (1991) The theory of planned behavior. Organ Behav Human Decis Processes 50: 179-211. doi: 10.1016/0749-5978(91)90020-T
![]() |
[39] | Glanz K, Rimer BK, Viswanath K (2008) Health behavior and health education: Theory, research, and practice (4th ed.), Jossey-Bass. |
[40] |
Davis K (1963) The theory of change and response in modern demographic history. Popul Index 29: 345-366. doi: 10.2307/2732014
![]() |
[41] | Omran A (1971) The epidemiologic transition. A theory of the epidemiology of population change. Milbank Q 49: 509-538. |
[42] |
Young FW, Lyson TA (2001) Structural pluralism and all-cause mortality. Am J Public Health 91: 136-138. doi: 10.2105/AJPH.91.1.136
![]() |
[43] |
Anderson NB, Armstead CA (1995) Toward understanding the association of socioeconomic status and health: A new challenge fro bio-psychological approach. Psychomatic Med 57: 213-225. doi: 10.1097/00006842-199505000-00003
![]() |
[44] |
Duncan OD, Schnore LF, Rossi PH (1959) Cultural, behavioral and ecological perspectives in the study of social organization. Am J Social O 65: 132-153. doi: 10.1086/222654
![]() |
[45] | Young FW, Minai K (2002) Community ecology: A new theory and an illustrative test. Res Human Ecol 9: 31-40. |
[46] | R Core Team (2019) R 3.6.1: A language and environment for statistical computing. Vienna, Austria: R Foundation for Statistical Computing. Available from: https://www.R-project.org. |
[47] | Revelle W (2018) psych: Procedures for personality and psychological research. 1.8.12 ed. Evanston, IL: Northwestern University. |
[48] |
Cronbach LJ (1951) Coefficient alpha and the internal structure of tests. Pschometrika 16: 297-334. doi: 10.1007/BF02310555
![]() |
[49] | Nunnaly JC (1978) Psychometric Theory. New York: McGraw-Hill. |
[50] | Wei T, Simko V (2017) "corr plot": Visualization of a correlation matrix. 0.84 ed. |
[51] | Fox J, Weisberg S (2019) An {R} Companion to Applied Regression, Third Edition. Thousand Oaks, CA: Sage. |
[52] |
Flavio B, Daniela C, Pasquale S (2018) Genetically modified food versus knowledge and fear: A Noumenic approach for consumer behaviour. Food Res Int 111: 682-688. doi: 10.1016/j.foodres.2018.06.013
![]() |
[53] |
Parimi V, Kotamraju VKK, Sudini HK (2018) On-farm demonstrations with a set of good agricultural practices (GAPs) proved cost-effective in reducing pre-harvest aflatoxin contamination in groundnut. Agronomy 8: 10. doi: 10.3390/agronomy8020010
![]() |
1. | Gabriel Camero, Guillermo Villamizar, Luis M. Pombo, Manuel Saba, Arthur L. Frank, Aníbal A. Teherán, Gerhard M. Acero, Epidemiology of Asbestosis between 2010–2014 and 2015–2019 Periods in Colombia: Descriptive Study, 2023, 89, 2214-9996, 10.5334/aogh.3963 | |
2. | Benoit Talbot, Antoinette Ludwig, Sheila F. O’Brien, Steven J. Drews, Nicholas H. Ogden, Manisha A. Kulkarni, Spatial and temporal analysis of West Nile virus infection in mosquito and human populations based on arboviral detection testing data, 2024, 14, 2045-2322, 10.1038/s41598-024-82739-3 |
Males |
Females |
|||||||
2011 | 2012 | 2013 | 2014 | 2011 | 2012 | 2013 | 2014 | |
0–19 | 0.03% | −2.51% | 0.04% | 1.43% | 0.01% | −2.24% | −0.09% | 1.03% |
20–39 | −1.22% | −5.22% | −1.32% | 0.45% | −1.10% | −4.33% | −1.50% | −0.14% |
40–59 | 1.22% | −1.36% | 1.69% | 3.40% | 1.49% | −0.53% | 1.57% | 3.13% |
60–79 | 1.04% | −0.66% | 1.19% | 2.25% | 0.52% | −0.91% | 0.72% | 1.60% |
80–100 | 4.74% | 1.96% | 3.58% | 4.03% | 3.47% | 0.77% | 2.31% | 2.77% |
All age groups | 0.43% | −2.33% | 0.57% | 2.06% | 0.52% | −1.75% | 0.42% | 1.63% |
2012 vs. 2011 | 2013 vs. 2012 | 2014 vs. 2013 | |
Abruzzo | 1.0275d | 0.9954 | 0.9839 |
Aosta Valley | 1.0127 | 0.9904 | 0.9942 |
Apulia | 1.0102 | 0.9998 | 0.9904 |
Basilicata | 1.0172 | 1.0024 | 0.9962 |
Calabria | 1.0271d | 1.0001 | 0.9887 |
Campania | 1.0121d | 0.9991 | 0.9829d |
Emilia Romagna | 1.021d | 0.9917 | 0.9845d |
Friuli-Venezia Giulia | 1.0148 | 0.9967 | 0.9939 |
Lazio | 1.0416c,d | 0.9897 | 0.9467c,d |
Liguria | 1.0315d | 1.0014 | 0.9832 |
Lombardy | 1.0224d | 0.9904d | 0.9821d |
Marche | 1.016 | 0.9971 | 0.9949 |
Molise | 1.0212 | 0.9994 | 0.9956 |
Piedmont | 1.0229d | 0.9963 | 0.9859d |
Sardinia | 1.0229d | 0.9985 | 0.9859 |
Sicily | 1.0102 | 0.9999 | 0.9814d |
Tuscany | 1.0224d | 0.9932 | 0.9846d |
Trentino-Alto Adige | 1.0073 | 0.9900 | 0.9886 |
Umbria | 1.0263 | 0.9966 | 0.9883 |
Veneto | 1.0173d | 0.9942 | 0.9909 |
Italy | 1.0207c,d | 0.9951d | 0.9819c,d |
Note: Statistically significant difference with “index year” fixed at incidence rate: (a) 1/100,000; (b) 10/100,000; (c) 100/100,000 and (d) 1,000/100,000.
Males |
Females |
|||||||
2011 | 2012 | 2013 | 2014 | 2011 | 2012 | 2013 | 2014 | |
0–19 | 0.03% | −2.51% | 0.04% | 1.43% | 0.01% | −2.24% | −0.09% | 1.03% |
20–39 | −1.22% | −5.22% | −1.32% | 0.45% | −1.10% | −4.33% | −1.50% | −0.14% |
40–59 | 1.22% | −1.36% | 1.69% | 3.40% | 1.49% | −0.53% | 1.57% | 3.13% |
60–79 | 1.04% | −0.66% | 1.19% | 2.25% | 0.52% | −0.91% | 0.72% | 1.60% |
80–100 | 4.74% | 1.96% | 3.58% | 4.03% | 3.47% | 0.77% | 2.31% | 2.77% |
All age groups | 0.43% | −2.33% | 0.57% | 2.06% | 0.52% | −1.75% | 0.42% | 1.63% |
2012 vs. 2011 | 2013 vs. 2012 | 2014 vs. 2013 | |
Abruzzo | 1.0275d | 0.9954 | 0.9839 |
Aosta Valley | 1.0127 | 0.9904 | 0.9942 |
Apulia | 1.0102 | 0.9998 | 0.9904 |
Basilicata | 1.0172 | 1.0024 | 0.9962 |
Calabria | 1.0271d | 1.0001 | 0.9887 |
Campania | 1.0121d | 0.9991 | 0.9829d |
Emilia Romagna | 1.021d | 0.9917 | 0.9845d |
Friuli-Venezia Giulia | 1.0148 | 0.9967 | 0.9939 |
Lazio | 1.0416c,d | 0.9897 | 0.9467c,d |
Liguria | 1.0315d | 1.0014 | 0.9832 |
Lombardy | 1.0224d | 0.9904d | 0.9821d |
Marche | 1.016 | 0.9971 | 0.9949 |
Molise | 1.0212 | 0.9994 | 0.9956 |
Piedmont | 1.0229d | 0.9963 | 0.9859d |
Sardinia | 1.0229d | 0.9985 | 0.9859 |
Sicily | 1.0102 | 0.9999 | 0.9814d |
Tuscany | 1.0224d | 0.9932 | 0.9846d |
Trentino-Alto Adige | 1.0073 | 0.9900 | 0.9886 |
Umbria | 1.0263 | 0.9966 | 0.9883 |
Veneto | 1.0173d | 0.9942 | 0.9909 |
Italy | 1.0207c,d | 0.9951d | 0.9819c,d |